Continuous-Time Functional Diffusion Processes
arXiv (Cornell University)(2023)
摘要
We introduce functional diffusion processes (FDPs), which generalize traditional score-based diffusion models to infinite-dimensional function spaces. FDPs require a new mathematical framework to describe the forward and backward dynamics, and several extensions to derive practical training objectives. These include infinite-dimensional versions of the Girsanov theorem, in order to be able to compute an ELBO, and of the sampling theorem, in order to guarantee that functional evaluations in a countable set of points are equivalent to infinite-dimensional functions. We use FDPs to build a new breed of generative models in function spaces, which do not require specialized network architectures, and that can work with any kind of continuous data. Our results on synthetic and real data illustrate the advantages of FDPs in simplifying the design requirements of diffusion models.
更多查看译文
关键词
diffusion,processes,functional,continuous-time
AI 理解论文
溯源树
样例
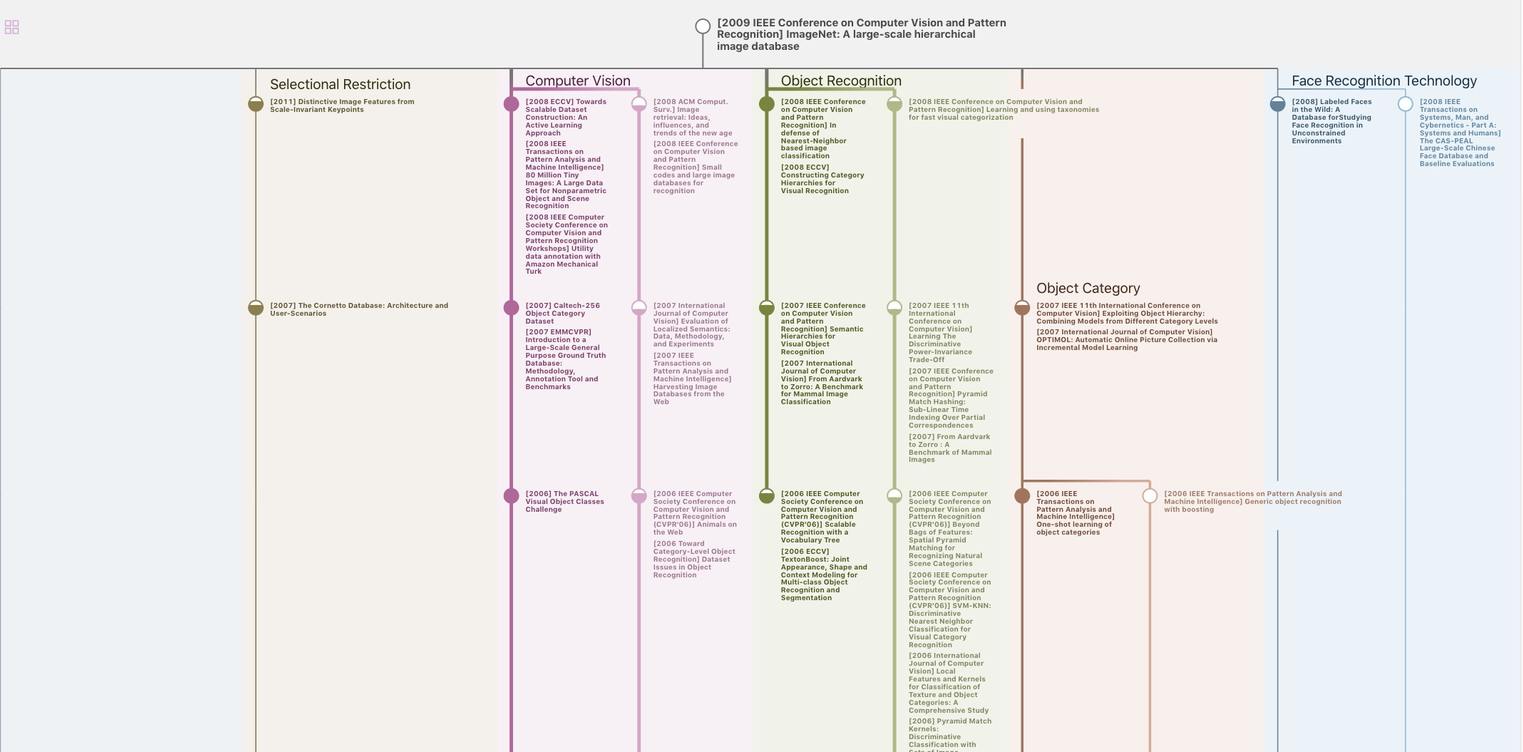
生成溯源树,研究论文发展脉络
Chat Paper
正在生成论文摘要