Multi-Source Soft Pseudo-Label Learning with Domain Similarity-based Weighting for Semantic Segmentation
2023 IEEE/RSJ INTERNATIONAL CONFERENCE ON INTELLIGENT ROBOTS AND SYSTEMS (IROS)(2023)
摘要
This paper describes a method of domain adaptive training for semantic segmentation using multiple source datasets that are not necessarily relevant to the target dataset. We propose a soft pseudo-label generation method by integrating predicted object probabilities from multiple source models. The prediction of each source model is weighted based on the estimated domain similarity between the source and the target datasets to emphasize contribution of a model trained on a source that is more similar to the target and generate reasonable pseudo-labels. We also propose a training method using the soft pseudo-labels considering their entropy to fully exploit information from the source datasets while suppressing the influence of possibly misclassified pixels. The experiments show comparative or better performance than our previous work and another existing multi-source domain adaptation method, and applicability to a variety of target environments.
更多查看译文
关键词
semantic segmentation,multi-source,pseudo-label,similarity-based
AI 理解论文
溯源树
样例
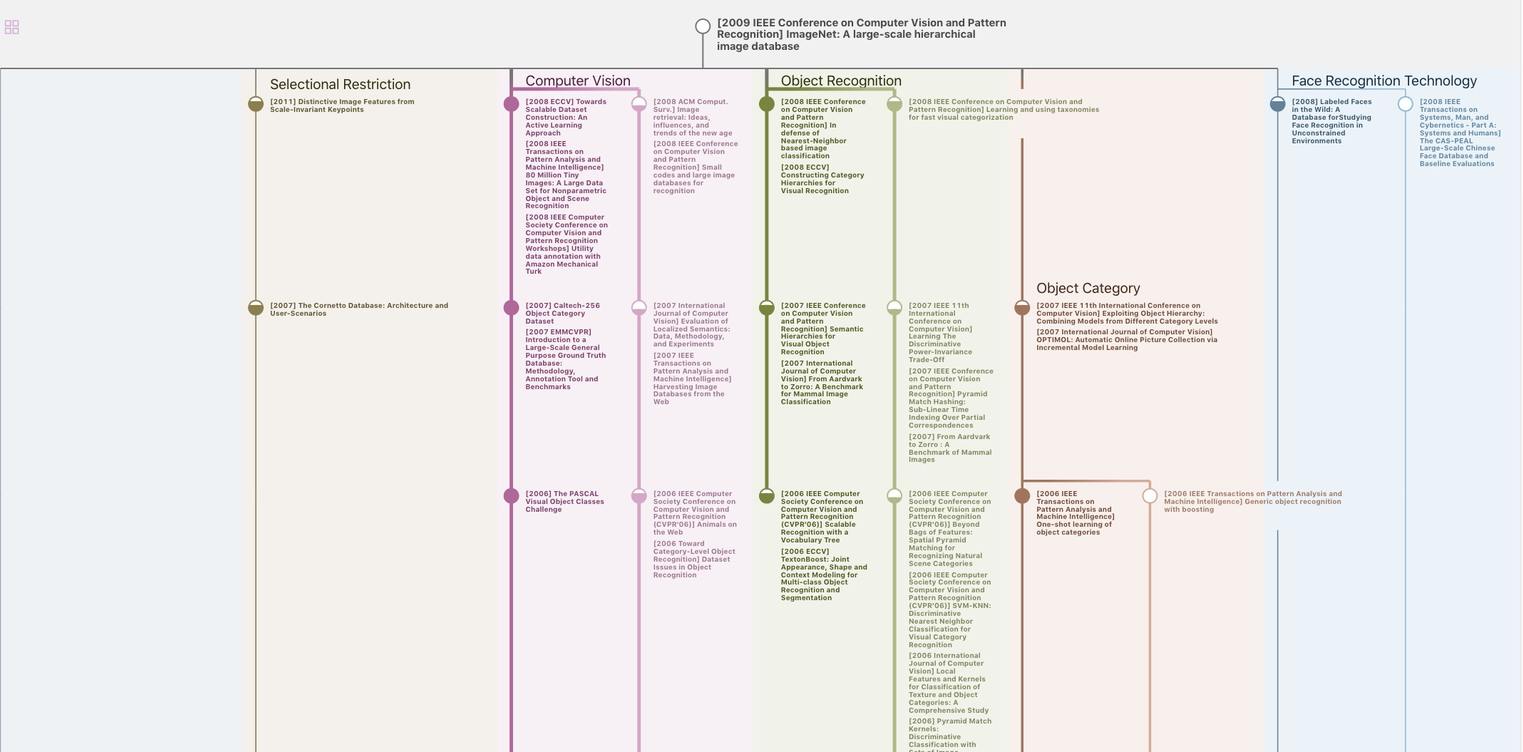
生成溯源树,研究论文发展脉络
Chat Paper
正在生成论文摘要