FESSD:SSD target detection based on feature fusion and feature enhancement
JOURNAL OF REAL-TIME IMAGE PROCESSING(2023)
摘要
In recent years, significant breakthroughs have been made in target detection. However, although the existing two-stage target detection algorithm has high precision, the detection velocity is slow to content the real-time requirements. One-stage target detection algorithms can meet real-time requirements but have poor detection capabilities, especially for detecting the small target. In this paper, we propose an end-to-end feature fusion and feature enhancement SSD (FESSD) target detection algorithm to increase the capability of one-stage target detection. Firstly, a deeper ResNet-50 is used to replace VGG16 as the backbone network to obtain richer semantic information. Five extra layers are added to generate feature maps of different sizes for multi-scale target detection. Then, the feature maps are fused by the maximum pooling feature fusion module (MPFFM) and upsampling feature fusion module (UPFFM) to generate a new feature pyramid, which introduces semantic information into the shallow feature mapping. Finally, the feature enhancement module (FEM) is used to expand the receptive field of the output feature map, introduce more context information, and further enhance the feature expression ability of the model. Experimental results on the PASCAL VOC and MS COCO datasets validated the method’s validity.
更多查看译文
关键词
Target detection, Feature fusion, Feature enhancement, Receptive field
AI 理解论文
溯源树
样例
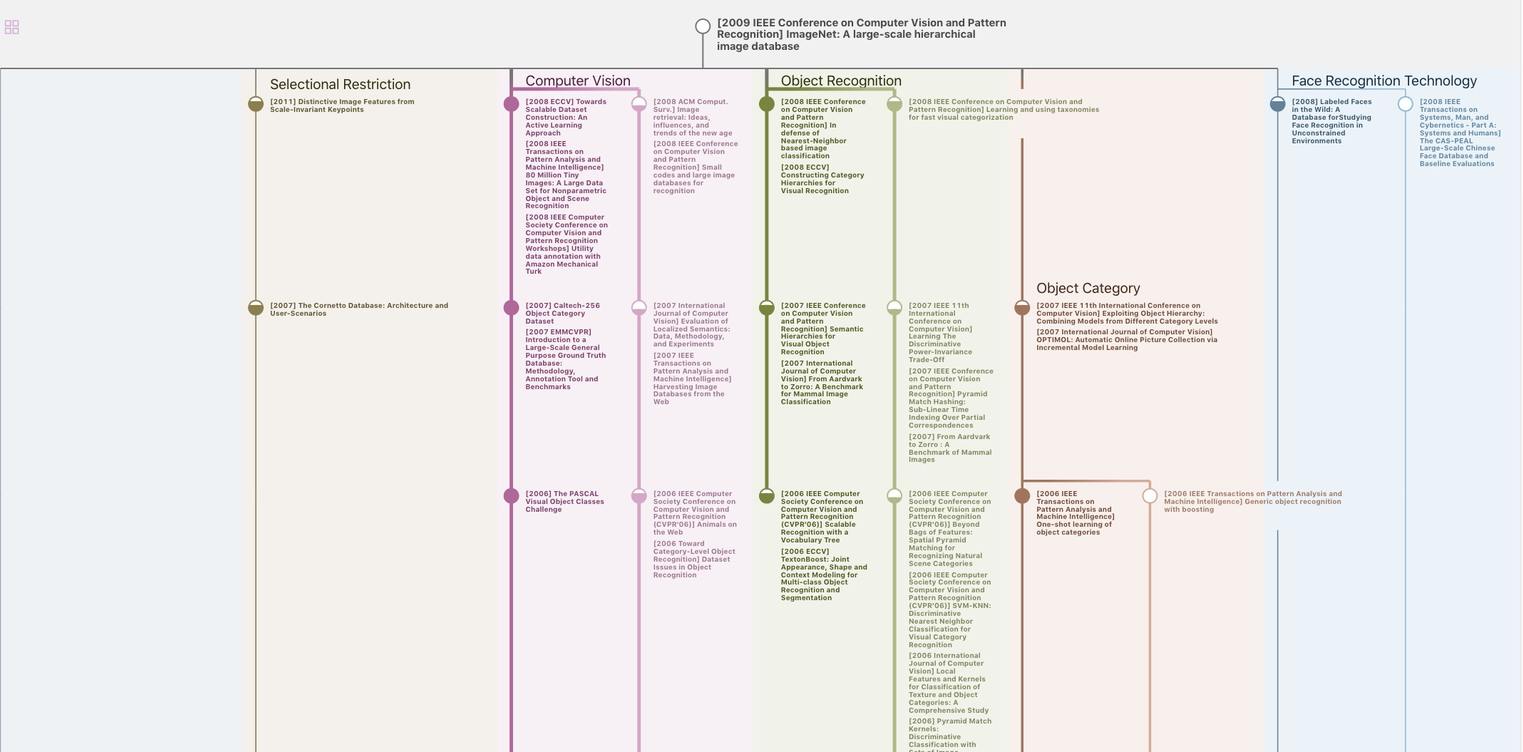
生成溯源树,研究论文发展脉络
Chat Paper
正在生成论文摘要