Implementing Active Learning in Cybersecurity: Detecting Anomalies in Redacted Emails
arxiv(2023)
摘要
Research on email anomaly detection has typically relied on specially prepared datasets that may not adequately reflect the type of data that occurs in industry settings. In our research, at a major financial services company, privacy concerns prevented inspection of the bodies of emails and attachment details (although subject headings and attachment filenames were available). This made labeling possible anomalies in the resulting redacted emails more difficult. Another source of difficulty is the high volume of emails combined with the scarcity of resources making machine learning (ML) a necessity, but also creating a need for more efficient human training of ML models. Active learning (AL) has been proposed as a way to make human training of ML models more efficient. However, the implementation of Active Learning methods is a human-centered AI challenge due to potential human analyst uncertainty, and the labeling task can be further complicated in domains such as the cybersecurity domain (or healthcare, aviation, etc.) where mistakes in labeling can have highly adverse consequences. In this paper we present research results concerning the application of Active Learning to anomaly detection in redacted emails, comparing the utility of different methods for implementing active learning in this context. We evaluate different AL strategies and their impact on resulting model performance. We also examine how ratings of confidence that experts have in their labels can inform AL. The results obtained are discussed in terms of their implications for AL methodology and for the role of experts in model-assisted email anomaly screening.
更多查看译文
关键词
active learning,detecting anomalies,cybersecurity
AI 理解论文
溯源树
样例
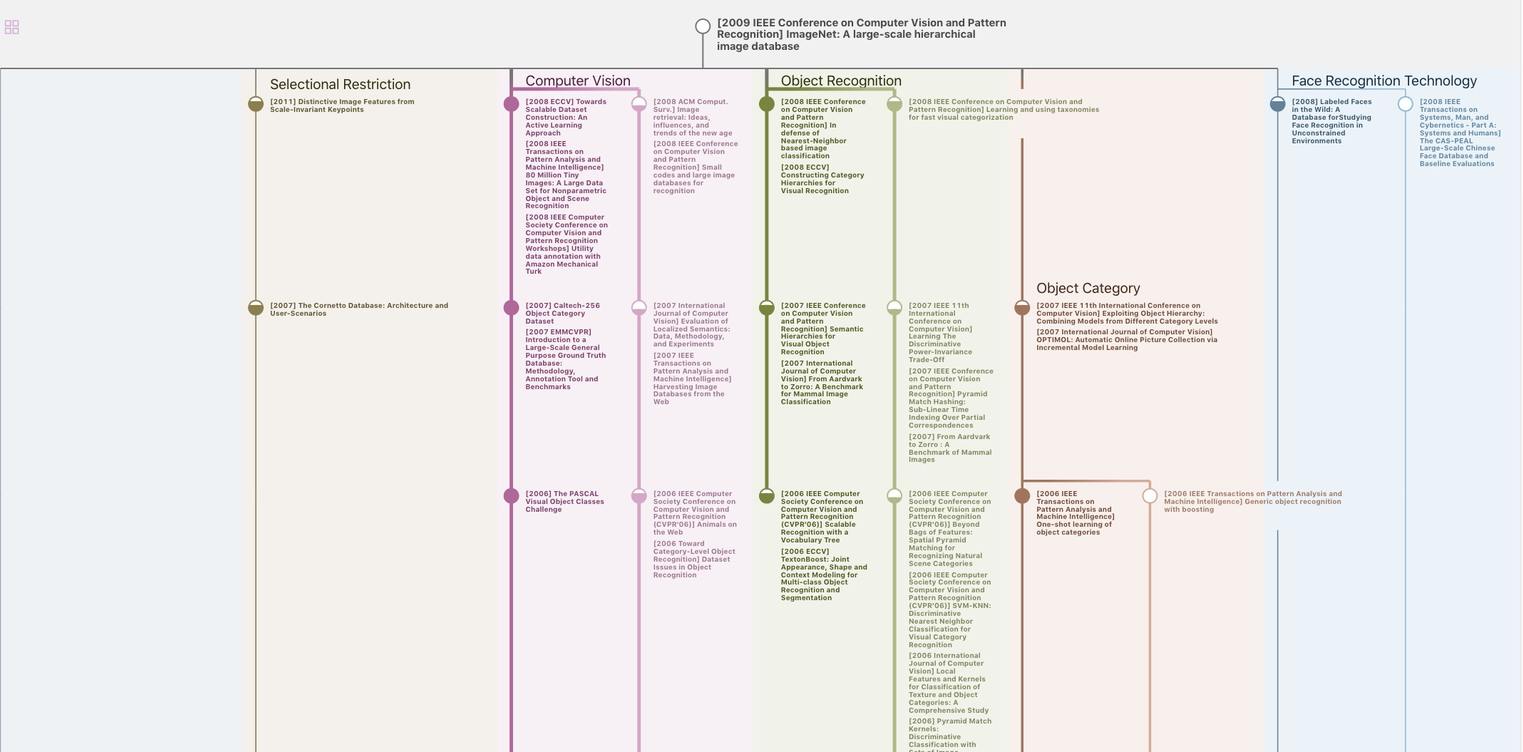
生成溯源树,研究论文发展脉络
Chat Paper
正在生成论文摘要