Taylor TD-learning
NeurIPS(2023)
摘要
Many reinforcement learning approaches rely on temporal-difference (TD) learning to learn a critic. However, TD-learning updates can be high variance due to their sole reliance on Monte Carlo estimates of the updates. Here, we introduce a model-based RL framework, Taylor TD, which reduces this variance. Taylor TD uses a first-order Taylor series expansion of TD updates. This expansion allows to analytically integrate over stochasticity in the action-choice, and some stochasticity in the state distribution for the initial state and action of each TD update. We include theoretical and empirical evidence of Taylor TD updates being lower variance than (standard) TD updates. Additionally, we show that Taylor TD has the same stable learning guarantees as (standard) TD-learning under linear function approximation. Next, we combine Taylor TD with the TD3 algorithm (Fujimoto et al., 2018), into TaTD3. We show TaTD3 performs as well, if not better, than several state-of-the art model-free and model-based baseline algorithms on a set of standard benchmark tasks. Finally, we include further analysis of the settings in which Taylor TD may be most beneficial to performance relative to standard TD-learning.
更多查看译文
关键词
td-learning
AI 理解论文
溯源树
样例
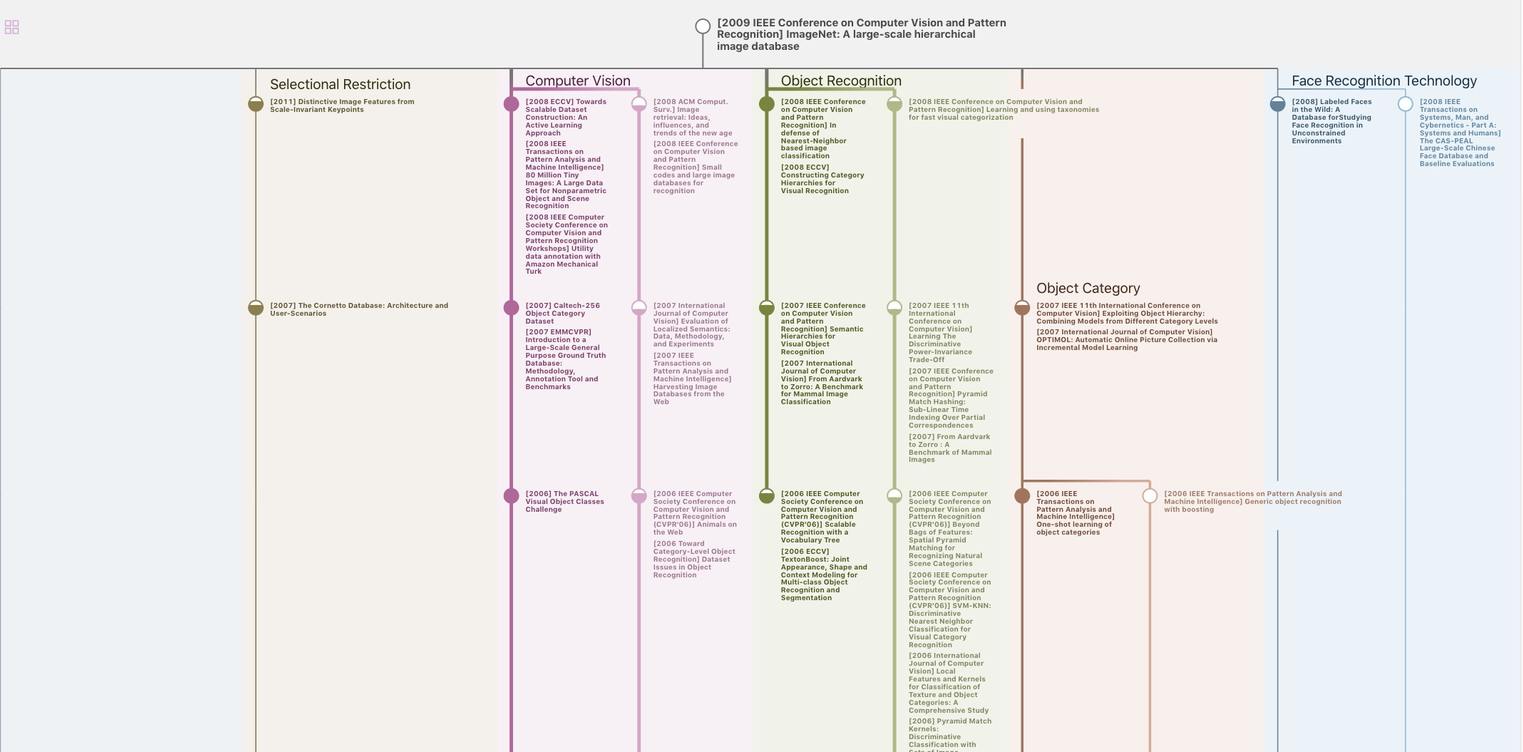
生成溯源树,研究论文发展脉络
Chat Paper
正在生成论文摘要