Feature Consistency-Based Prototype Network for Open-Set Hyperspectral Image Classification.
IEEE transactions on neural networks and learning systems(2023)
摘要
Hyperspectral image (HSI) classification methods have made great progress in recent years. However, most of these methods are rooted in the closed-set assumption that the class distribution in the training and testing stages is consistent, which cannot handle the unknown class in open-world scenes. In this work, we propose a feature consistency-based prototype network (FCPN) for open-set HSI classification, which is composed of three steps. First, a three-layer convolutional network is designed to extract the discriminative features, where a contrastive clustering module is introduced to enhance the discrimination. Then, the extracted features are used to construct a scalable prototype set. Finally, a prototype-guided open-set module (POSM) is proposed to identify the known samples and unknown samples. Extensive experiments reveal that our method achieves remarkable classification performance over other state-of-the-art classification techniques.
更多查看译文
关键词
Feature extraction,Prototypes,Training,Testing,Hyperspectral imaging,Convolutional neural networks,Task analysis,Contrastive clustering,feature consistency,hyperspectral image (HSI),open-set classification,prototype network
AI 理解论文
溯源树
样例
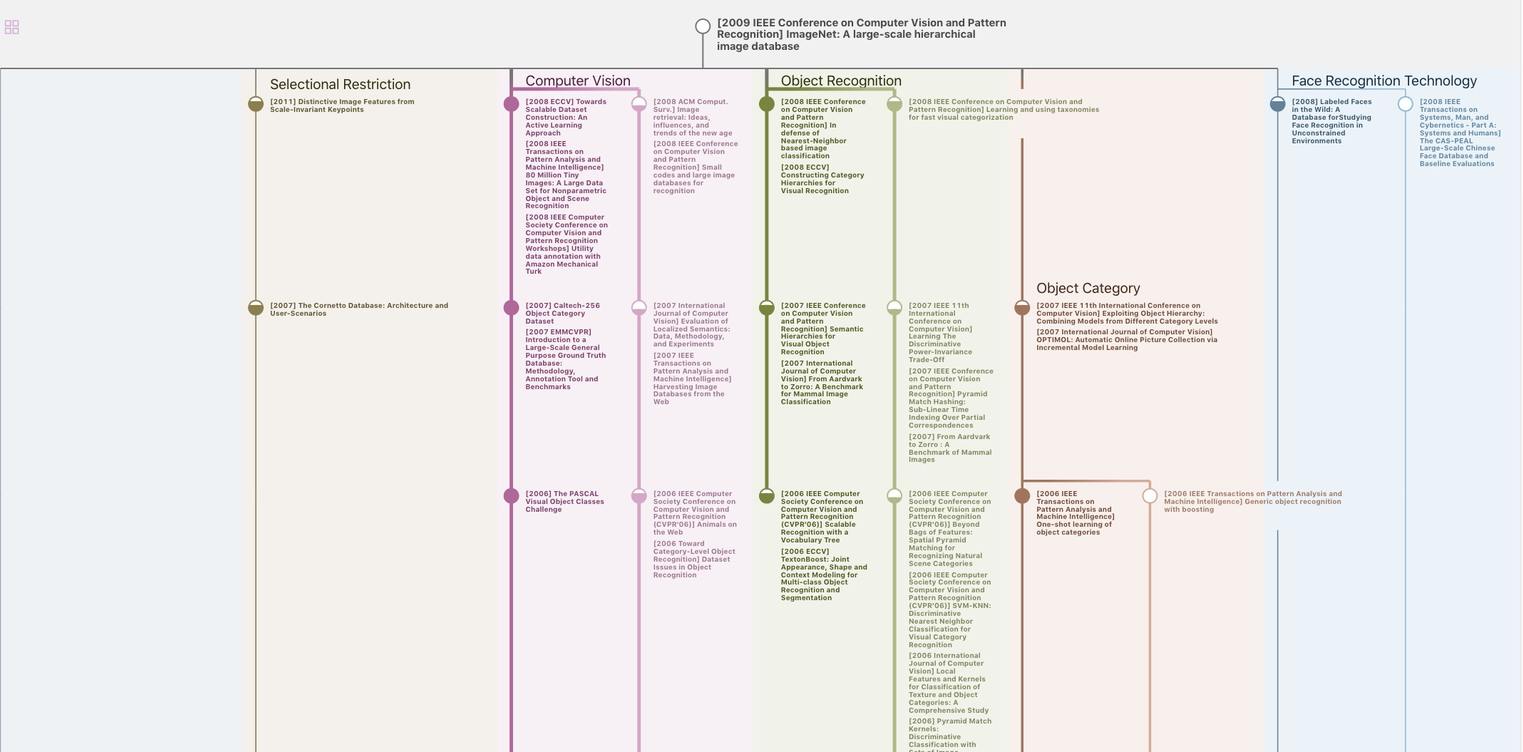
生成溯源树,研究论文发展脉络
Chat Paper
正在生成论文摘要