Dfree-LSTM: An error distribution free deep learning for short-term traffic flow forecasting
NEUROCOMPUTING(2023)
摘要
Timely and accurate traffic flow forecasting is open challenging. Canonical long short-term memory (LSTM) network is considered qualified to capture the long-term temporal dependencies in traffic flow. However, the training of LSTM networks is often guided by the mean square error (MSE) criterion. Such criterion depends on a strong assumption that the errors between the traffic flow and its predictions are Gaussian independent identically distributed. In this regard, the forecasting performance is seriously deteriorated by non-Gaussian noises inside the traffic flow sequences. To address this issue, we relax the assumption of the prediction errors to arbitrary distribution by a negative guided mixed correntropy cri-terion. Then, we formulate a robust loss function by the negative guided mixed correntropy criterion. We subsequently equip the loss function in an LSTM network, termed Dfree-LSTM, for short-term traffic flow forecasting. Extensive experiments on four benchmark datasets demonstrate that the Dfree-LSTM network outperforms the traditional parametric and nonparametric models, as well as state-of-the-art LSTM fam-ily models. The source code is available athttps://github.com/541764418/Delta-free-LSTM.(c) 2023 Elsevier B.V. All rights reserved.
更多查看译文
关键词
Intelligent transportation system,Traffic flow modeling,Time series analysis,Deep learning,Noise -immune learning
AI 理解论文
溯源树
样例
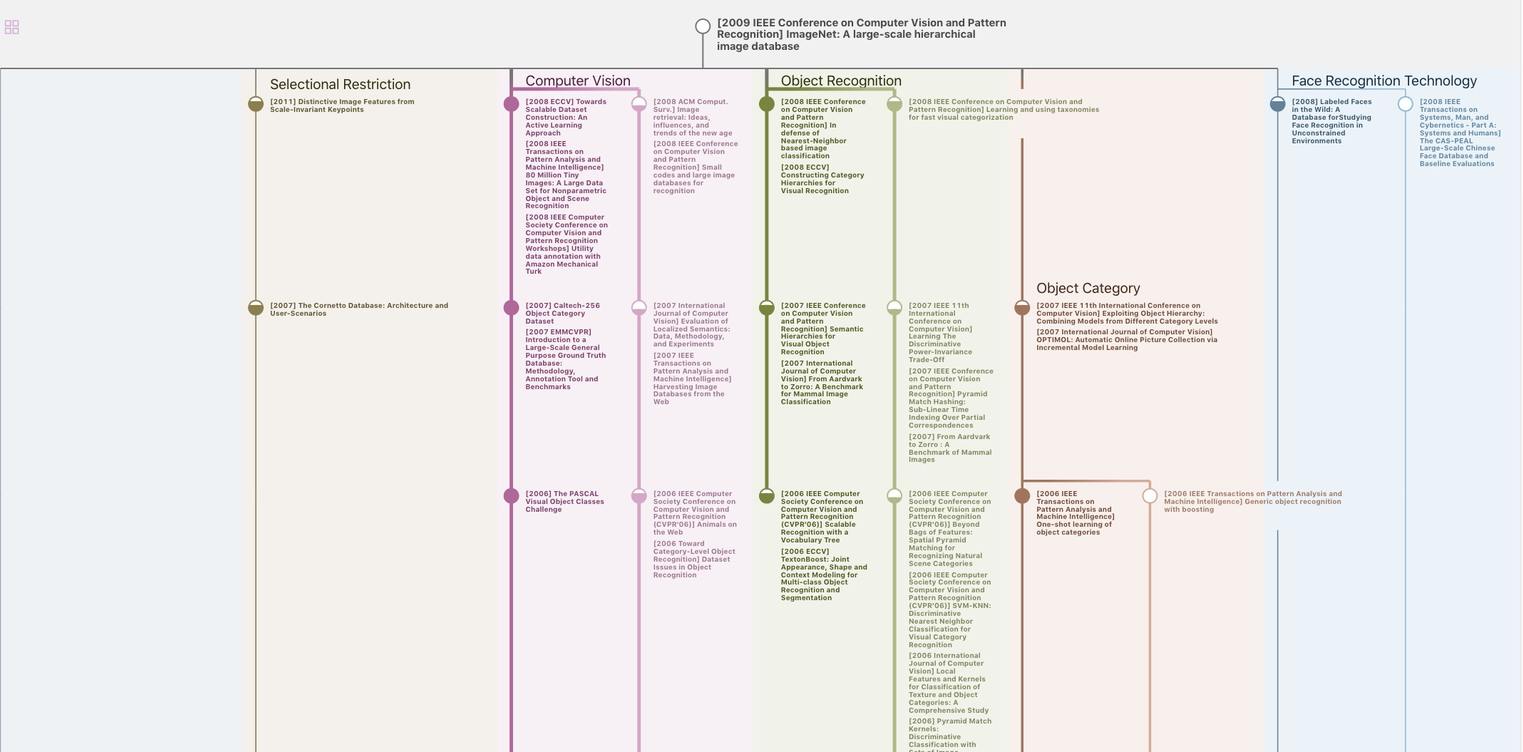
生成溯源树,研究论文发展脉络
Chat Paper
正在生成论文摘要