Deep transformer and few-shot learning for hyperspectral image classification
CAAI TRANSACTIONS ON INTELLIGENCE TECHNOLOGY(2023)
摘要
Recently, deep learning has achieved considerable results in the hyperspectral image (HSI) classification. However, most available deep networks require ample and authentic samples to better train the models, which is expensive and inefficient in practical tasks. Existing few-shot learning (FSL) methods generally ignore the potential relationships between non-local spatial samples that would better represent the underlying features of HSI. To solve the above issues, a novel deep transformer and few-shot learning (DT-FSL) classification framework is proposed, attempting to realize fine-grained classification of HSI with only a few-shot instances. Specifically, the spatial attention and spectral query modules are introduced to overcome the constraint of the convolution kernel and consider the information between long-distance location (non-local) samples to reduce the uncertainty of classes. Next, the network is trained with episodes and task-based learning strategies to learn a metric space, which can continuously enhance its modelling capability. Furthermore, the developed approach combines the advantages of domain adaptation to reduce the variation in inter-domain distribution and realize distribution alignment. On three publicly available HSI data, extensive experiments have indicated that the proposed DT-FSL yields better results concerning state-of-the-art algorithms.
更多查看译文
关键词
deep learning,feature extraction,hyperspectral,image classification
AI 理解论文
溯源树
样例
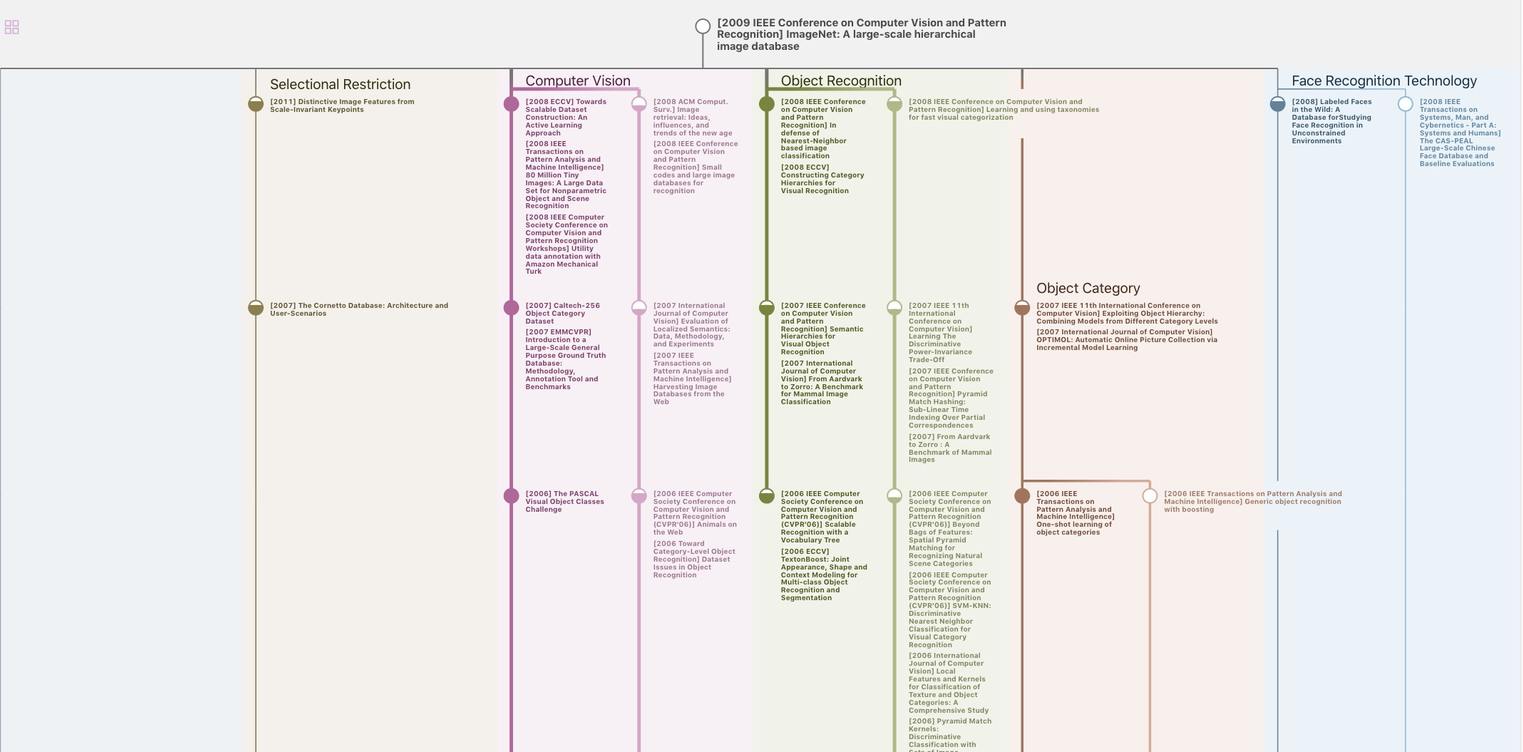
生成溯源树,研究论文发展脉络
Chat Paper
正在生成论文摘要