Soft-Search: Two Datasets to Study the Identification and Production of Research Software
arxiv(2023)
摘要
Software is an important tool for scholarly work, but software produced for research is in many cases not easily identifiable or discoverable. A potential first step in linking research and software is software identification. In this paper we present two datasets to study the identification and production of research software. The first dataset contains almost 1000 human labeled annotations of software production from National Science Foundation (NSF) awarded research projects. We use this dataset to train models that predict software production. Our second dataset is created by applying the trained predictive models across the abstracts and project outcomes reports for all NSF funded projects between the years of 2010 and 2023. The result is an inferred dataset of software production for over 150,000 NSF awards. We release the Soft-Search dataset to aid in identifying and understanding research software production: https://github.com/si2-urssi/eager
更多查看译文
关键词
datasets,text classification,research software
AI 理解论文
溯源树
样例
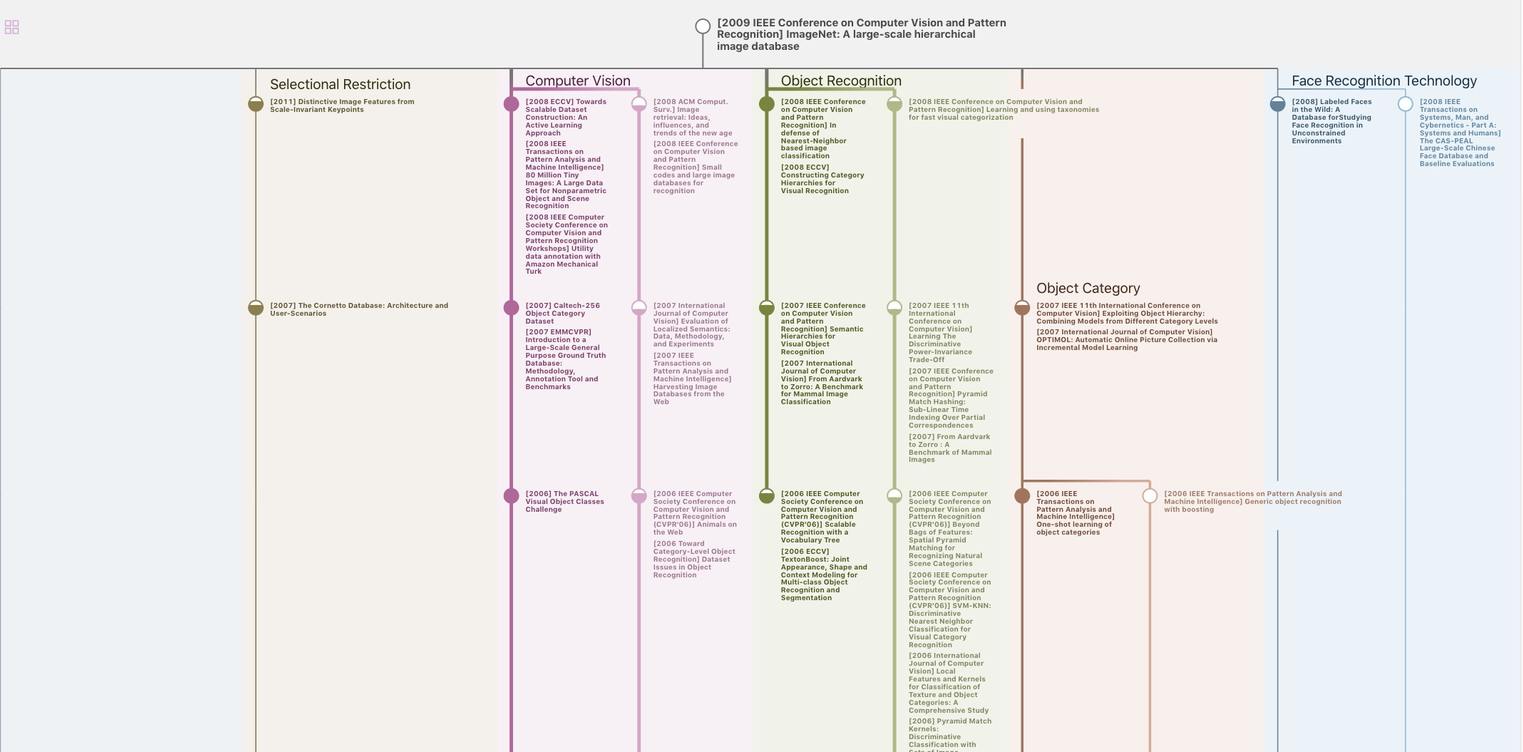
生成溯源树,研究论文发展脉络
Chat Paper
正在生成论文摘要