A Reinforcement Learning Handover Parameter Adaptation Method Based on LSTM-Aided Digital Twin for UDN.
Sensors (Basel, Switzerland)(2023)
摘要
Adaptation of handover parameters in ultra-dense networks has always been one of the key issues in optimizing network performance. Aiming at the optimization goal of effective handover ratio, this paper proposes a deep Q-learning (DQN) method that dynamically selects handover parameters according to wireless signal fading conditions. This approach seeks good backward compatibility. In order to enhance the efficiency and performance of the DQN method, Long Short Term Memory (LSTM) is used to build a digital twin and assist the DQN algorithm to achieve a more efficient search. Simulation experiments prove that the enhanced method has a faster convergence speed than the ordinary DQN method, and at the same time, achieves an average effective handover ratio increase of 2.7%. Moreover, in different wireless signal fading intervals, the method proposed in this paper has achieved better performance.
更多查看译文
关键词
deep Q-learning,digital twin,handover parameters,long short-term memory,ultra-dense network
AI 理解论文
溯源树
样例
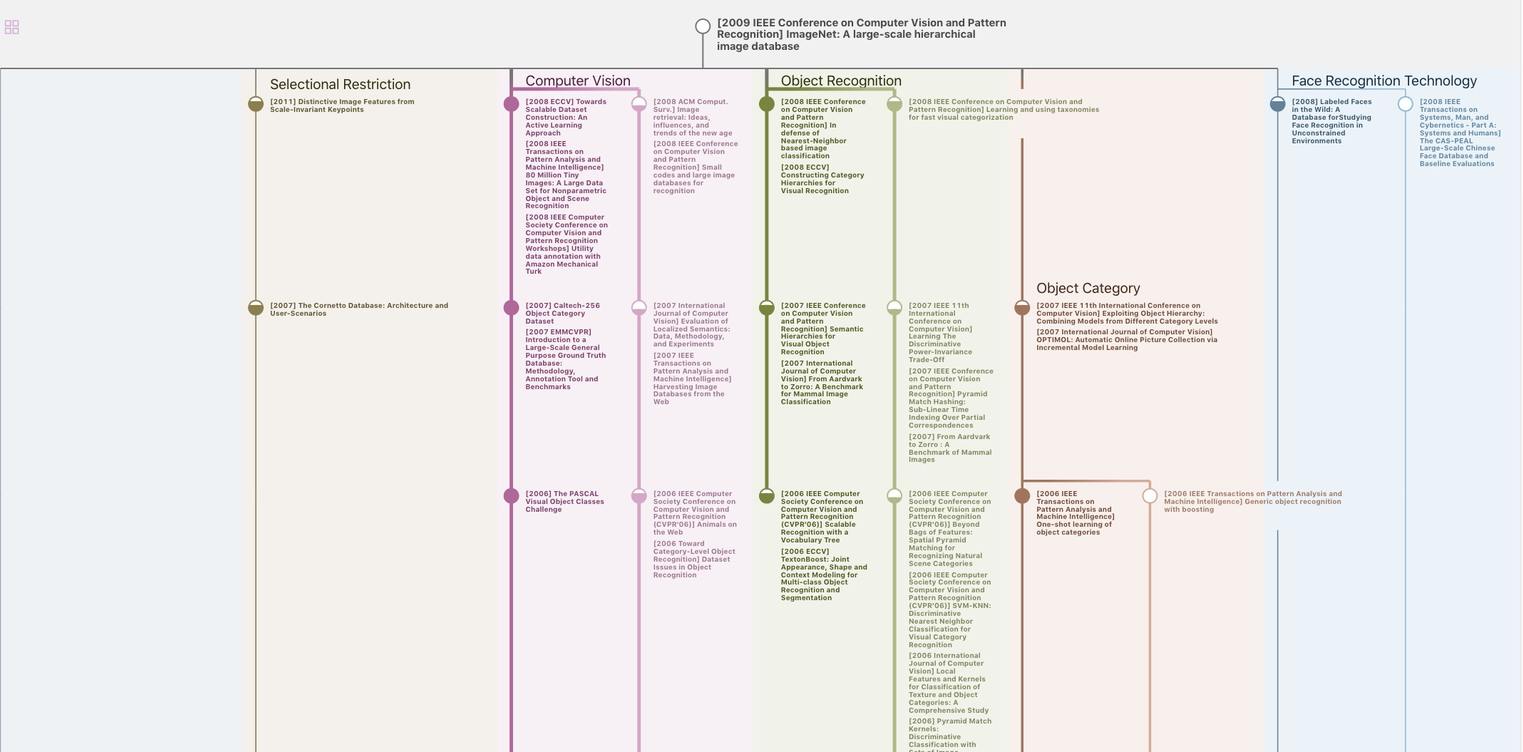
生成溯源树,研究论文发展脉络
Chat Paper
正在生成论文摘要