Improved Segmentation of Deep Sulci in Cortical Gray Matter Using a Deep Learning Framework Incorporating Laplace's Equation
Lecture Notes in Computer Science(2023)
摘要
When developing tools for automated cortical segmentation, the ability to produce topologically correct segmentations is important in order to compute geometrically valid morphometry measures. In practice, accurate cortical segmentation is challenged by image artifacts and the highly convoluted anatomy of the cortex itself. To address this, we propose a novel deep learning-based cortical segmentation method in which prior knowledge about the geometry of the cortex is incorporated into the network during the training process. We design a loss function which uses the theory of Laplace's equation applied to the cortex to locally penalize unresolved boundaries between tightly folded sulci. Using an ex vivo MRI dataset of human medial temporal lobe specimens, we demonstrate that our approach outperforms baseline segmentation networks, both quantitatively and qualitatively.
更多查看译文
关键词
cortical gray matter,deep sulci,deep learning
AI 理解论文
溯源树
样例
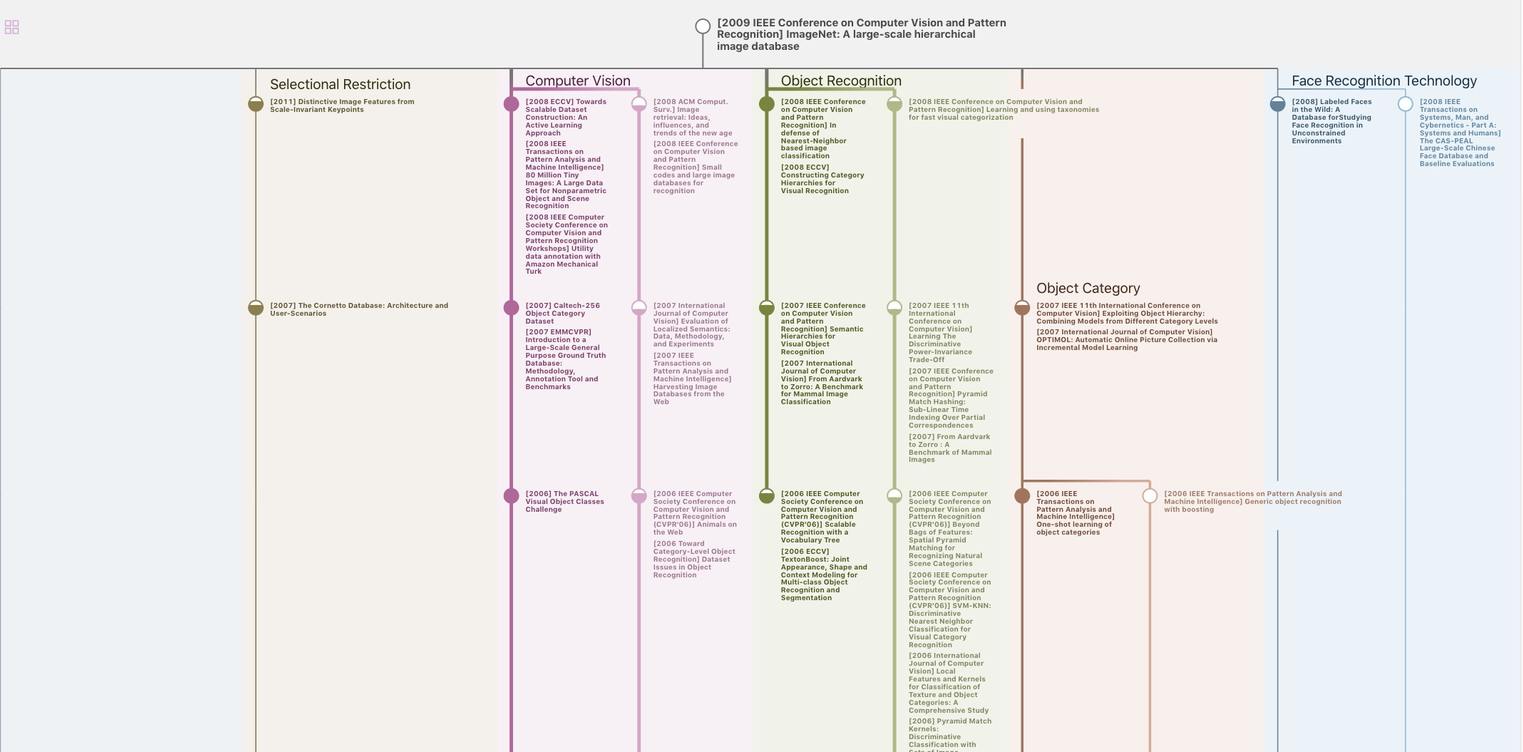
生成溯源树,研究论文发展脉络
Chat Paper
正在生成论文摘要