One Step of Gait Information From Sensing Walking Surface for Personal Identification
IEEE Sensors Journal(2023)
摘要
The accuracy of gait sensing detection and identification determines the usability and acceptability in field applications. Four inertial measurement units (IMUs) recorded from heel contact to toe-off as a footstep in gait. The 1400 footsteps from 70 participants were collected, and the 13 footsteps from each were randomly selected for the Siamese convolutional neural network (SCNN) model training. The inputs in the SCNN model were a matrix of 4 (sensor)
$\times150$
(sample) with six channels, which were associated with three axes of acceleration and gyroscopes of one footstep. Subsequently, different numbers of enrolled people were used in the training model, and different footsteps were set as a threshold for ingroup or imposter detections. Even in the smallest number of enrolled people dataset in ingroup detection, the performance of the SCNN was significantly better than the long short-term memory (LSTM). The highest accuracy rate of ingroup detection was 96.33% in ten enrolled people, and the highest for imposter detection was 81.93% in 60 enrolled people under the 13 footsteps threshold. The best accuracy rate was in 30 enrolled people when employed ingroup and imposter detections together, significantly higher than the other enrolled numbers. The present study highlighted two contributions of an embedded IMU surface measurement system in gait information collection. First, only 13 steps of each participant were required in training, which was considered a minimal data input. Second, only one footstep achieved very promising personal identification for each person in the ingroup population and imposter detection, making our system highly applicable to gait biometrics.
更多查看译文
关键词
Gait pattern recognition,imposter detection,inertial measurement unit (IMU),personal identification,Siamese convolutional neural network (SCNN)
AI 理解论文
溯源树
样例
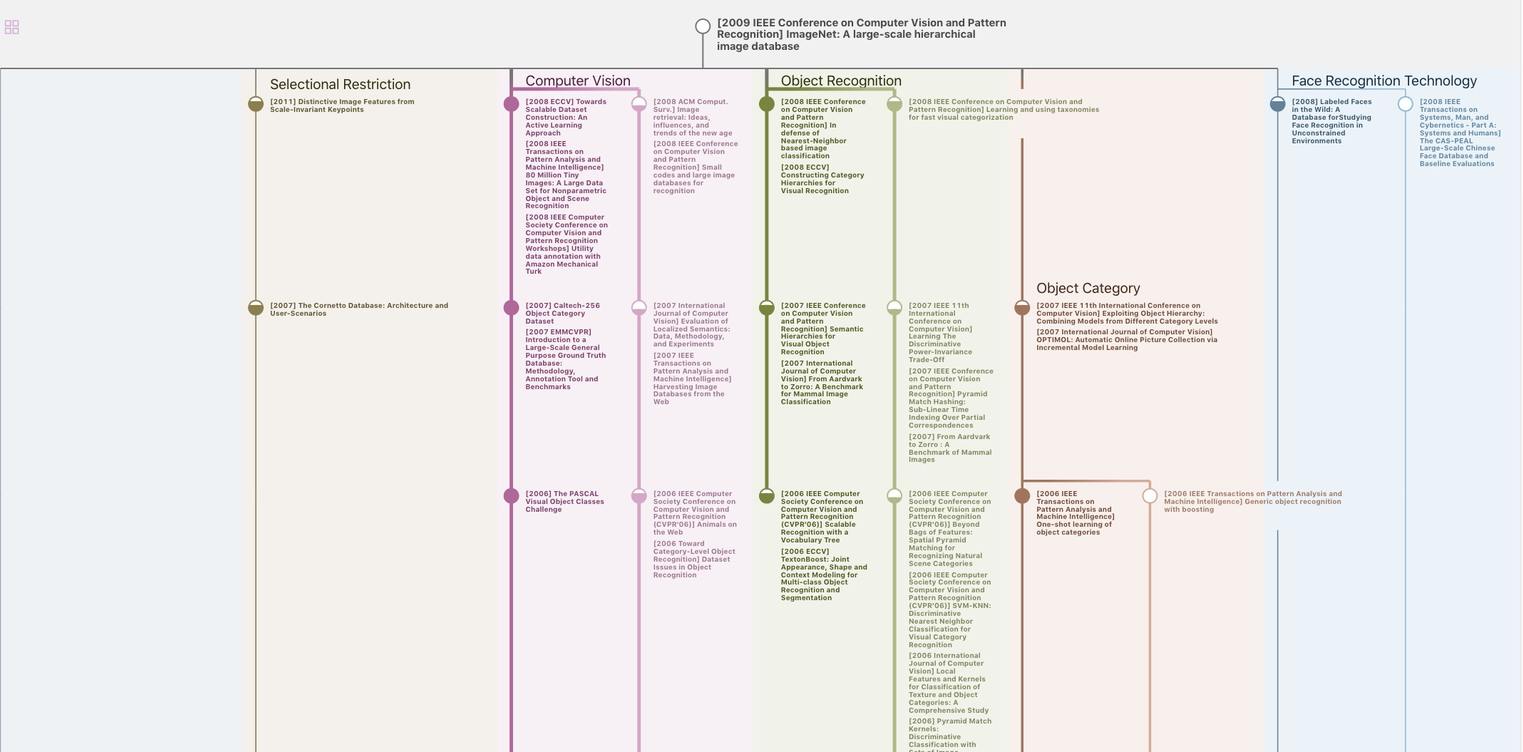
生成溯源树,研究论文发展脉络
Chat Paper
正在生成论文摘要