Reinforcement Learning with Depreciating Assets
AAMAS(2023)
摘要
A basic assumption of traditional reinforcement learning is that the value of a reward does not change once it is received by an agent. The present work forgoes this assumption and considers the situation where the value of a reward decays proportionally to the time elapsed since it was obtained. Emphasizing the inflection point occurring at the time of payment, we use the term asset to refer to a reward that is currently in the possession of an agent. Adopting this language, we initiate the study of depreciating assets within the framework of infinite-horizon quantitative optimization. In particular, we propose a notion of asset depreciation, inspired by classical exponential discounting, where the value of an asset is scaled by a fixed discount factor at each time step after it is obtained by the agent. We formulate a Bellman-style equational characterization of optimality in this context and develop a model-free reinforcement learning approach to obtain optimal policies.
更多查看译文
关键词
depreciating assets,reinforcement learning
AI 理解论文
溯源树
样例
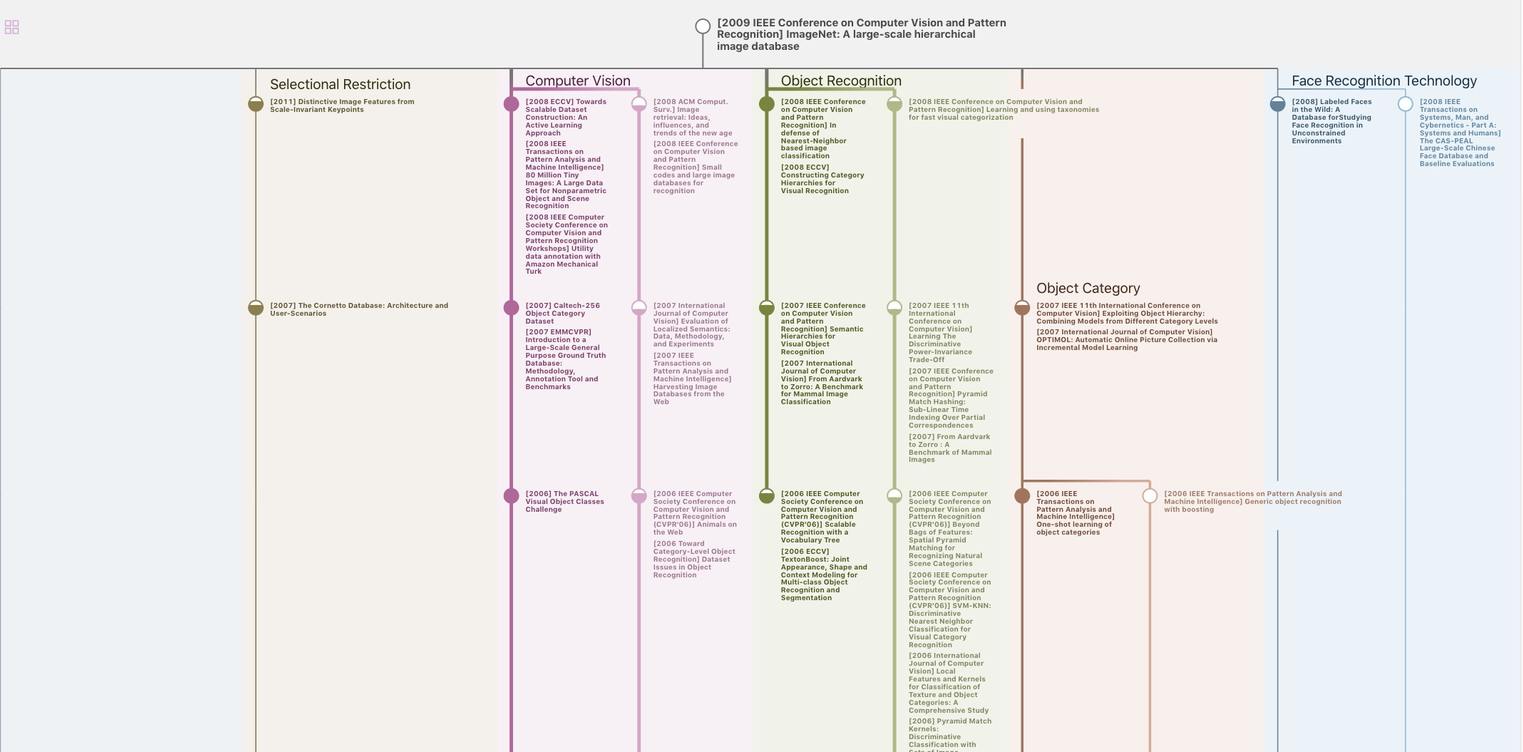
生成溯源树,研究论文发展脉络
Chat Paper
正在生成论文摘要