SafeCool: Safe and Energy-Efficient Cooling Management in Data Centers With Model-Based Reinforcement Learning
IEEE TRANSACTIONS ON EMERGING TOPICS IN COMPUTATIONAL INTELLIGENCE(2023)
摘要
Optimizing the cooling system plays a central role for capping the data center power consumption. However, the performance of traditional cooling management strategies is not satisfactory due to the complexity of thermodynamic process. Recently, several works leveraged Reinforcement Learning (RL) to improve the energy efficiency of data center cooling system. While they demonstrated that it is possible to reduce the cooling power consumption via RL, there are still some key challenges that have to be addressed before field deployment, such as safe operation guarantee and sample complexity, etc. In this paper, we propose SafeCool, an actor-critic Model-Based Reinforcement Learning (MBRL) algorithm for data center cooling management. SafeCool incorporates two system models, i.e., a transition model to predict the future system state and a risk model to estimate the negative effect of executing an action. The safety of proposed algorithm is ensured by Model Predictive Control (MPC) and risk-guided exploration. In addition, by employing the MBRL framework, SafeCool achieves higher sample efficiency and accelerated convergence. Simulations using real-world workload trace reveal that SafeCool saves up to 13.18% cooling power compared with state-of-the-art MBRL data center cooling solutions.
更多查看译文
关键词
Cooling,Data centers,Atmospheric modeling,Data models,Servers,Power demand,Temperature distribution,Data center cooling management,safe operation and exploration,model-based reinforcement learning
AI 理解论文
溯源树
样例
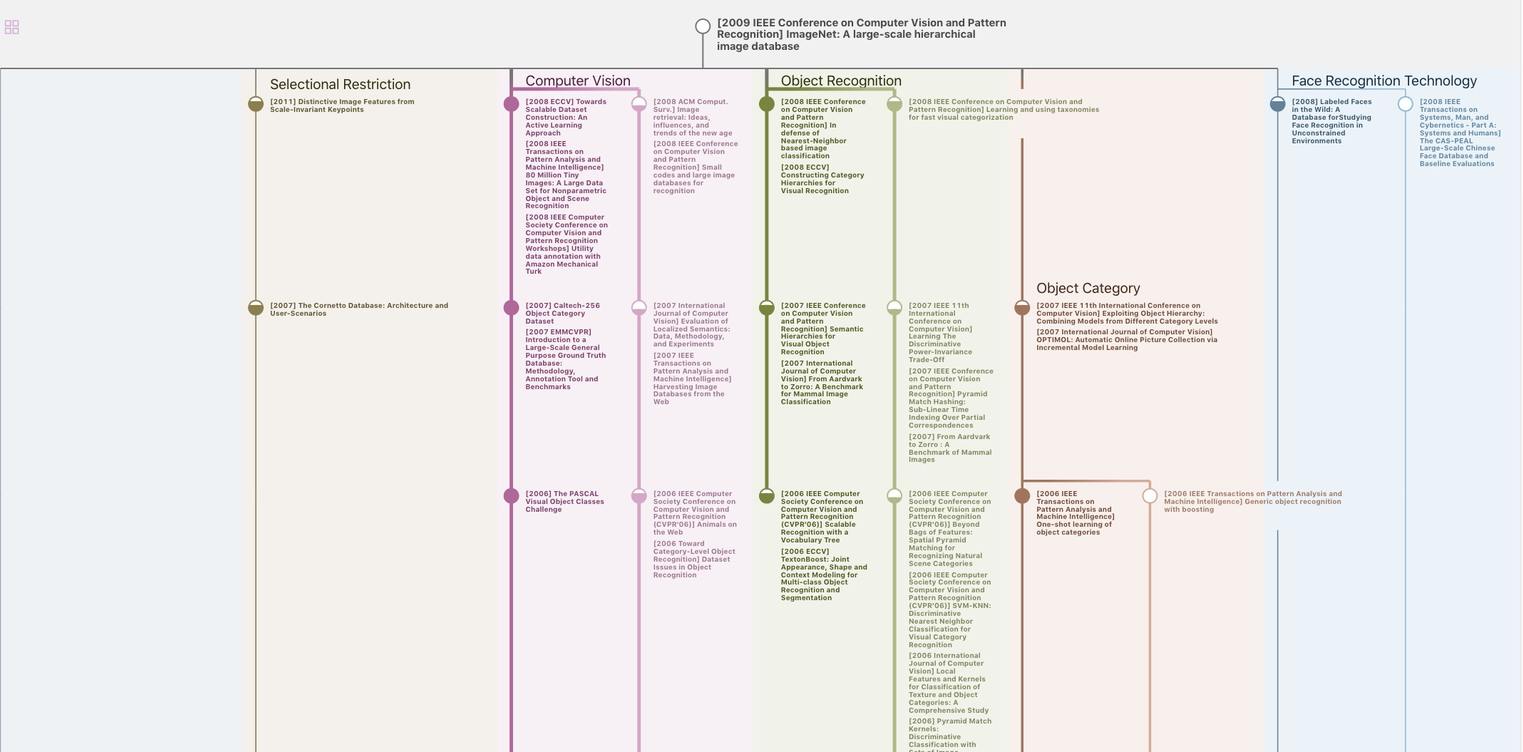
生成溯源树,研究论文发展脉络
Chat Paper
正在生成论文摘要