Rethinking the Reasonability of the Test Set for Simultaneous Machine Translation
arxiv(2023)
摘要
Simultaneous machine translation (SimulMT) models start translation before the end of the source sentence, making the translation monotonically aligned with the source sentence. However, the general full-sentence translation test set is acquired by offline translation of the entire source sentence, which is not designed for SimulMT evaluation, making us rethink whether this will underestimate the performance of SimulMT models. In this paper, we manually annotate a monotonic test set based on the MuST-C English-Chinese test set, denoted as SiMuST-C. Our human evaluation confirms the acceptability of our annotated test set. Evaluations on three different SimulMT models verify that the underestimation problem can be alleviated on our test set. Further experiments show that finetuning on an automatically extracted monotonic training set improves SimulMT models by up to 3 BLEU points.
更多查看译文
关键词
test set,translation,reasonability,simultaneous machine
AI 理解论文
溯源树
样例
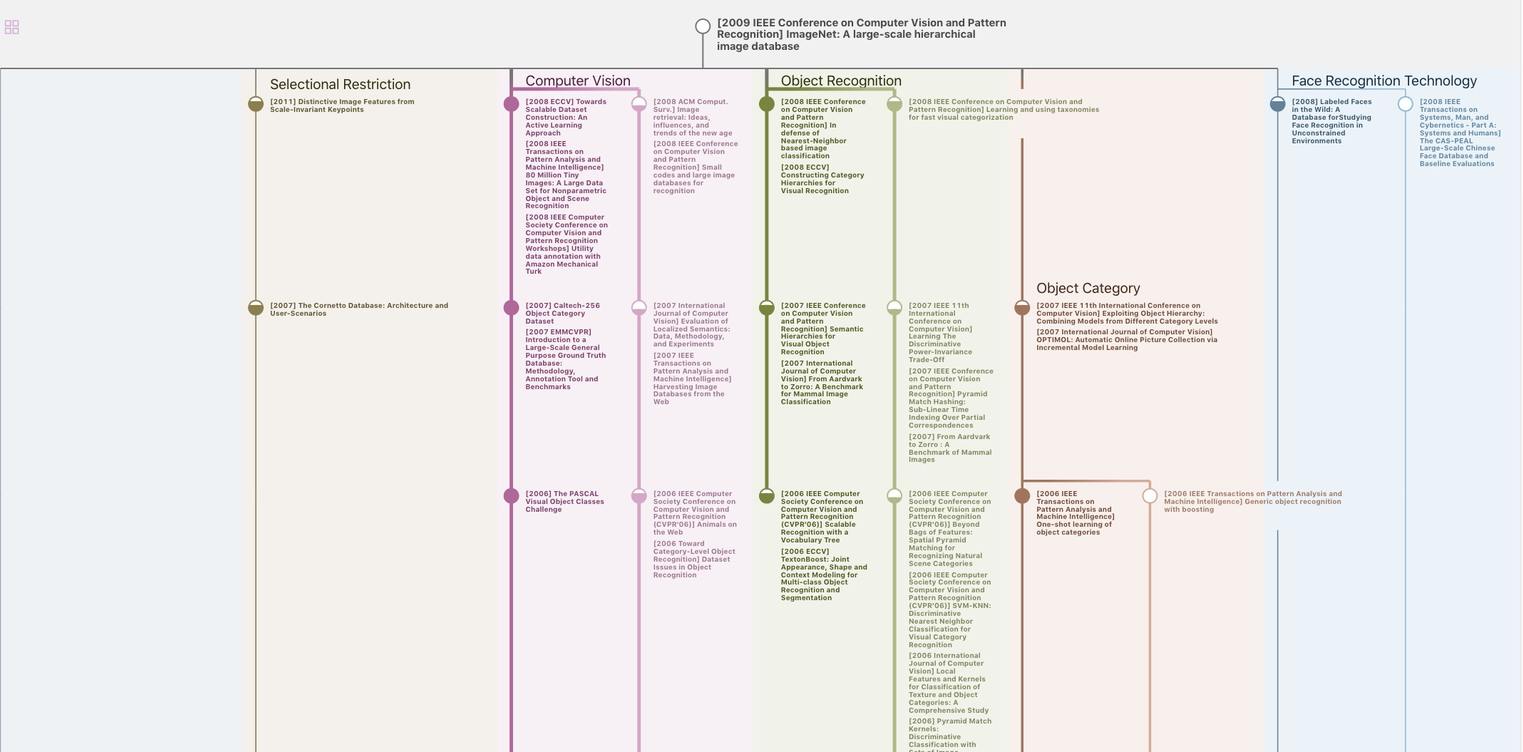
生成溯源树,研究论文发展脉络
Chat Paper
正在生成论文摘要