Low-Complexity Blind Parameter Estimation in Wireless Systems with Noisy Sparse Signals
IEEE TRANSACTIONS ON WIRELESS COMMUNICATIONS(2023)
摘要
Baseband processing algorithms often require knowledge of the noise power, signal power, or signal-to-noise ratio (SNR). In practice, these parameters are typically unknown and must be estimated. Furthermore, the mean-square error (MSE) is a desirable metric to be minimized in a variety of estimation and signal recovery algorithms. However, the MSE cannot directly be used as it depends on the true signal that is generally unknown to the estimator. In this paper, we propose novel blind estimators for the average noise power, average receive signal power, SNR, and MSE. The proposed estimators can be computed at low complexity and solely rely on the large-dimensional and sparse nature of the processed data. Our estimators can be used (i) to quickly track some of the key system parameters while avoiding additional pilot overhead, (ii) to design low-complexity nonparametric algorithms that require such quantities, and (iii) to accelerate more sophisticated estimation or recovery algorithms. We conduct a theoretical analysis of the proposed estimators for a Bernoulli complex Gaussian (BCG) prior, and we demonstrate their efficacy via synthetic experiments. We also provide three application examples that deviate from the BCG prior in millimeter-wave multi-antenna and cell-free wireless systems for which we develop nonparametric denoising algorithms that improve channel-estimation accuracy with a performance comparable to denoisers that assume perfect knowledge of the system parameters.
更多查看译文
关键词
Blind parameter estimation,channel-vector denoising,sparsity,Stein's unbiased risk estimator (SURE),variance estimation,wireless communication
AI 理解论文
溯源树
样例
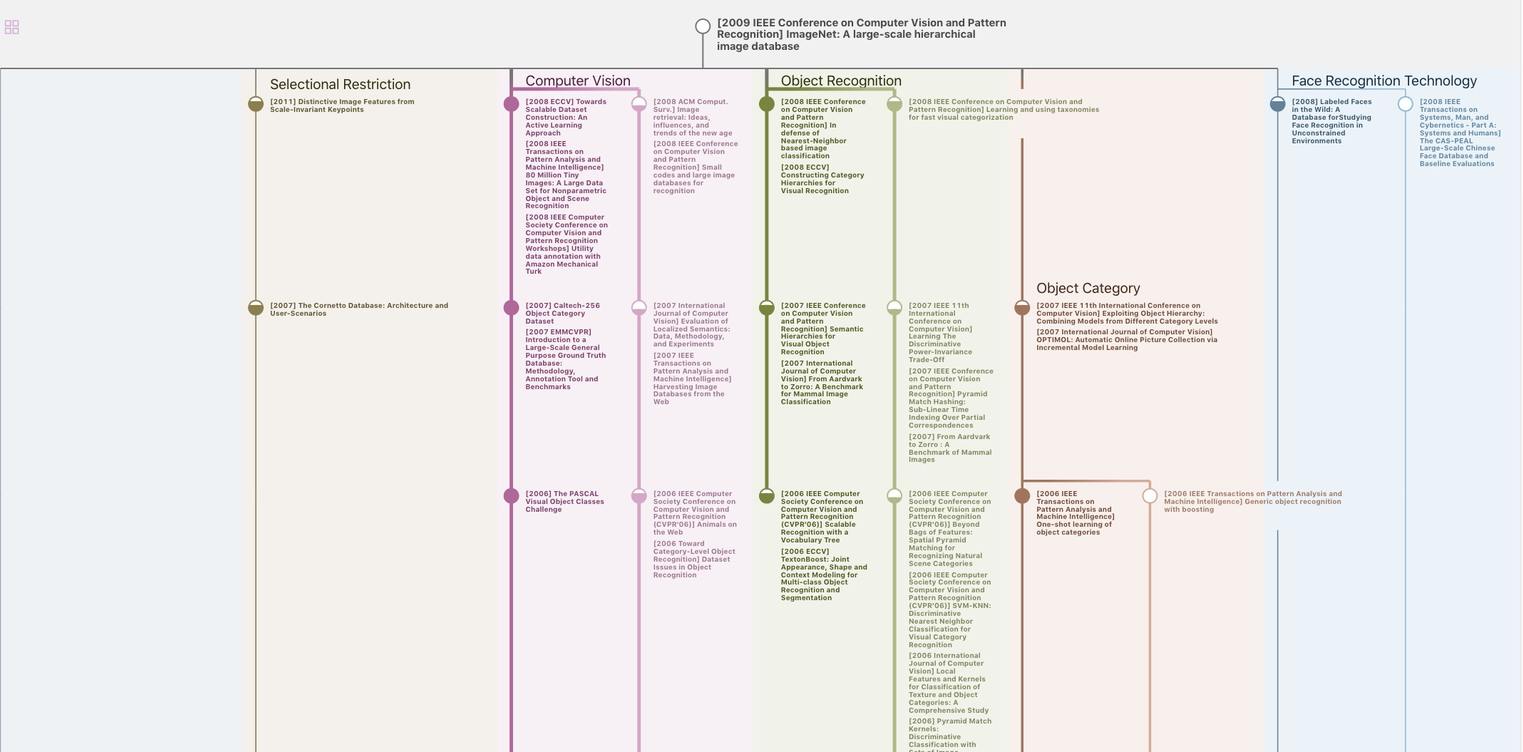
生成溯源树,研究论文发展脉络
Chat Paper
正在生成论文摘要