Optimization of a Regional Marine Environment Mobile Observation Network Based on Deep Reinforcement Learning
JOURNAL OF MARINE SCIENCE AND ENGINEERING(2023)
摘要
The observation path planning of an ocean mobile observation network is an important part of the ocean mobile observation system. With the aim of developing a traditional algorithm to solve the observation path of the mobile observation network, a complex objective function needs to be constructed, and an improved deep reinforcement learning algorithm is proposed. The improved deep reinforcement learning algorithm does not need to establish the objective function. The agent samples the marine environment information by exploring and receiving feedback from the environment. Focusing on the real-time dynamic variability of the marine environment, our experiment shows that adding bidirectional recurrency to the Deep Q-network allows the Q-network to better estimate the underlying system state. Compared with the results of existing algorithms, the improved deep reinforcement learning algorithm can effectively improve the sampling efficiency of the observation platform. To improve the prediction accuracy of the marine environment numerical prediction system, we conduct sampling path experiments on a single platform, double platform, and five platforms. The experimental results show that increasing the number of observation platforms can effectively improve the prediction accuracy of the numerical prediction system, but when the number of observation platforms exceeds 2, increasing the number of observation platforms will not improve the prediction accuracy, and there is a certain degree of decline. In addition, in the multi-platform experiment, the improved deep reinforcement learning algorithm is compared with the unimproved algorithm, and the results show that the proposed algorithm is better than the existing algorithm.
更多查看译文
关键词
deep reinforcement learning,marine environment observation,USV,path optimization,LSTM
AI 理解论文
溯源树
样例
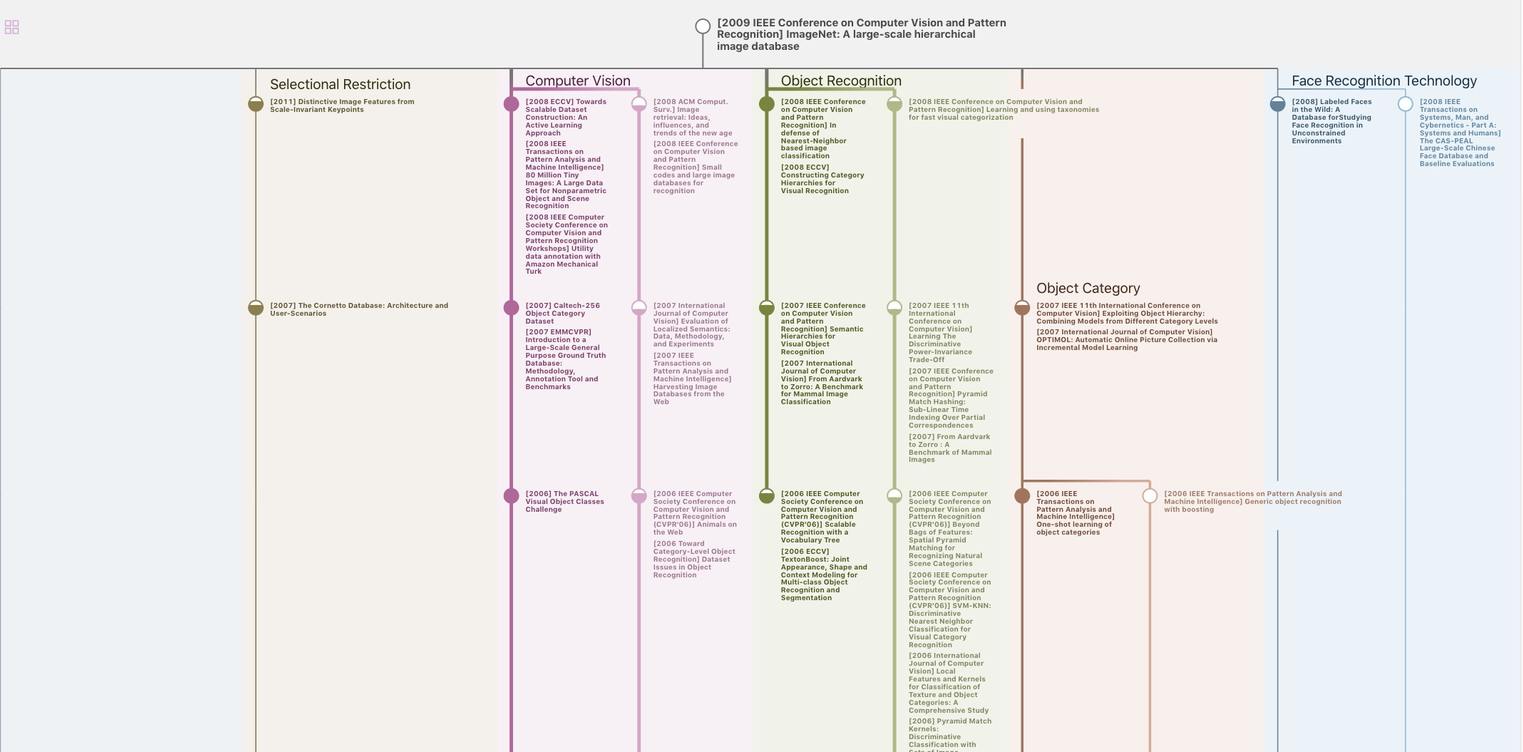
生成溯源树,研究论文发展脉络
Chat Paper
正在生成论文摘要