Revisiting Variable Ordering for Real Quantifier Elimination using Machine Learning
arxiv(2023)
摘要
Cylindrical Algebraic Decomposition (CAD) is a key proof technique for formal verification of cyber-physical systems. CAD is computationally expensive, with worst-case doubly-exponential complexity. Selecting an optimal variable ordering is paramount to efficient use of CAD. Prior work has demonstrated that machine learning can be useful in determining efficient variable orderings. Much of this work has been driven by CAD problems extracted from applications of the MetiTarski theorem prover. In this paper, we revisit this prior work and consider issues of bias in existing training and test data. We observe that the classical MetiTarski benchmarks are heavily biased towards particular variable orderings. To address this, we apply symmetries to create a new dataset containing more than 41K MetiTarski challenges designed to remove bias. Furthermore, we evaluate issues of information leakage, and test the generalizability of our models on the new dataset.
更多查看译文
关键词
real quantifier elimination,variable ordering,machine learning
AI 理解论文
溯源树
样例
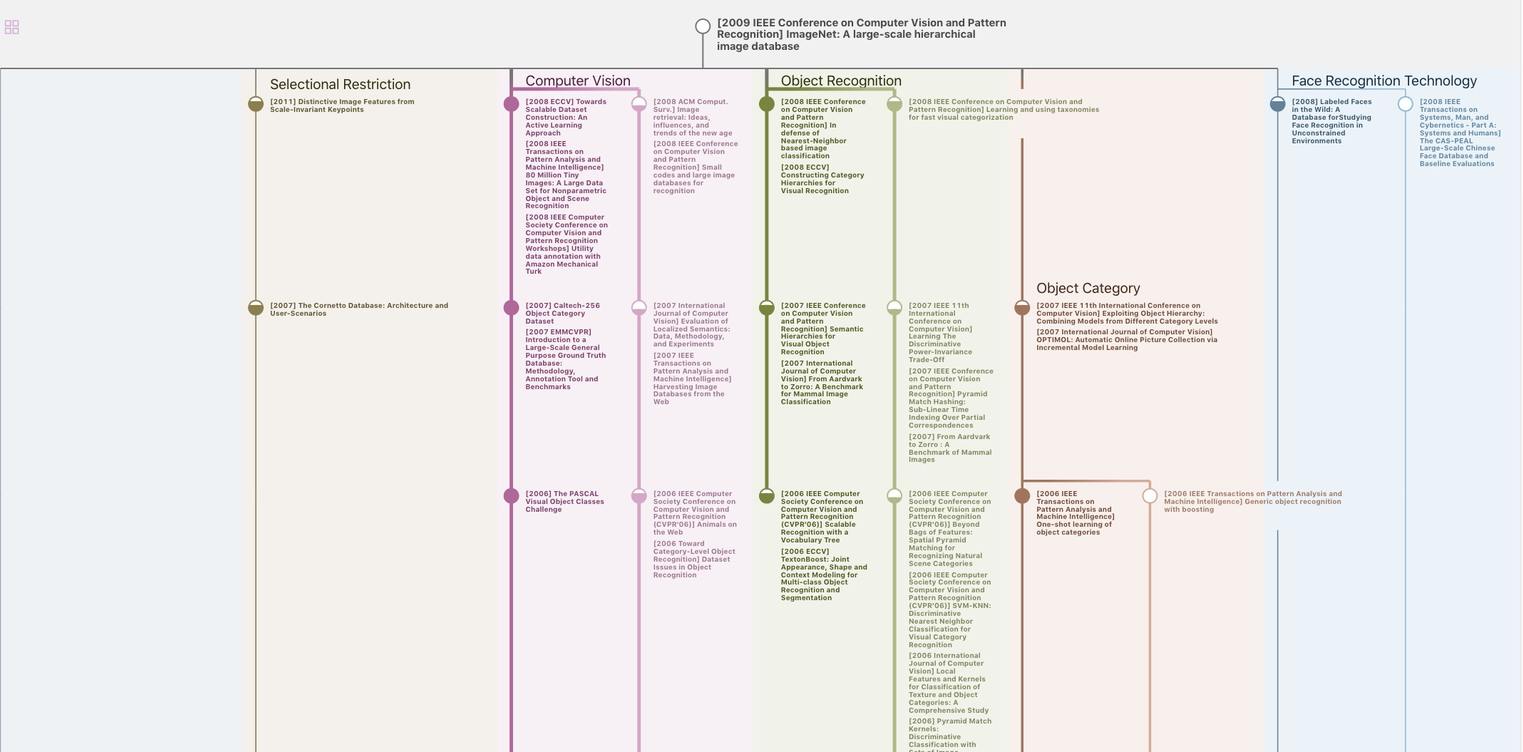
生成溯源树,研究论文发展脉络
Chat Paper
正在生成论文摘要