Developing a Prediction Model of Demolition-Waste Generation-Rate via Principal Component Analysis.
International journal of environmental research and public health(2023)
摘要
Construction and demolition waste accounts for a sizable proportion of global waste and is harmful to the environment. Its management is therefore a key challenge in the construction industry. Many researchers have utilized waste generation data for waste management, and more accurate and efficient waste management plans have recently been prepared using artificial intelligence models. Here, we developed a hybrid model to forecast the demolition-waste-generation rate in redevelopment areas in South Korea by combining principal component analysis (PCA) with decision tree, k-nearest neighbors, and linear regression algorithms. Without PCA, the decision tree model exhibited the highest predictive performance ( = 0.872) and the k-nearest neighbors (Chebyshev distance) model exhibited the lowest ( = 0.627). The hybrid PCA-k-nearest neighbors (Euclidean uniform) model exhibited significantly better predictive performance ( = 0.897) than the non-hybrid k-nearest neighbors (Euclidean uniform) model ( = 0.664) and the decision tree model. The mean of the observed values, k-nearest neighbors (Euclidean uniform) and PCA-k-nearest neighbors (Euclidean uniform) models were 987.06 (kg·m), 993.54 (kg·m) and 991.80 (kg·m), respectively. Based on these findings, we propose the k-nearest neighbors (Euclidean uniform) model using PCA as a machine-learning model for demolition-waste-generation rate predictions.
更多查看译文
关键词
demolition-waste-generation rate,hybrid model,machine learning,principal component analysis,waste management
AI 理解论文
溯源树
样例
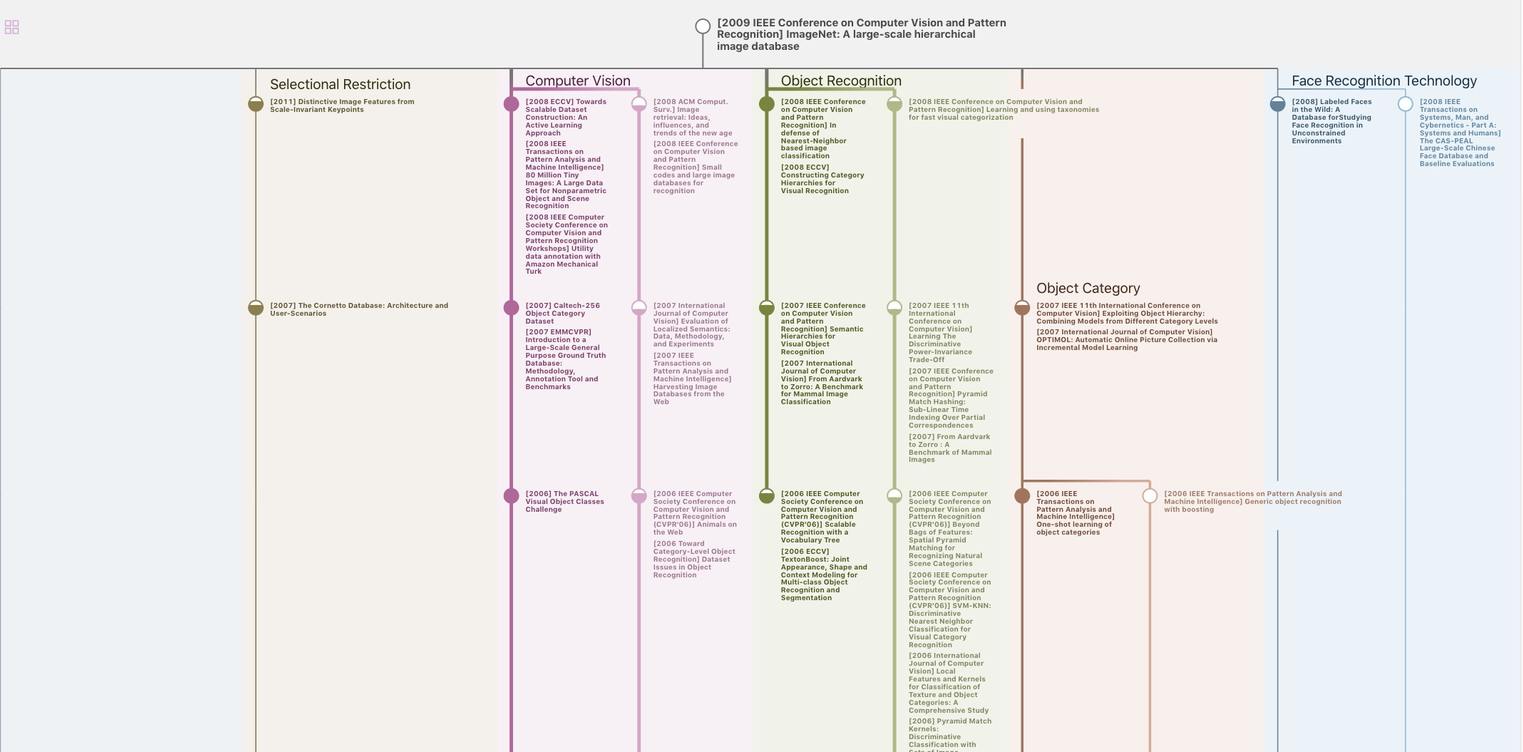
生成溯源树,研究论文发展脉络
Chat Paper
正在生成论文摘要