Research on Intelligent Identification and Grading of Nonmetallic Inclusions in Steels Based on Deep Learning.
Micromachines(2023)
摘要
Non-metallic inclusions are unavoidable defects in steel, and their type, quantity, size, and distribution have a great impact on the quality of steel. At present, non-metallic inclusions are mainly detected manually, which features high work intensity, low efficiency, proneness to misjudgment, and low consistency of results. In this paper, based on deep neural network algorithm, a small number of manually labeled, low-resolution metallographic images collected by optical microscopes are used as the dataset for intelligent boundary extraction, classification, and rating of non-metallic inclusions. The training datasets are cropped into those containing only a single non-metallic inclusion to reduce the interference of background information and improve the accuracy. To deal with the unbalanced distribution of each category of inclusions, the reweighting cross entropy loss and focal loss are respectively used as the category prediction loss and boundary prediction loss of the DeepLabv3+ semantic segmentation model. Finally, the length and width of the minimum enclosing rectangle of the segmented inclusions are measured to calculate the grade of inclusions. The resulting accuracy is 90.34% in segmentation and 90.35% in classification. As is verified, the model-based rating results are consistent with those of manual labeling. For a single sample, the detection time is reduced from 30 min to 15 s, significantly improving the detection efficiency.
更多查看译文
关键词
deep learning,intelligent rating,non-metallic inclusions,segmentation and classification
AI 理解论文
溯源树
样例
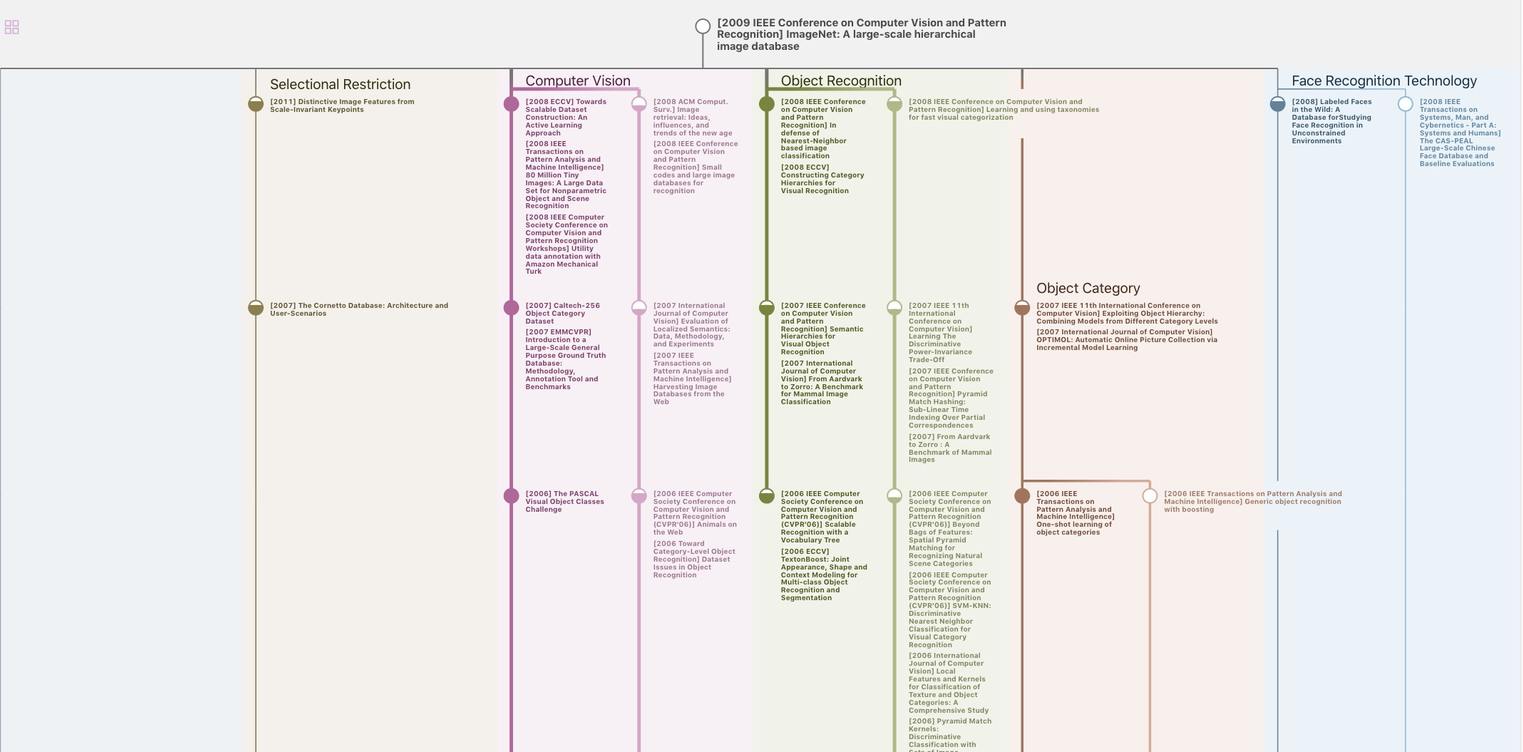
生成溯源树,研究论文发展脉络
Chat Paper
正在生成论文摘要