The Role of Pre-training Data in Transfer Learning
ICLR 2023(2023)
摘要
The transfer learning paradigm of model pre-training and subsequent fine-tuning produces high-accuracy models. While most studies recommend scaling the pre-training size to benefit most from transfer learning, a question remains: what data and method should be used for pre-training? We investigate the impact of pre-training data distribution on the few-shot and full fine-tuning performance using 3 pre-training methods (supervised, contrastive language-image and image-image), 7 pre-training datasets, and 9 downstream datasets. Through extensive controlled experiments, we find that the choice of the pre-training data source is essential for the few-shot transfer, but its role decreases as more data is made available for fine-tuning. Additionally, we explore the role of data curation and examine the trade-offs between label noise and the size of the pre-training dataset. We find that using 2000X more pre-training data from LAION can match the performance of supervised ImageNet pre-training. Furthermore, we investigate the effect of pre-training methods, comparing language-image contrastive vs. image-image contrastive, and find that the latter leads to better downstream accuracy
更多查看译文
关键词
pretraining,transfer learning,supervised training,constrastive learning,clip,simclr
AI 理解论文
溯源树
样例
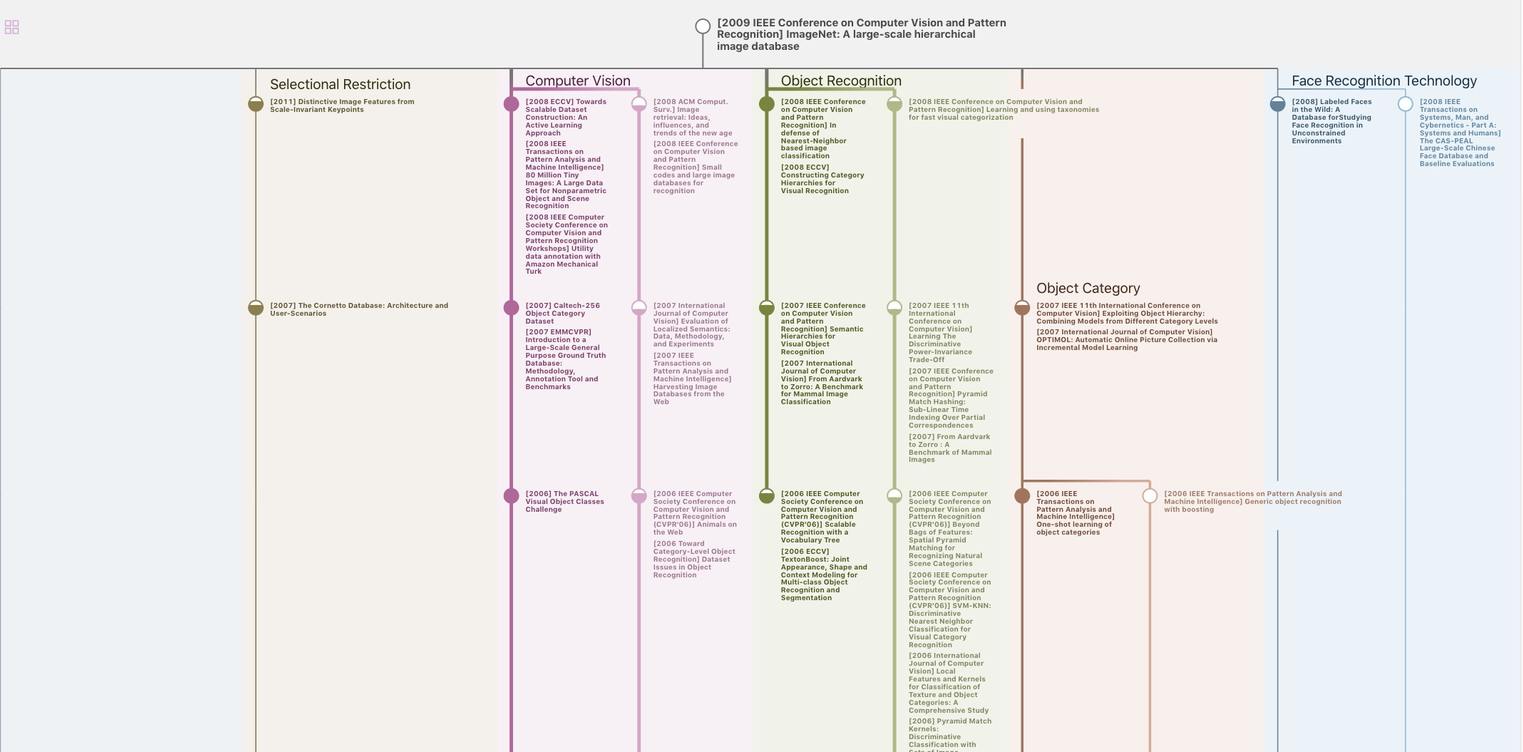
生成溯源树,研究论文发展脉络
Chat Paper
正在生成论文摘要