Compensating for Sensing Failures via Delegation in Human-AI Hybrid Systems
Sensors (Basel, Switzerland)(2023)
摘要
Given an increasing prevalence of intelligent systems capable of autonomous actions or augmenting human activities, it is important to consider scenarios in which the human, autonomous system, or both can exhibit failures as a result of one of several contributing factors (e.g. perception). Failures for either humans or autonomous agents can lead to simply a reduced performance level, or a failure can lead to something as severe as injury or death. For our topic, we consider the hybrid human-AI teaming case where a managing agent is tasked with identifying when to perform a delegation assignment and whether the human or autonomous system should gain control. In this context, the manager will estimate its best action based on the likelihood of either (human, autonomous) agent failure as a result of their sensing capabilities and possible deficiencies. We model how the environmental context can contribute to, or exacerbate, the sensing deficiencies. These contexts provide cases where the manager must learn to attribute capabilities to suitability for decision-making. As such, we demonstrate how a Reinforcement Learning (RL) manager can correct the context-delegation association and assist the hybrid team of agents in outperforming the behavior of any agent working in isolation.
更多查看译文
关键词
simulated sensing,entity detection,reinforcement learning,delegation
AI 理解论文
溯源树
样例
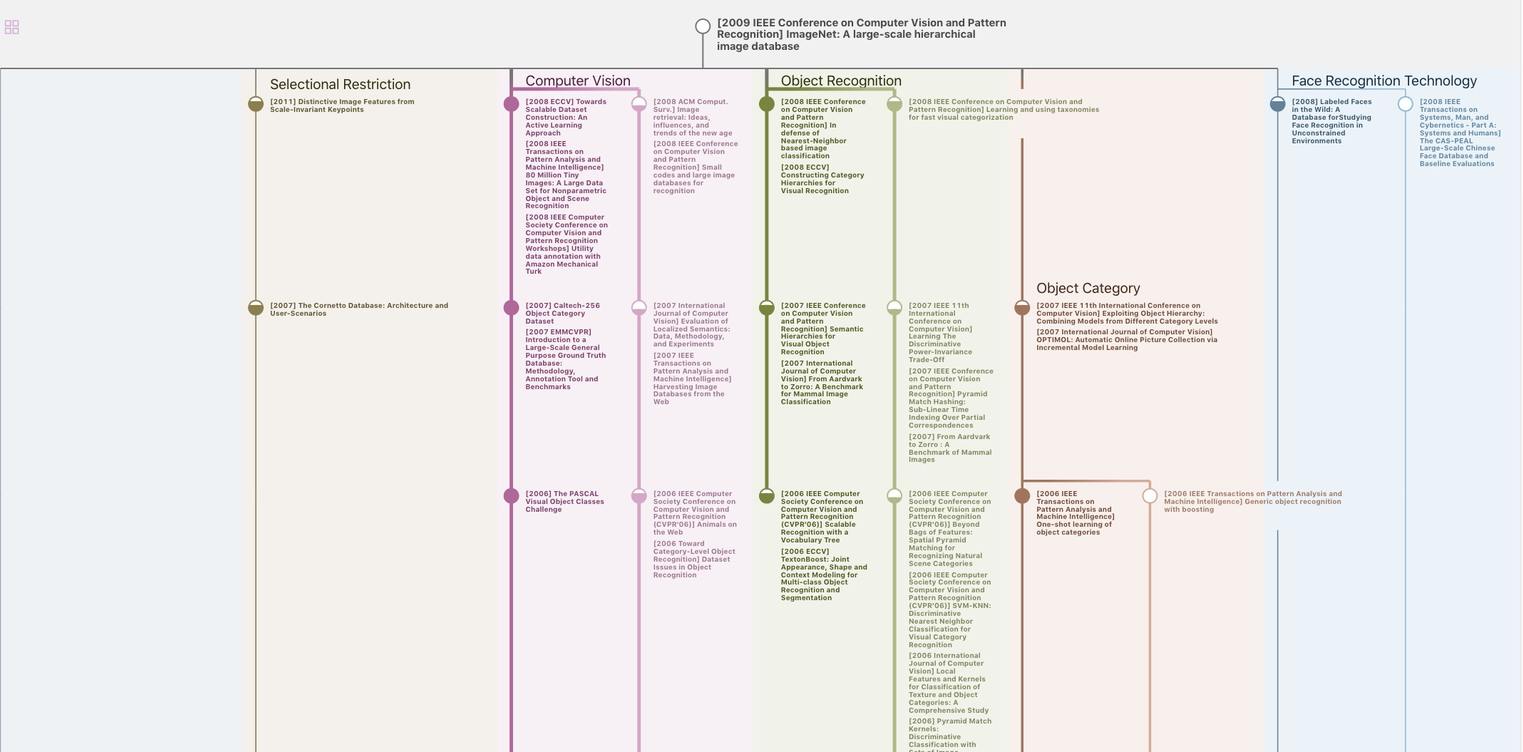
生成溯源树,研究论文发展脉络
Chat Paper
正在生成论文摘要