An Integrated machine learning and DEA-predefined performance outcome prediction framework with high-dimensional imbalanced data
INFOR(2024)
摘要
In performance evaluation, emerging studies utilize machine learning to increase the interpretability and robustness of data envelopment analysis (DEA), a non-parametric tool for assessing the relative performance of decision-making units (DMUs). In these studies, the machine learning dynamics typically do not replicate the DEA process in terms of directly labeling DMUs based on their relative performance. Practically, there is no standardized methodological framework that serves this purpose. We propose a data-driven and computationally efficient system that imitates DEA and predicts performance outcomes, which are grouped into several classes. First, a DEA composite index was constructed, and the subsequent DEA scores were labeled as the good, the acceptable, and the underperforming classes. Next, synthetic minority oversampling technique (SMOTE) with Manhattan distance metric was used to solve class imbalance in the labeled, high-dimensional dataset. The framework was built using different classifiers, including random forest, support vector machine, and logistic regression, to verify that the framework is not model-dependent. They achieved comparable recall rates (82.70%-95.39%). Moreover, the impacts of contextual variables on DMU performance were unveiled using model-based feature selection and logistic regression. The framework was tested on a banking dataset and an independent dataset containing the electronics, service, and retail industries.
更多查看译文
关键词
Data envelopment analysis,machine learning,feature selection,performance evaluation,contextual variables
AI 理解论文
溯源树
样例
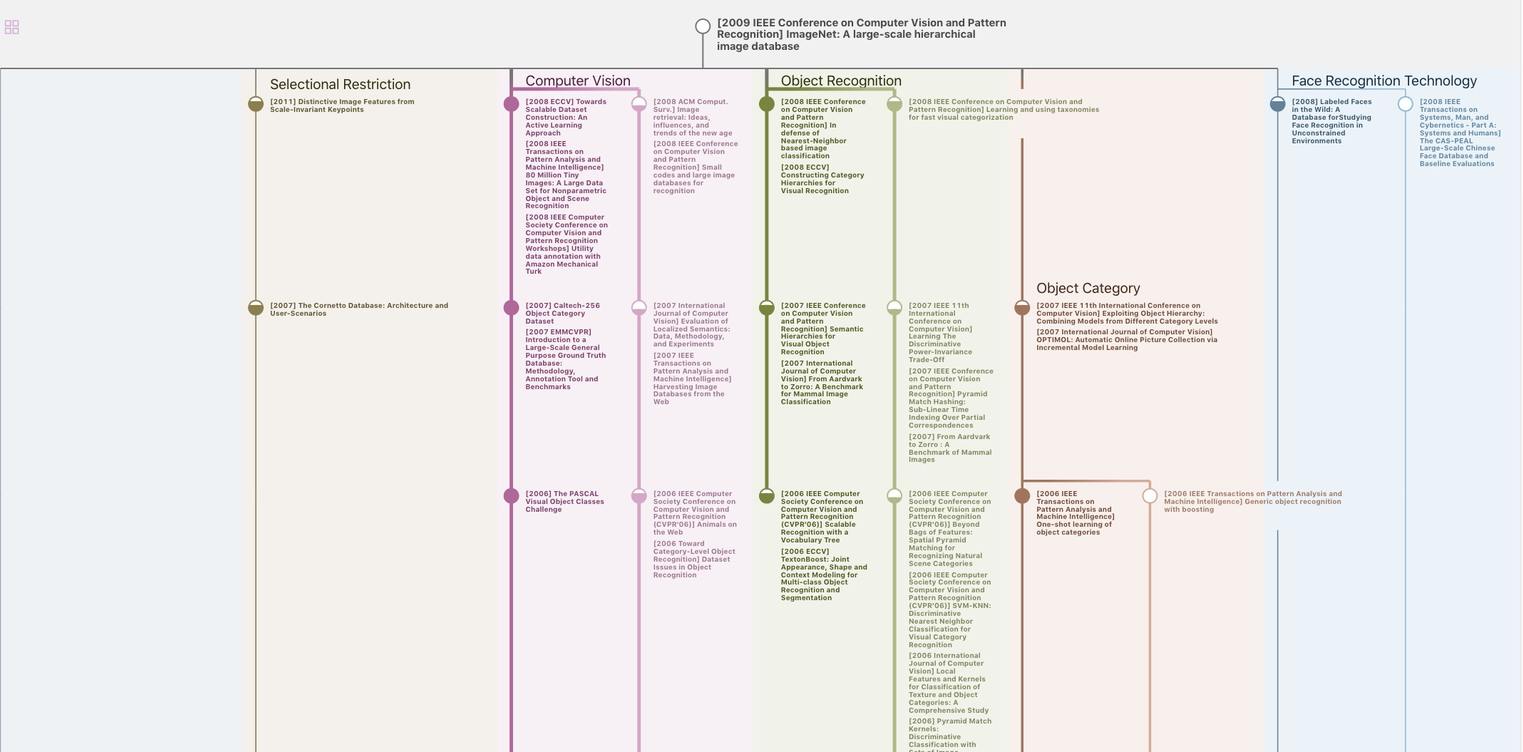
生成溯源树,研究论文发展脉络
Chat Paper
正在生成论文摘要