Self Correspondence Distillation for End-to-End Weakly-Supervised Semantic Segmentation
arxiv(2023)
摘要
Efficiently training accurate deep models for weakly supervised semantic segmentation (WSSS) with image-level labels is challenging and important. Recently, end-to-end WSSS methods have become the focus of research due to their high training efficiency. However, current methods suffer from insufficient extraction of comprehensive semantic information, resulting in low-quality pseudo-labels and sub-optimal solutions for end-to-end WSSS. To this end, we propose a simple and novel Self Correspondence Distillation (SCD) method to refine pseudo-labels without introducing external supervision. Our SCD enables the network to utilize feature correspondence derived from itself as a distillation target, which can enhance the network's feature learning process by complementing semantic information. In addition, to further improve the segmentation accuracy, we design a Variation-aware Refine Module to enhance the local consistency of pseudo-labels by computing pixel-level variation. Finally, we present an efficient end-to-end Transformer-based framework (TSCD) via SCD and Variation-aware Refine Module for the accurate WSSS task. Extensive experiments on the PASCAL VOC 2012 and MS COCO 2014 datasets demonstrate that our method significantly outperforms other state-of-the-art methods. Our code is available at {https://github.com/Rongtao-Xu/RepresentationLearning/tree/main/SCD-AAAI2023}.
更多查看译文
关键词
segmentation,correspondence,self,end-to-end,weakly-supervised
AI 理解论文
溯源树
样例
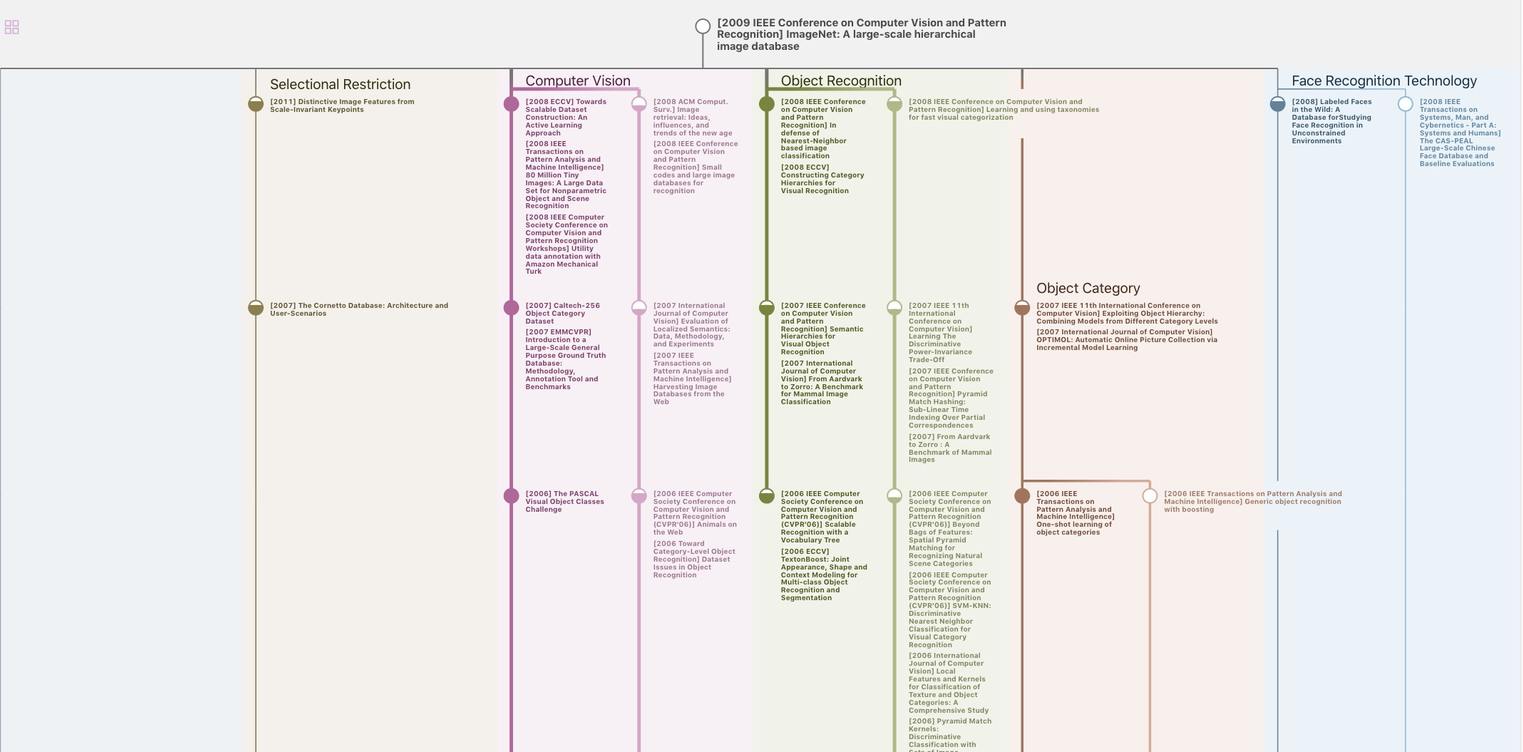
生成溯源树,研究论文发展脉络
Chat Paper
正在生成论文摘要