Implicit Poisoning Attacks in Two-Agent Reinforcement Learning: Adversarial Policies for Training-Time Attacks
arxiv(2023)
摘要
In targeted poisoning attacks, an attacker manipulates an agent-environment interaction to force the agent into adopting a policy of interest, called target policy. Prior work has primarily focused on attacks that modify standard MDP primitives, such as rewards or transitions. In this paper, we study targeted poisoning attacks in a two-agent setting where an attacker implicitly poisons the effective environment of one of the agents by modifying the policy of its peer. We develop an optimization framework for designing optimal attacks, where the cost of the attack measures how much the solution deviates from the assumed default policy of the peer agent. We further study the computational properties of this optimization framework. Focusing on a tabular setting, we show that in contrast to poisoning attacks based on MDP primitives (transitions and (unbounded) rewards), which are always feasible, it is NP-hard to determine the feasibility of implicit poisoning attacks. We provide characterization results that establish sufficient conditions for the feasibility of the attack problem, as well as an upper and a lower bound on the optimal cost of the attack. We propose two algorithmic approaches for finding an optimal adversarial policy: a model-based approach with tabular policies and a model-free approach with parametric/neural policies. We showcase the efficacy of the proposed algorithms through experiments.
更多查看译文
关键词
implicit poisoning attacks,adversarial policies,two-agent,training-time
AI 理解论文
溯源树
样例
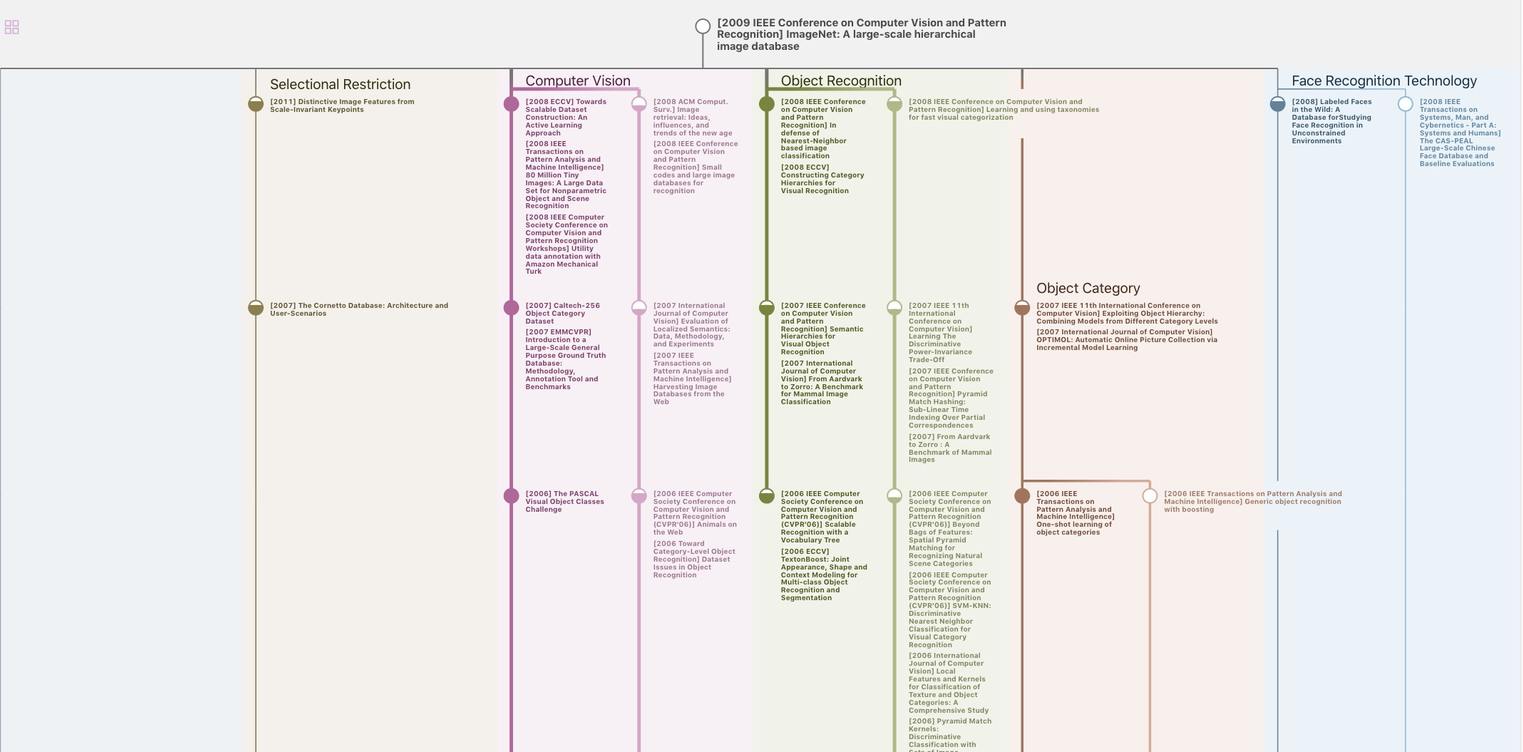
生成溯源树,研究论文发展脉络
Chat Paper
正在生成论文摘要