Feature-Limited Prediction on the UCI Heart Disease Dataset
CMC-COMPUTERS MATERIALS & CONTINUA(2023)
摘要
Heart diseases are the undisputed leading causes of death globally. Unfortunately, the conventional approach of relying solely on the patient's medical history is not enough to reliably diagnose heart issues. Several potentially indicative factors exist, such as abnormal pulse rate, high blood pressure, diabetes, high cholesterol, etc. Manually analyzing these health signals' interactions is challenging and requires years of medical training and experience. Therefore, this work aims to harness machine learning techniques that have proved helpful for data-driven applications in the rise of the artificial intelligence era. More specifically, this paper builds a hybrid model as a tool for data mining algorithms like feature selection. The goal is to determine the most critical factors that play a role in discriminating patients with heart illnesses from healthy individuals. The contribution in this field is to provide the patients with accurate and timely tentative results to help prevent further complications and heart attacks using minimum information. The developed model achieves 84.24% accuracy, 89.22% Recall, and 83.49% Precision using only a subset of the features.
更多查看译文
关键词
Machine learning,feature selection,heart disease
AI 理解论文
溯源树
样例
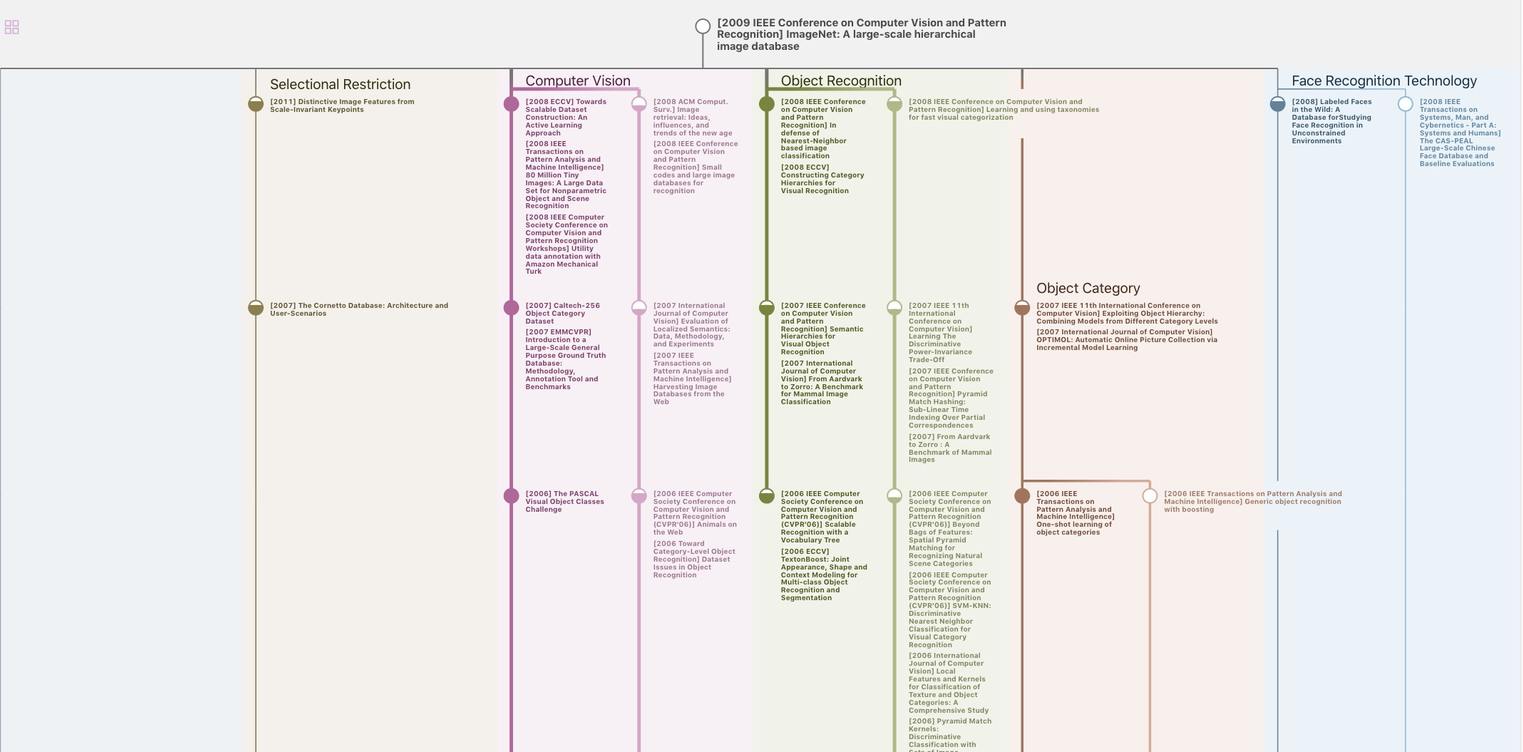
生成溯源树,研究论文发展脉络
Chat Paper
正在生成论文摘要