A Methodology of Retrieving Volume Emission Rate from Limb-Viewed Airglow Emission Intensity by Combining the Techniques of Abel Inversion and Deep Learning
ATMOSPHERE(2023)
摘要
The conversion of airglow intensity to volume emission rate (VER) is a common method for studying the ionosphere, but the contribution of the intensity conversion process to the uncertainty in estimated electron or ion density is significant. The Abel inversion is a commonly used method for retrieving VERs from vertical profiles of airglow intensities accumulated along the rays horizontally at the tangent point, but it requires that the intensities converge to zero at their uppermost height, which is often not the case due to observational limitations. In this study, we present a method for optimizing the retrieval of VER from satellite-measured airglow intensities using the techniques of deep learning and Abel inversion. This method can be applied to fill in unobserved or discontinuous observations in airglow intensity profiles with the Chapman function, allowing them to be used with the Abel inversion to determine VERs. We validate the method using limb 135.6 nm airglow emission intensity data from the NASA Global-scale Observations of the Limb and Disk (GOLD) mission. Our training process involves using three hidden layers with varying numbers of neurons, and we compare the performance of the best-performing deep learning models to Abel-transformed results from real-time observations. The combination of Abel inversion and deep learning has the potential to optimize the process of converting intensity to VER and improve the capacity for analyzing ionospheric observations.
更多查看译文
关键词
airglow,GOLD,Chapman distribution,Abel inversion,deep learning
AI 理解论文
溯源树
样例
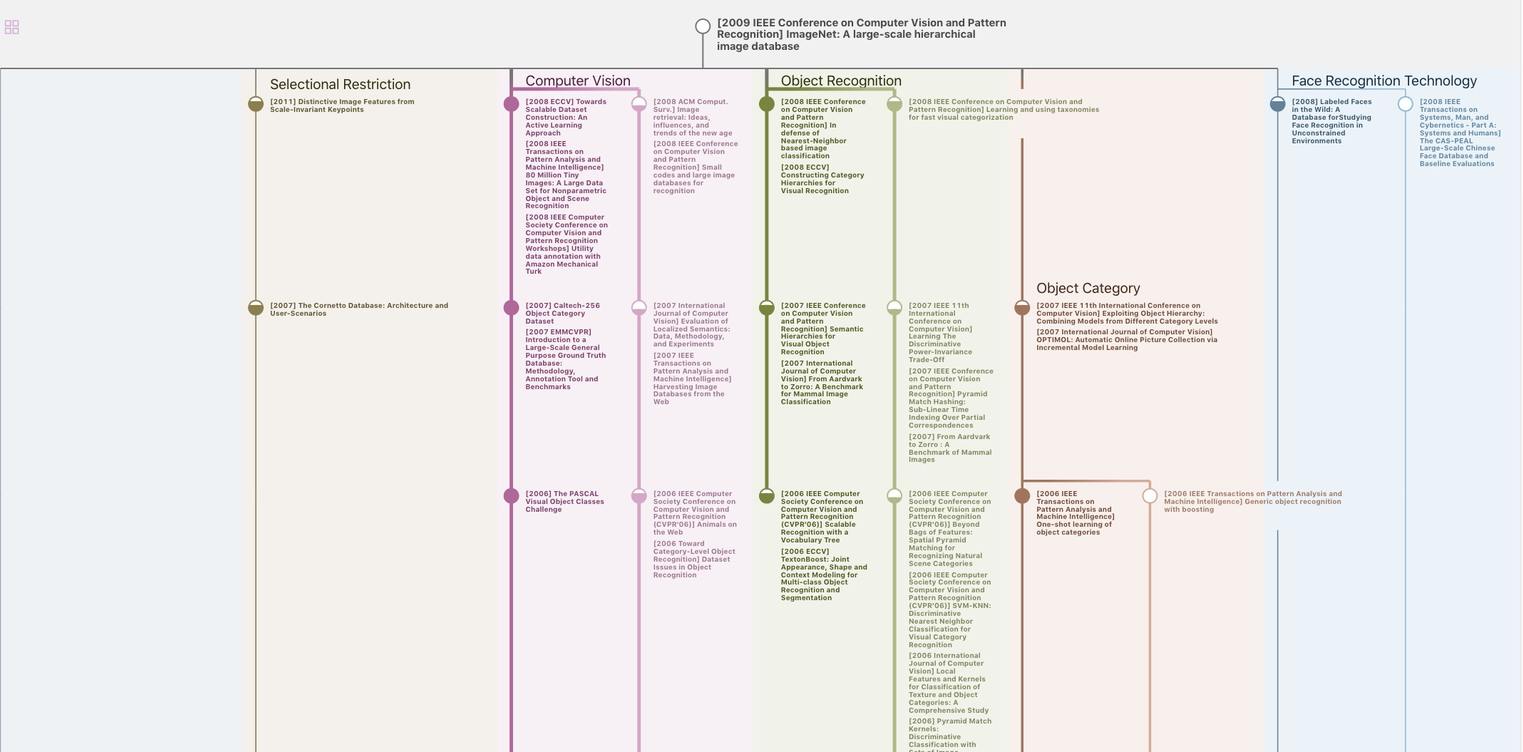
生成溯源树,研究论文发展脉络
Chat Paper
正在生成论文摘要