Application of convolutional neural networks based on Bayesian optimization to landslide susceptibility mapping of transmission tower foundation
BULLETIN OF ENGINEERING GEOLOGY AND THE ENVIRONMENT(2023)
摘要
The stability of tower foundation slopes is an important factor to maintain the operation of a power system. However, it is time-consuming and expensive to evaluate tower foundation slopes one by one due to the large area. The aim of this study is to investigate the performance of CNNs with different architectures and training options for transmission tower foundation landslide spatial prediction (LSP) by Bayesian optimization. Accordingly, fourteen influencing factors related to landslide evaluated by gain ratio technique are considered and 424 historical landslide locations in Luoding and Xinyi Counties (Guangdong Province, China) are randomly divided into 80% for training and 20% for testing the CNNs. The CNN performances are investigated by permutating and combining different numbers of convolutional layers, pooling layers and learning rate strategy. In 59 Bayesian optimized cases, three conclusions are drawn: (a) the CNNs yielded the best result with 3 convolution layers, (b) the CNN without a pooling layer performs best, and (c) a piece-wise decay learning rate strategy yields better performance. Meanwhile, the excellent performance of the CNN obtained by Bayesian optimization (CNN B ) has also been validated by comparisons with gravitational search optimization algorithm and other landslide spatial models, which indicates that CNN B can be applied to generate the susceptibility maps for locating transmission tower foundations in high landslide susceptibility zones and reducing the impact of landslides on power supply by taking measures in advance.
更多查看译文
关键词
Convolutional neural networks,Bayesian optimization,Landslide susceptibility map,Geographic information system,Transmission tower foundation
AI 理解论文
溯源树
样例
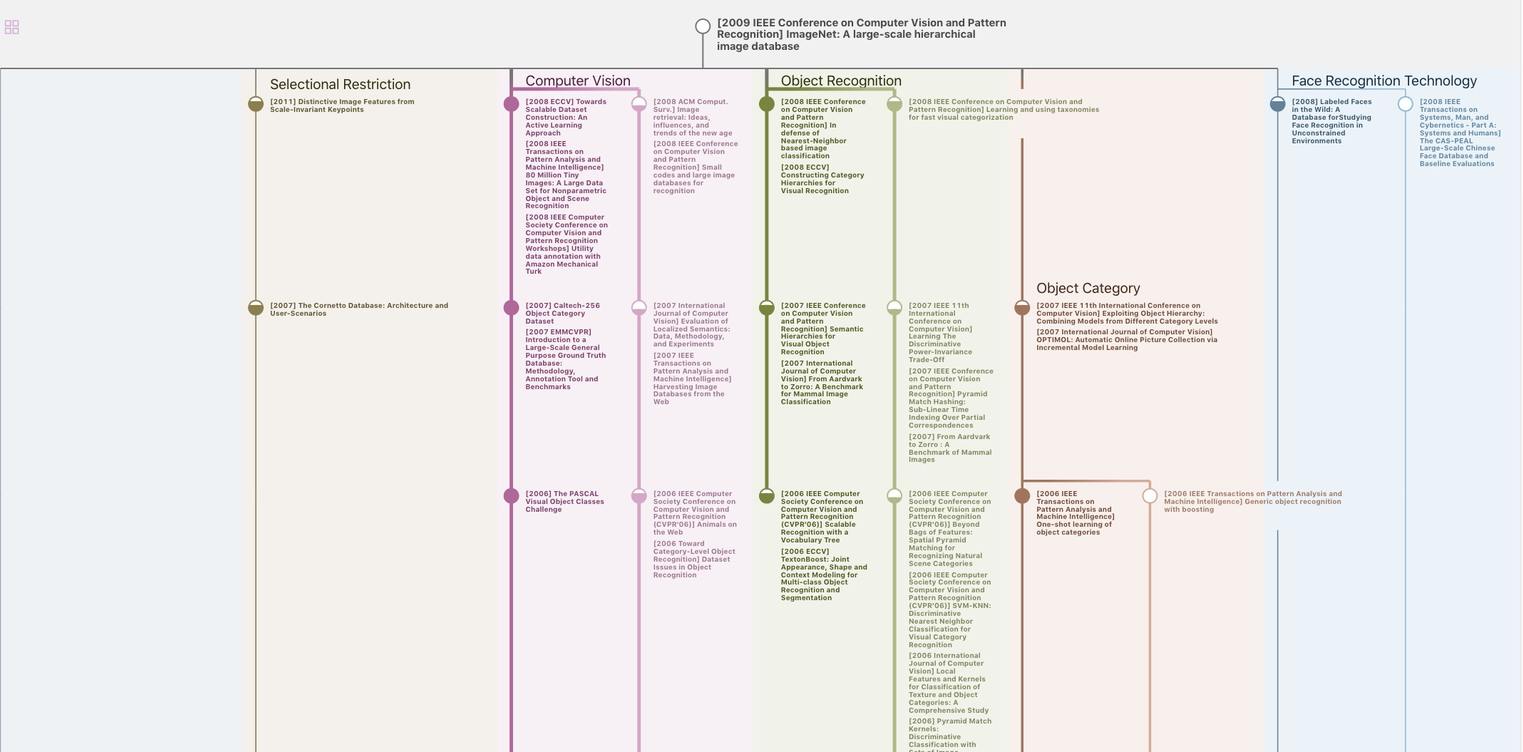
生成溯源树,研究论文发展脉络
Chat Paper
正在生成论文摘要