Causal isotonic calibration for heterogeneous treatment effects
arxiv(2023)
摘要
We propose causal isotonic calibration, a novel nonparametric method for calibrating predictors of heterogeneous treatment effects. In addition, we introduce a novel data-efficient variant of calibration that avoids the need for hold-out calibration sets, which we refer to as cross-calibration. Causal isotonic cross-calibration takes cross-fitted predictors and outputs a single calibrated predictor obtained using all available data. We establish under weak conditions that causal isotonic calibration and cross-calibration both achieve fast doubly-robust calibration rates so long as either the propensity score or outcome regression is estimated well in an appropriate sense. The proposed causal isotonic calibrator can be wrapped around any black-box learning algorithm to provide strong distribution-free calibration guarantees while preserving predictive performance.
更多查看译文
关键词
causal isotonic calibration,heterogeneous treatment effects
AI 理解论文
溯源树
样例
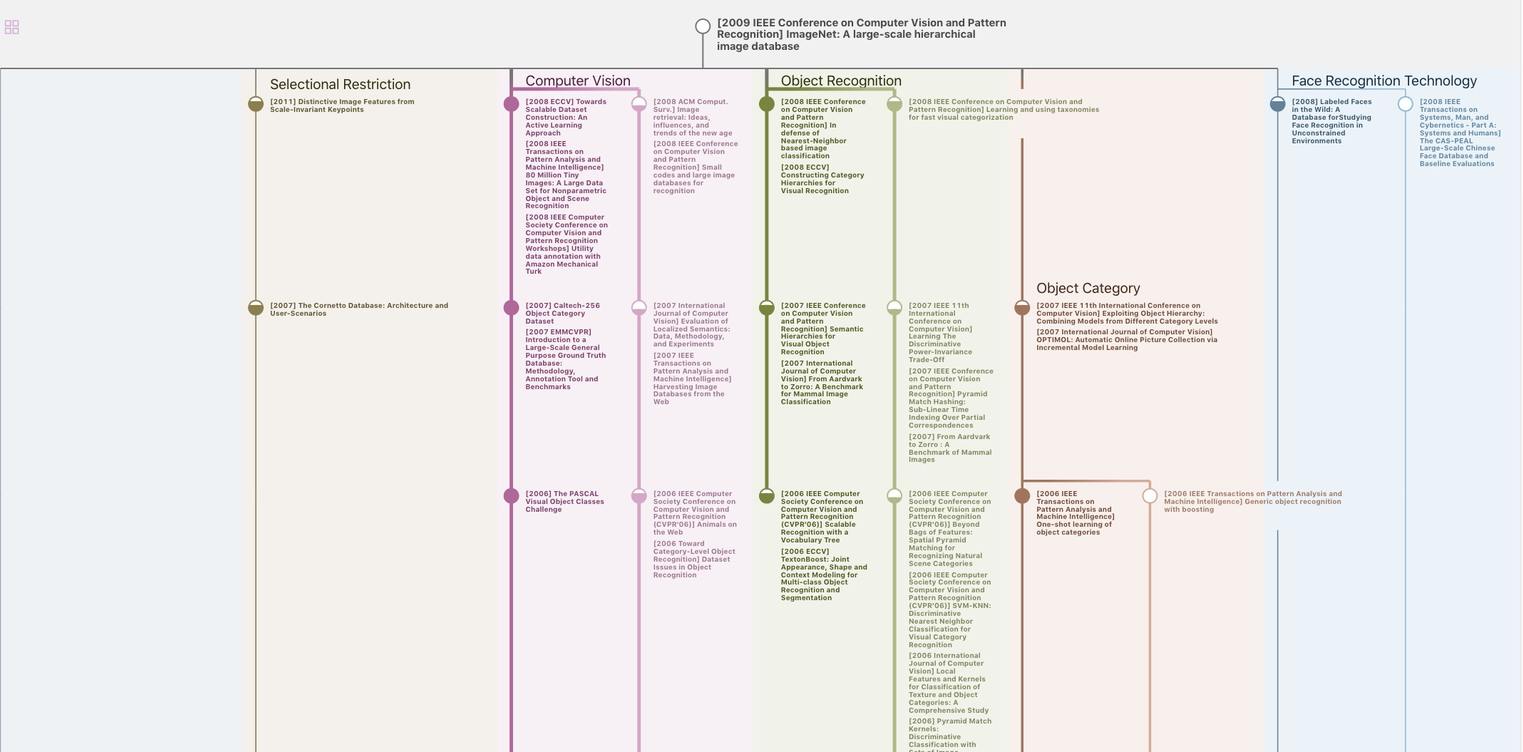
生成溯源树,研究论文发展脉络
Chat Paper
正在生成论文摘要