Temporal Fusion Based 1-D Sequence Semantic Segmentation Model for Automatic Precision Side Scan Sonar Bottom Tracking
IEEE Transactions on Geoscience and Remote Sensing(2023)
摘要
Precision bottom tracking is a core step in the data processing of side scan sonar (SSS), which is of critical value for the quality of the final SSS production. Currently, the automatic precision SSS bottom tracking remains challenging due to the complex noise caused by the measuring environment; especially, the existing methods did not fully exploit the temporal correlations or depended on the hand-crafted setting. Therefore, we proposed a novel temporal fusion-based 1-D sequence semantic segmentation model, TFSSM–1–D, to fuse the temporal correlation features and perform automatic precision SSS bottom tracking. The TFSSM–1–D uses the deep learning (DL) encoder–decoder model for mapping inputs to 1-D semantic label outputs, with the aid of preprocess and temporal fusion modules, to improve the accuracy and robustness. Among them, preprocess module is used to introduce the prior knowledge of bilateral symmetry, which alleviates the defect of long-distance features correlation caused by the inductive bias of convolution neural network (CNN). The temporal fusion consists of the point-wise temporal fusion module (PTFM), and the bi-directional attention propagation module (BAPM) guides the model to explicitly fuse the temporal variation features on different scales. The experimental results demonstrate the effectiveness of TFSSM–1–D, and its mean port offset error (MPOE) reaches 2.7058 on the testing set without downsampling, which is 40% lower than the previous DL based model with single ping input, and other evaluation metrics have also significantly improved. The inference on unseen data shows that TFSSM–1–D can achieve precision bottom tracking with good noise immunity.
更多查看译文
关键词
1-D sequence,bottom tracking,deep learning (DL),semantic segmentation,side scan sonar,temporal fusion
AI 理解论文
溯源树
样例
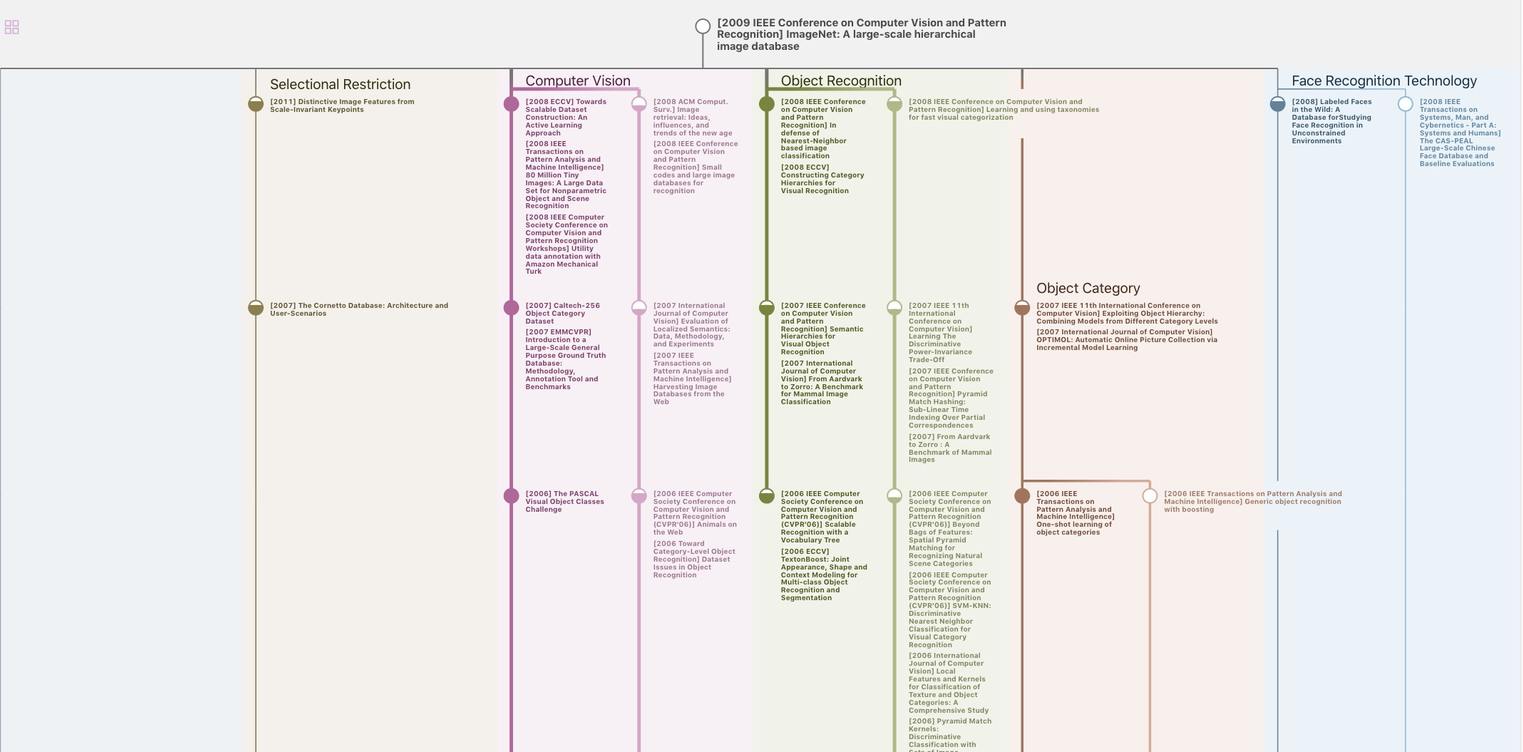
生成溯源树,研究论文发展脉络
Chat Paper
正在生成论文摘要