Vehicle Color Identification Framework using Pixel-level Color Estimation from Segmentation Masks of Car Parts
2022 IEEE 5th International Conference on Image Processing Applications and Systems (IPAS)(2022)
摘要
Color comprises one of the most significant and dominant cues for various applications. As one of the most noticeable and stable attributes of vehicles, color can constitute a valuable key component in several practices of intelligent surveillance systems. In this paper, we propose a deep-learning-based framework that combines semantic segmentation masks with pixels clustering for automatic vehicle color recognition. Different from conventional methods, which usually consider only the features of the vehicle's front side, the proposed algorithm is able for view-independent color identification, which is more effective for the surveillance tasks. To the best of our knowledge, this is the first work that employs semantic segmentation masks along with color clustering for the extraction of the vehicle's color representative parts and the recognition of the dominant color, respectively. To evaluate the performance of the proposed method, we introduce a challenging multi-view dataset of 500 car-related RGB images extending the publicly available DSMLR Car Parts dataset for vehicle parts segmentation. The experiments demonstrate that the proposed approach achieves excellent performance and accurate results reaching an accuracy of 93.06% in the multi-view scenario. To facilitate further research, the evaluation dataset and the pre-trained models will be released at https://github.com/klearchos-stav/vehicle_color_recognition.
更多查看译文
关键词
vehicle color identification,semantic segmentation,intelligent surveillance
AI 理解论文
溯源树
样例
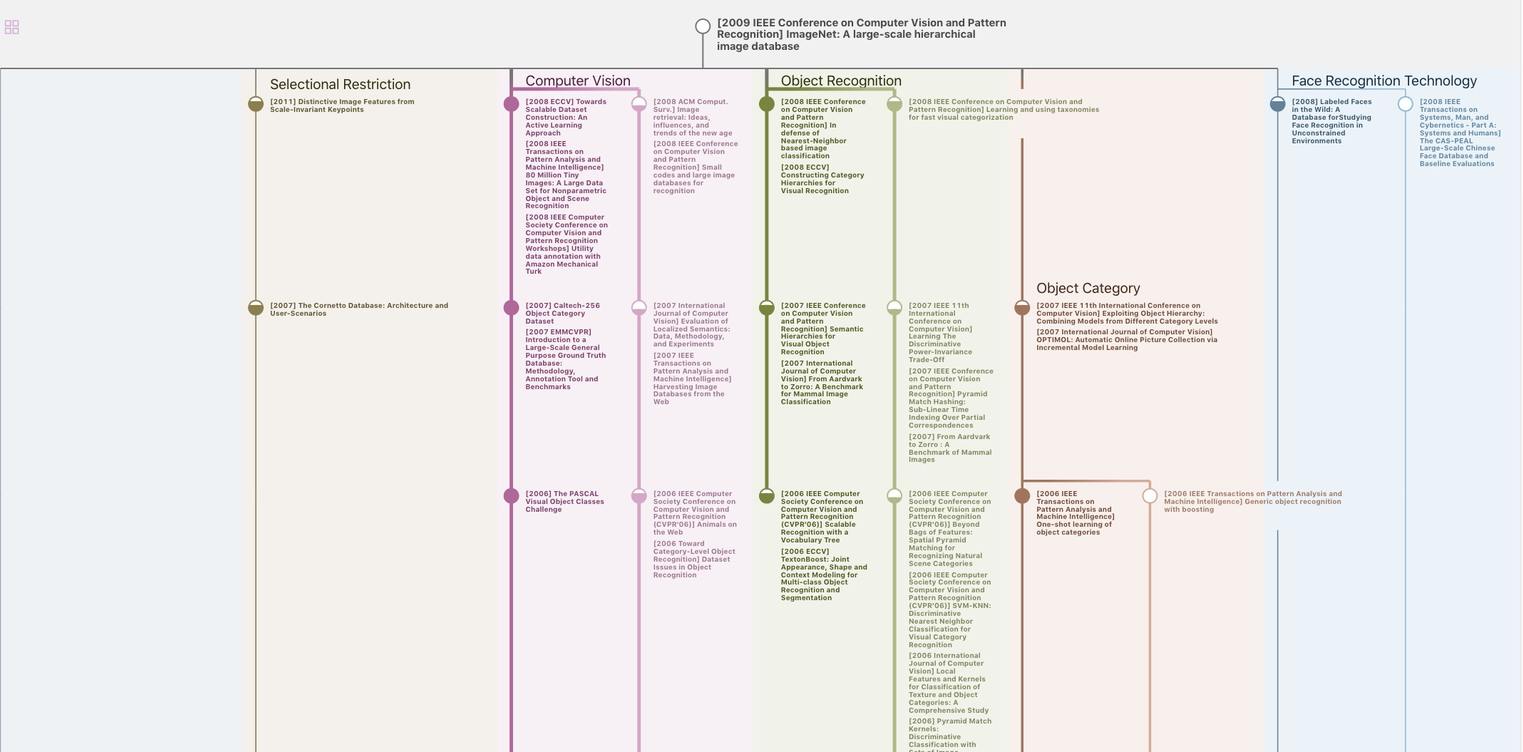
生成溯源树,研究论文发展脉络
Chat Paper
正在生成论文摘要