Transportation Object Counting With Graph-Based Adaptive Auxiliary Learning
IEEE Transactions on Intelligent Transportation Systems(2023)
摘要
This paper proposes an adaptive auxiliary task learning-based approach for transport object counting problems such as humans and vehicles. These problems are essential in many real-world tasks such as video surveillance, traffic monitoring, public security, and urban planning, to aid intelligent transportation systems. Unlike existing auxiliary task learning-based methods, we develop an attention-enhanced adaptively shared backbone network to enable both task-shared and task-tailored features that are learned in an end-to-end manner. The network seamlessly combines a standard Convolution Neural Network (CNN) and a Graph Convolution Network (GCN) for feature extraction and feature reasoning among different domains of tasks. Our approach gains enriched contextual information by iteratively and hierarchically fusing features across different task branches of the adaptive CNN backbone. The whole framework pays special attention to objects’ spatial locations and varied density levels, informed by object (or crowd) segmentation and density level segmentation auxiliary tasks. In particular, thanks to the proposed dilated contrastive density loss function, our network benefits from individual and regional context supervision, along with strengthened robustness. Experiments on six challenging multi-domain datasets demonstrate that our method achieves superior performance compared with state-of-the-art auxiliary task learning-based counting methods. Our code is publicly available.
更多查看译文
关键词
Object counting,GCN,dilated contrastive density loss,adaptive auxiliary task
AI 理解论文
溯源树
样例
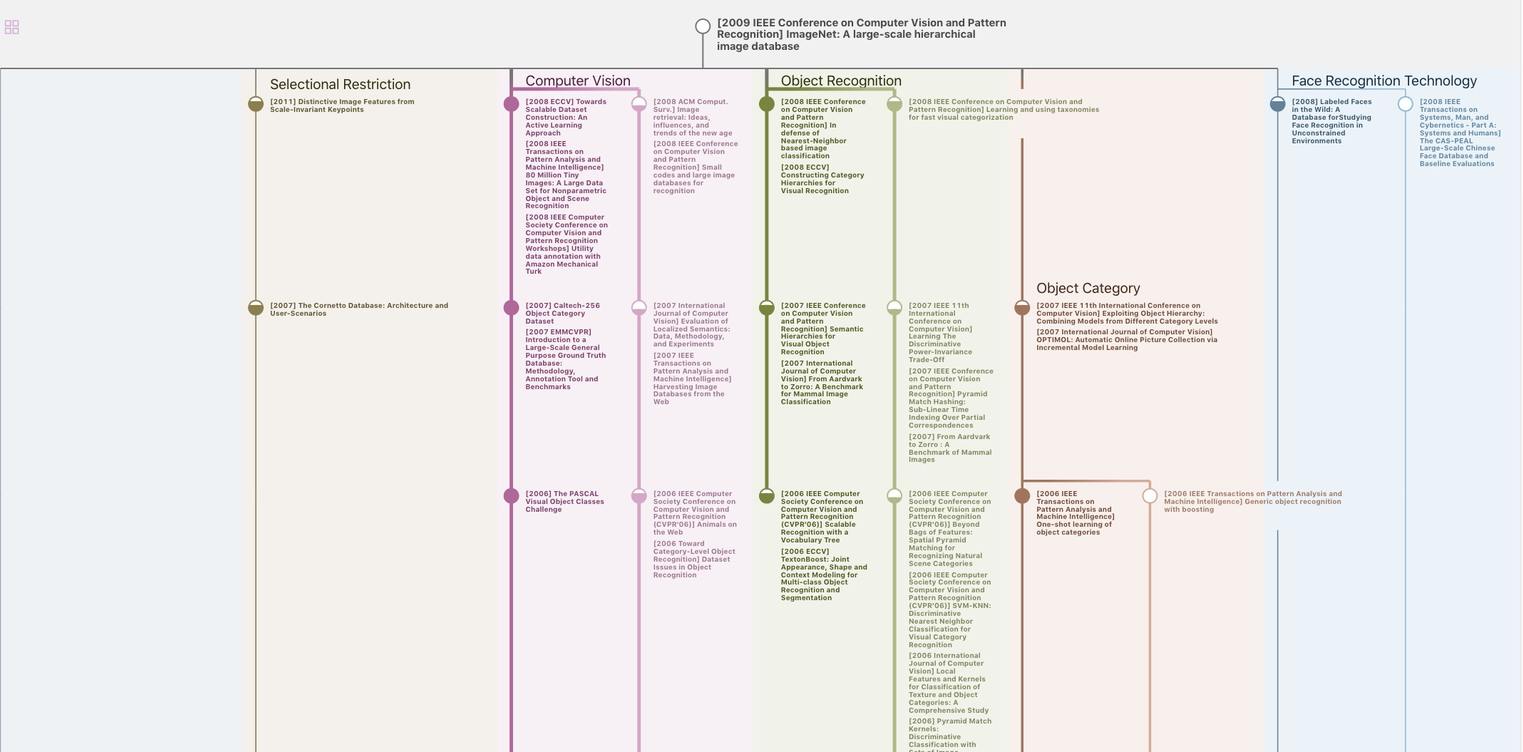
生成溯源树,研究论文发展脉络
Chat Paper
正在生成论文摘要