Machine Learning Models for Predicting Adverse Pregnancy Outcomes in Pregnant Women with Systemic Lupus Erythematosus.
Diagnostics (Basel, Switzerland)(2023)
摘要
Predicting adverse outcomes is essential for pregnant women with systemic lupus erythematosus (SLE) to minimize risks. Applying statistical analysis may be limited for the small sample size of childbearing patients, while the informative medical records could be provided. This study aimed to develop predictive models applying machine learning (ML) techniques to explore more information. We performed a retrospective analysis of 51 pregnant women exhibiting SLE, including 288 variables. After correlation analysis and feature selection, six ML models were applied to the filtered dataset. The efficiency of these overall models was evaluated by the Receiver Operating Characteristic Curve. Meanwhile, real-time models with different timespans based on gestation were also explored. Eighteen variables demonstrated statistical differences between the two groups; more than forty variables were screened out by ML variable selection strategies as contributing predictors, while the overlap of variables were the influential indicators testified by the two selection strategies. The Random Forest (RF) algorithm demonstrated the best discrimination ability under the current dataset for overall predictive models regardless of the data missing rate, while Multi-Layer Perceptron models ranked second. Meanwhile, RF achieved best performance when assessing the real-time predictive accuracy of models. ML models could compensate the limitation of statistical methods when the small sample size problem happens along with numerous variables acquired, while RF classifier performed relatively best when applied to such structured medical records.
更多查看译文
关键词
SLE,gestation,machine learning,prediction,pregnancy,random forest,systemic lupus erythematosus
AI 理解论文
溯源树
样例
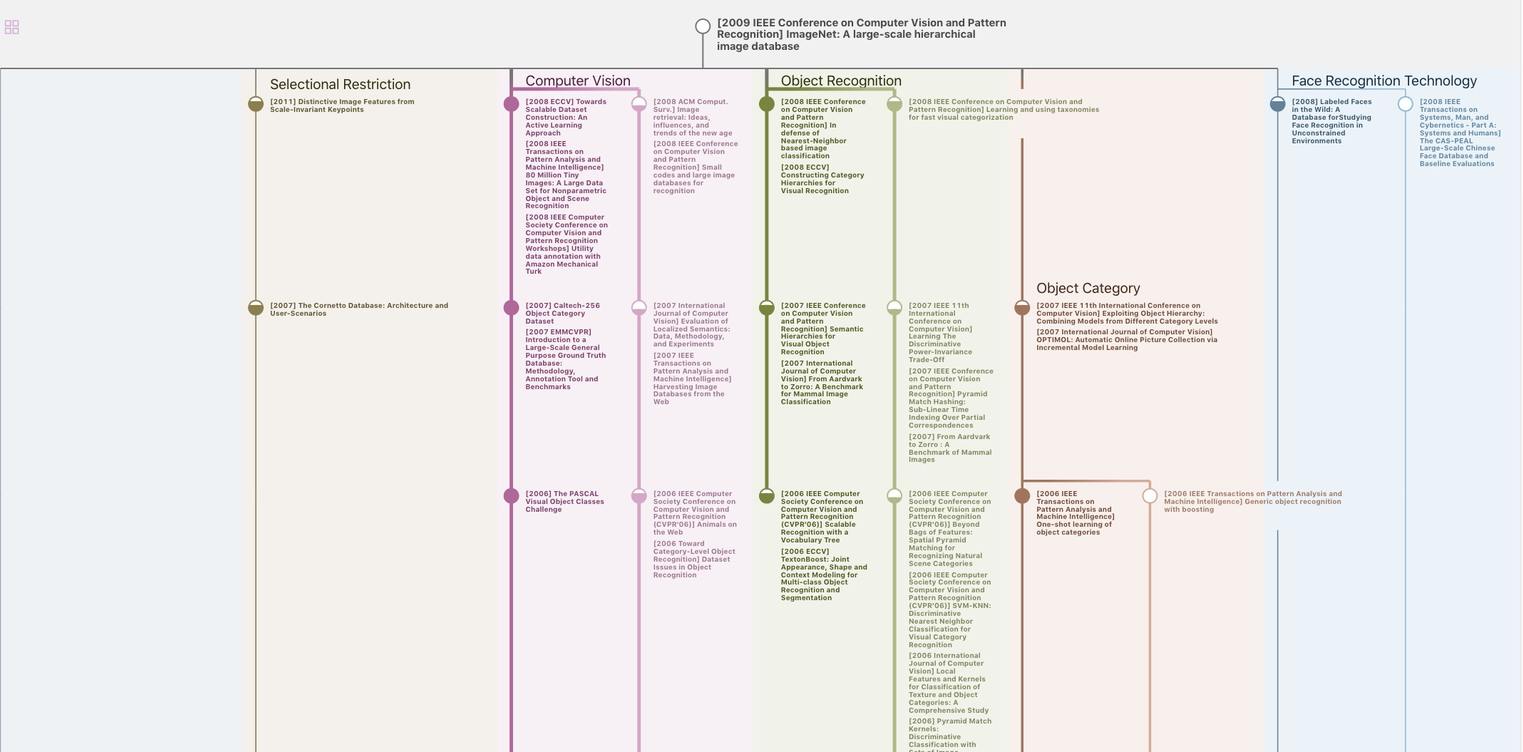
生成溯源树,研究论文发展脉络
Chat Paper
正在生成论文摘要