BIG Hype: Best Intervention in Games via Distributed Hypergradient Descent
arxiv(2023)
摘要
Hierarchical decision making problems, such as bilevel programs and
Stackelberg games, are attracting increasing interest in both the engineering
and machine learning communities. Yet, existing solution methods lack either
convergence guarantees or computational efficiency, due to the absence of
smoothness and convexity. In this work, we bridge this gap by designing a
first-order hypergradient-based algorithm for Stackelberg games and
mathematically establishing its convergence using tools from nonsmooth
analysis. To evaluate the hypergradient, namely, the gradient of the
upper-level objective, we develop an online scheme that simultaneously computes
the lower-level equilibrium and its Jacobian. Crucially, this scheme exploits
and preserves the original hierarchical and distributed structure of the
problem, which renders it scalable and privacy-preserving. We numerically
verify the computational efficiency and scalability of our algorithm on a
large-scale hierarchical demand-response model.
更多查看译文
AI 理解论文
溯源树
样例
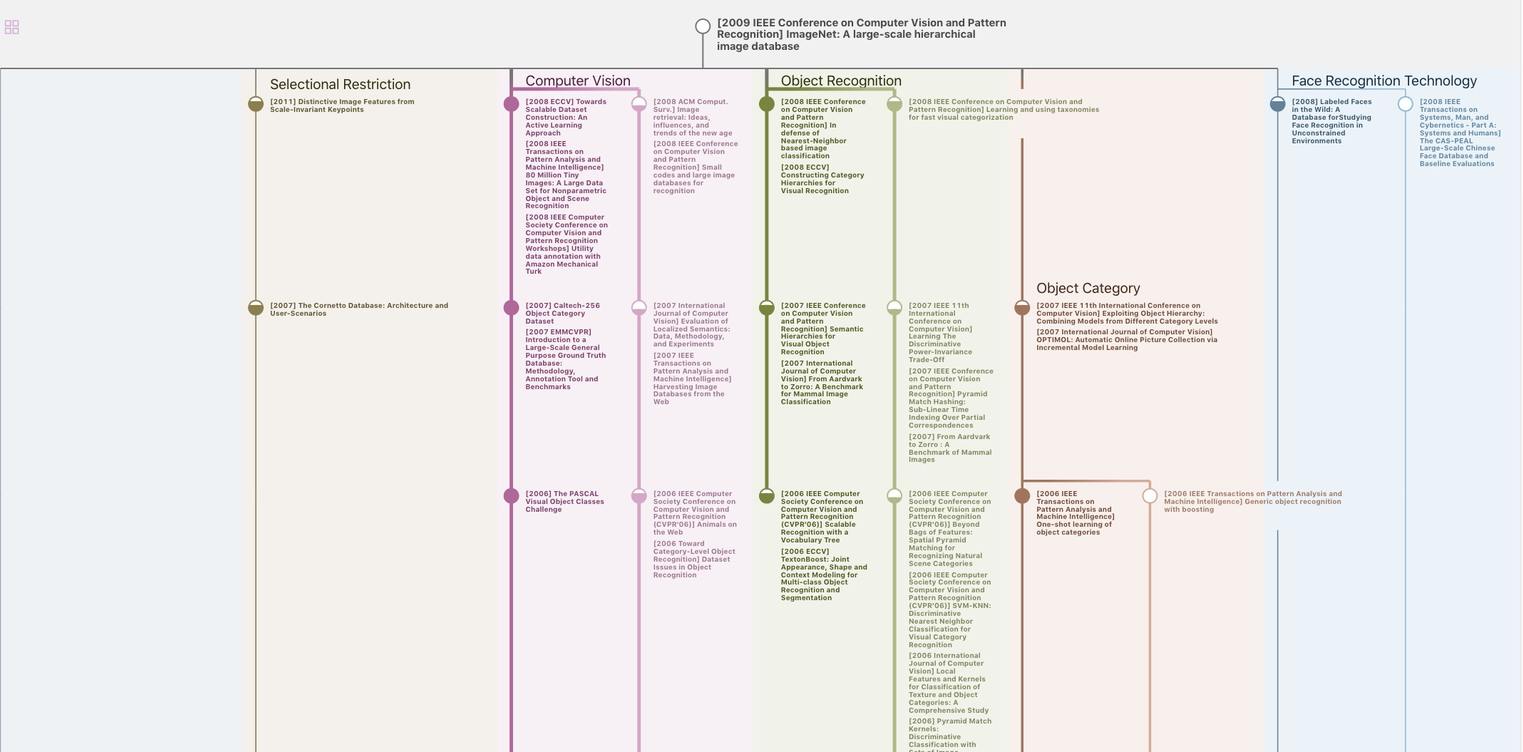
生成溯源树,研究论文发展脉络
Chat Paper
正在生成论文摘要