Neonatal seizure detection using deep belief networks from multichannel EEG data
NEURAL COMPUTING & APPLICATIONS(2023)
摘要
Seizures in neonates happen to be one of the most difficult emergency circumstances to deal with. They are the first signs of significant neurological implications and should be precisely diagnosed for prompt medical intervention to avoid serious morbidities and occasional fatalities. In most cases, unlike adults, newborns show practically no physical indications of an epileptic fit. Hitherto, seizure diagnosis has relied solely on consistent laborious manual monitoring and interpretation of long-duration recordings of electroencephalograms (EEG). However, because the brains of the newborns remain nascent during the neonatal period, interpreting the EEG data necessitates the expertise of highly skilled experts. This study demonstrates a Deep Belief Network-based Machine Learning architecture for binary classification of seizure and seizure-free periods. The classifier was trained and its performance was assessed using a publicly available annotated dataset containing EEG recordings of 79 infants collected at Helsinki University Hospital. The proposed model was highly discriminative for seizure detection (AUC: 97.1%). Our architecture establishes a high accuracy of 98.7 percentage, when applied to multichannel EEG recordings. The mean sensitivity and specificity rates were observed to be 96.1% and 99.2%, respectively, deeming the model comparable to recent advancements in machine learning classifiers on our dataset.
更多查看译文
关键词
Neonatal seizures,EEG,Signal processing,Triplet half-band filter,Deep belief networks,Restricted Boltzmann machine
AI 理解论文
溯源树
样例
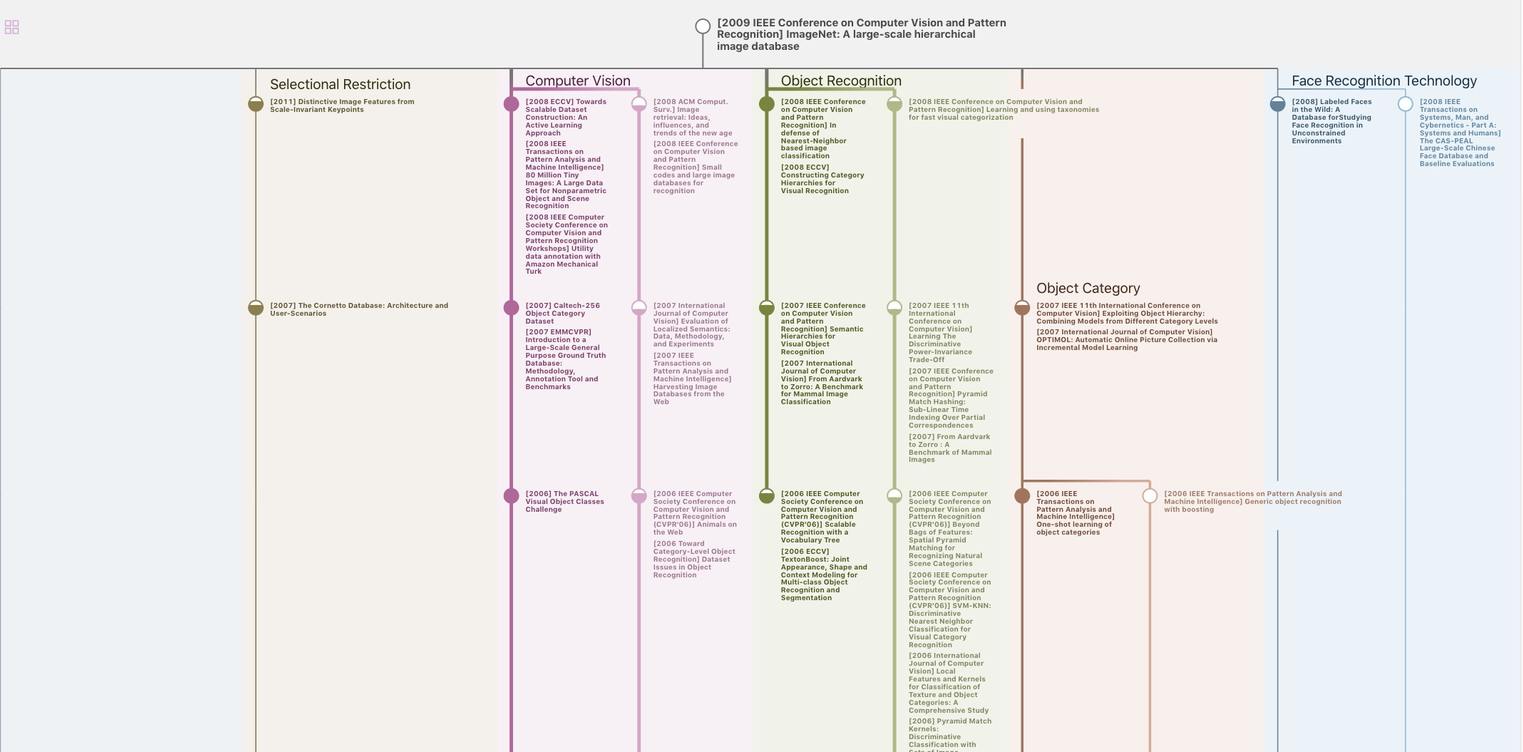
生成溯源树,研究论文发展脉络
Chat Paper
正在生成论文摘要