Lazy Lagrangians for Optimistic Learning With Budget Constraints
IEEE-ACM TRANSACTIONS ON NETWORKING(2023)
摘要
We consider the general problem of online convex optimization with time-varying budget constraints in the presence of predictions for the next cost and constraint functions, that arises in a plethora of network resource management problems. A novel saddle-point algorithm is designed by combining a Follow-The-Regularized-Leader iteration with prediction-adaptive dynamic steps. The algorithm achieves O(T(3-beta)/4) regret and O(T(1+beta)/2) constraint violation bounds that are tunable via parameter beta is an element of [1/2, 1) and have constant factors that shrink with the predictions quality, achieving eventually O(1) regret for perfect predictions. Our work extends the seminal FTRL framework for this new OCO setting and outperforms the respective state-of-the-art greedy based solutions which naturally cannot benefit from predictions, without imposing conditions on the (unknown) quality of predictions, the cost functions or the geometry of constraints, beyond convexity.
更多查看译文
关键词
Prediction algorithms,Costs,Benchmark testing,Heuristic algorithms,Indexes,IEEE transactions,Convex functions,Network control,network management,resource allocation,online convex optimization (OCO),online learning
AI 理解论文
溯源树
样例
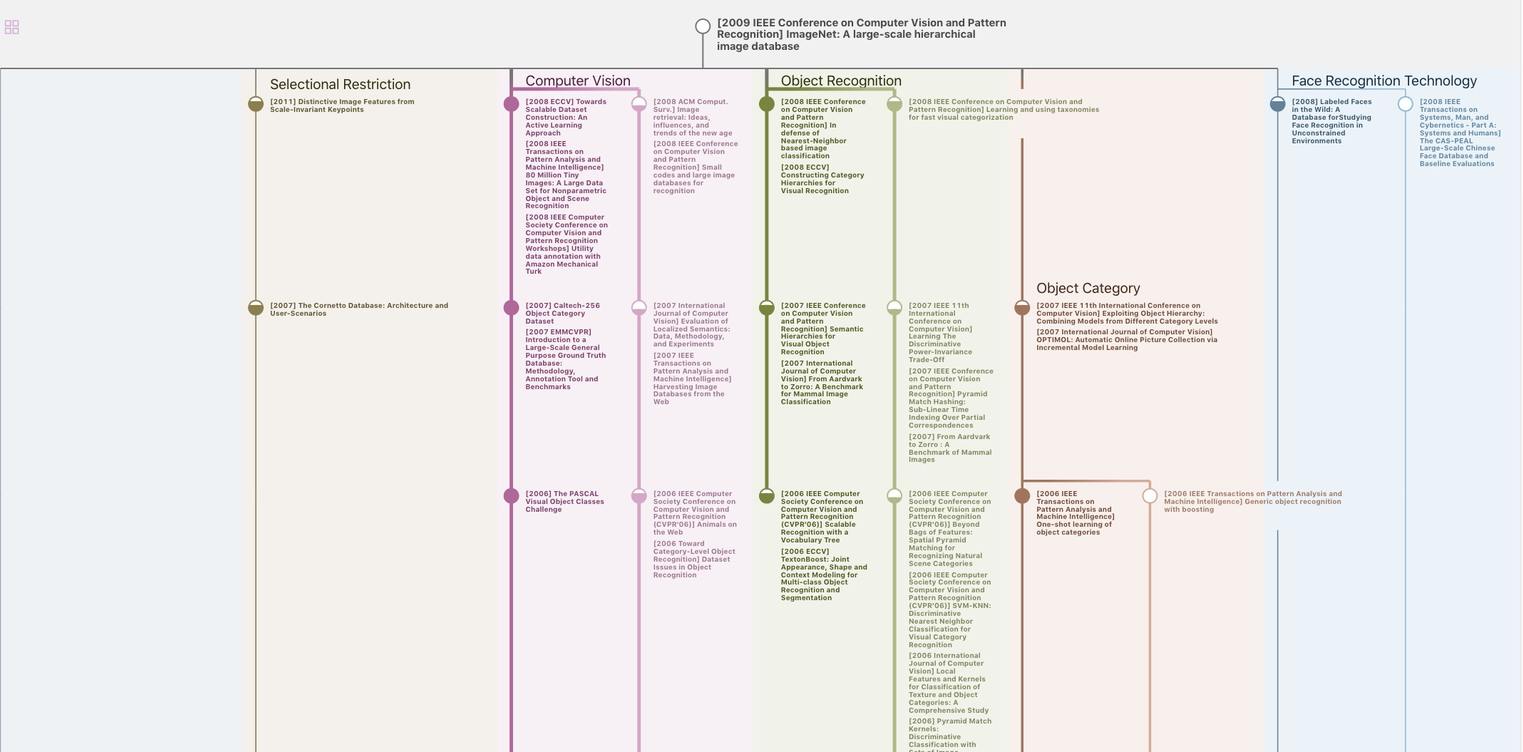
生成溯源树,研究论文发展脉络
Chat Paper
正在生成论文摘要