Event-Guided Image Super-Resolution Reconstruction.
Sensors (Basel, Switzerland)(2023)
摘要
The event camera efficiently detects scene radiance changes and produces an asynchronous event stream with low latency, high dynamic range (HDR), high temporal resolution, and low power consumption. However, the large output data caused by the asynchronous imaging mechanism makes the increase in spatial resolution of the event camera limited. In this paper, we propose a novel event camera super-resolution (SR) network (EFSR-Net) based on a deep learning approach to address the problems of low spatial resolution and poor visualization of event cameras. The network model is capable of reconstructing high-resolution (HR) intensity images using event streams and active sensor pixel (APS) frame information. We design the coupled response blocks (CRB) in the network that are able of fusing the feature information of both data to achieve the recovery of detailed textures in the shadows of real images. We demonstrate that our method is able to reconstruct high-resolution intensity images with more details and less blurring in synthetic and real datasets, respectively. The proposed EFSR-Net can improve the peak signal-to-noise ratio (PSNR) metric by 1-2 dB compared with state-of-the-art methods.
更多查看译文
关键词
deep learning,event cameras,image reconstruction,super-resolution
AI 理解论文
溯源树
样例
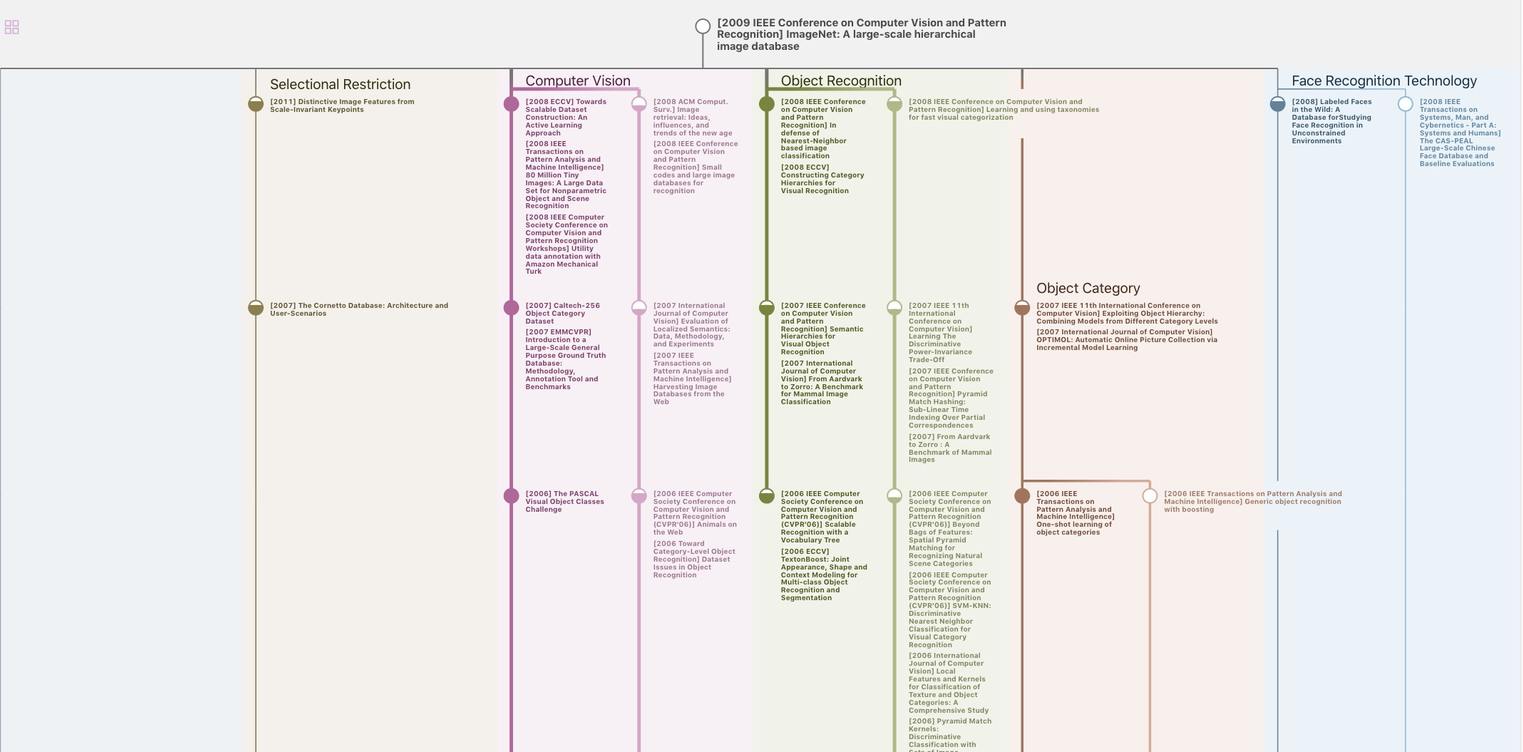
生成溯源树,研究论文发展脉络
Chat Paper
正在生成论文摘要