Failure Identification Using Model-Implemented Fault Injection with Domain Knowledge-Guided Reinforcement Learning.
Sensors (Basel, Switzerland)(2023)
摘要
The safety assessment of cyber-physical systems (CPSs) requires tremendous effort, as the complexity of cyber-physical systems is increasing. A well-known approach for the safety assessment of CPSs is fault injection (FI). The goal of fault injection is to find a catastrophic fault that can cause the system to fail by injecting faults into it. These catastrophic faults are less likely to occur, and finding them requires tremendous labor and cost. In this study, we propose a reinforcement learning (RL)-based method to automatically configure faults in the system under test and to find catastrophic faults in the early stage of system development at the model level. The proposed method provides a guideline to utilize high-level domain knowledge about a system model for constructing the reinforcement learning agent and fault injection setup. In this study, we used the system (safety) specification to shape the reward function in the reinforcement learning agent. The reinforcement learning agent dynamically interacted with the model under test to identify catastrophic faults. We compared the proposed method with random-based fault injection in two case studies using MATLAB/Simulink. Our proposed method outperformed random-based fault injection in terms of the severity and number of faults found.
更多查看译文
关键词
domain knowledge,fault identification,fault injection,reinforcement learning,safety assessment,signal temporal logic
AI 理解论文
溯源树
样例
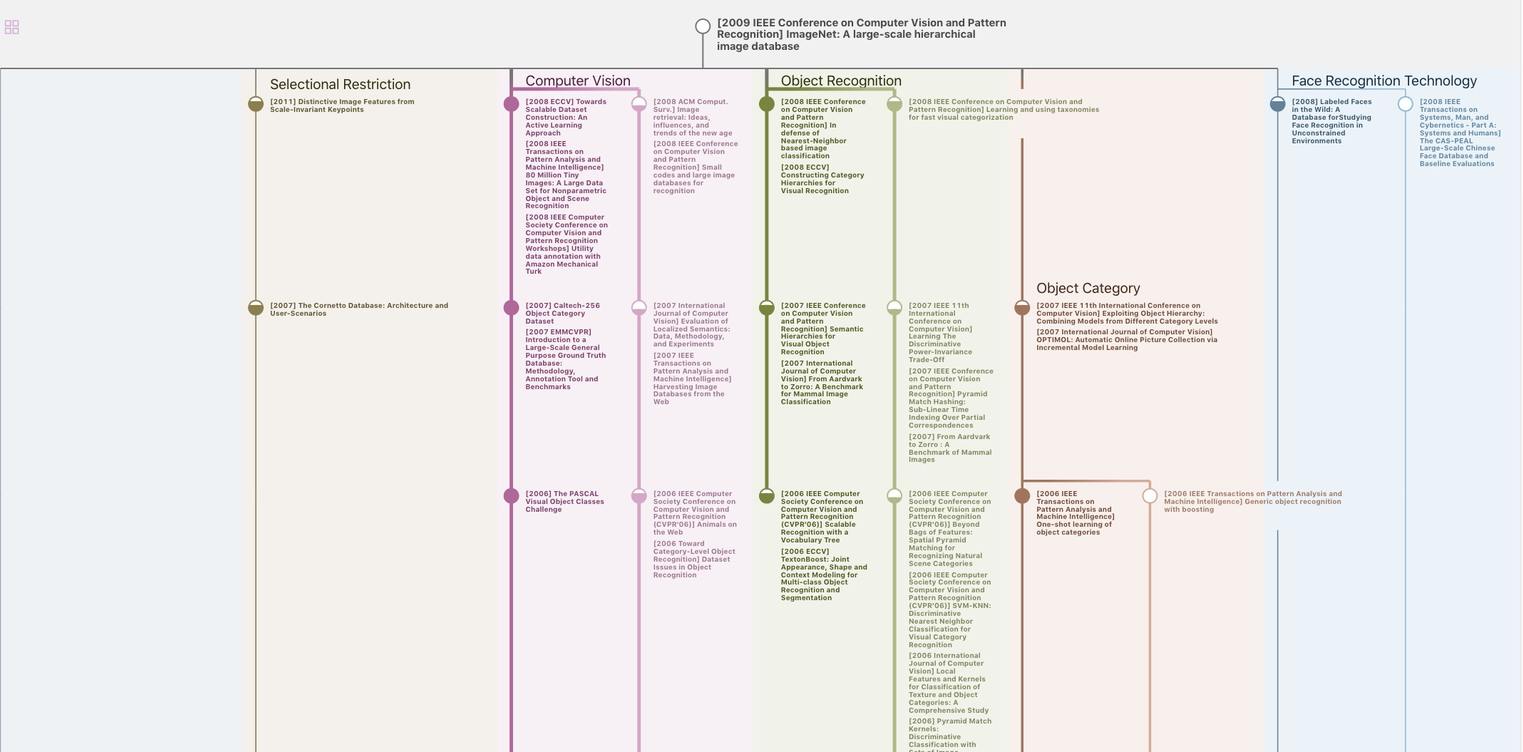
生成溯源树,研究论文发展脉络
Chat Paper
正在生成论文摘要