NeU-NBV: Next Best View Planning Using Uncertainty Estimation in Image-Based Neural Rendering
2023 IEEE/RSJ INTERNATIONAL CONFERENCE ON INTELLIGENT ROBOTS AND SYSTEMS (IROS)(2023)
摘要
Autonomous robotic tasks require actively perceiving the environment to achieve application-specific goals. In this paper, we address the problem of positioning an RGB camera to collect the most informative images to represent an unknown scene, given a limited measurement budget. We propose a novel mapless planning framework to iteratively plan the next best camera view based on collected image measurements. A key aspect of our approach is a new technique for uncertainty estimation in image-based neural rendering, which guides measurement acquisition at the most uncertain view among view candidates, thus maximising the information value during data collection. By incrementally adding new measurements into our image collection, our approach efficiently explores an unknown scene in a mapless manner. We show that our uncertainty estimation is generalisable and valuable for view planning in unknown scenes. Our planning experiments using synthetic and real-world data verify that our uncertainty-guided approach finds informative images leading to more accurate scene representations when compared against baselines.
更多查看译文
关键词
best view planning,neural rendering,uncertainty estimation,neu-nbv,image-based
AI 理解论文
溯源树
样例
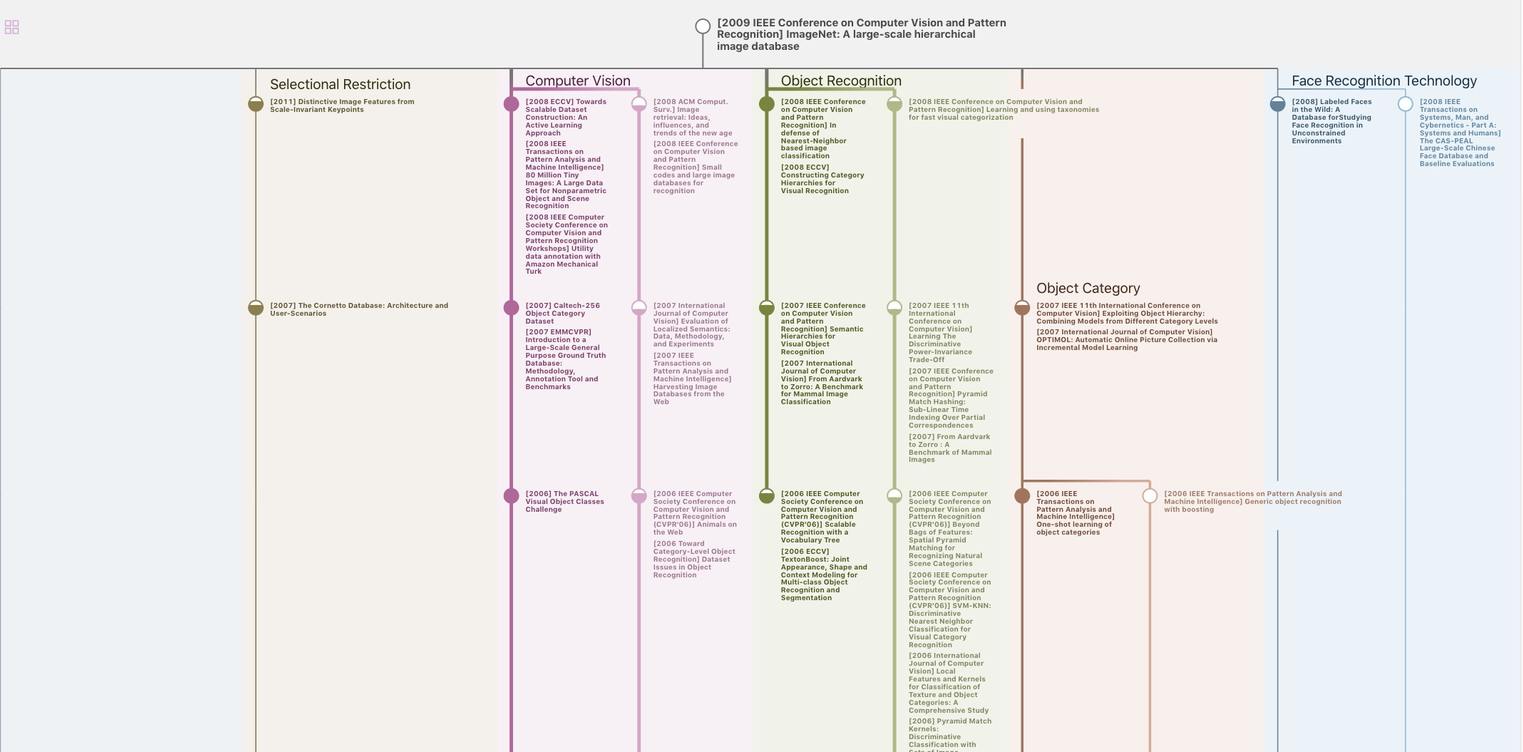
生成溯源树,研究论文发展脉络
Chat Paper
正在生成论文摘要