FLAG: Fast Label-Adaptive Aggregation for Multi-label Classification in Federated Learning
arxiv(2023)
摘要
Federated learning aims to share private data to maximize the data utility without privacy leakage. Previous federated learning research mainly focuses on multi-class classification problems. However, multi-label classification is a crucial research problem close to real-world data properties. Nevertheless, a limited number of federated learning studies explore this research problem. Existing studies of multi-label federated learning did not consider the characteristics of multi-label data, i.e., they used the concept of multi-class classification to verify their methods' performance, which means it will not be feasible to apply their methods to real-world applications. Therefore, this study proposed a new multi-label federated learning framework with a Clustering-based Multi-label Data Allocation (CMDA) and a novel aggregation method, Fast Label-Adaptive Aggregation (FLAG), for multi-label classification in the federated learning environment. The experimental results demonstrate that our methods only need less than 50\% of training epochs and communication rounds to surpass the performance of state-of-the-art federated learning methods.
更多查看译文
关键词
aggregation,classification,label-adaptive,multi-label
AI 理解论文
溯源树
样例
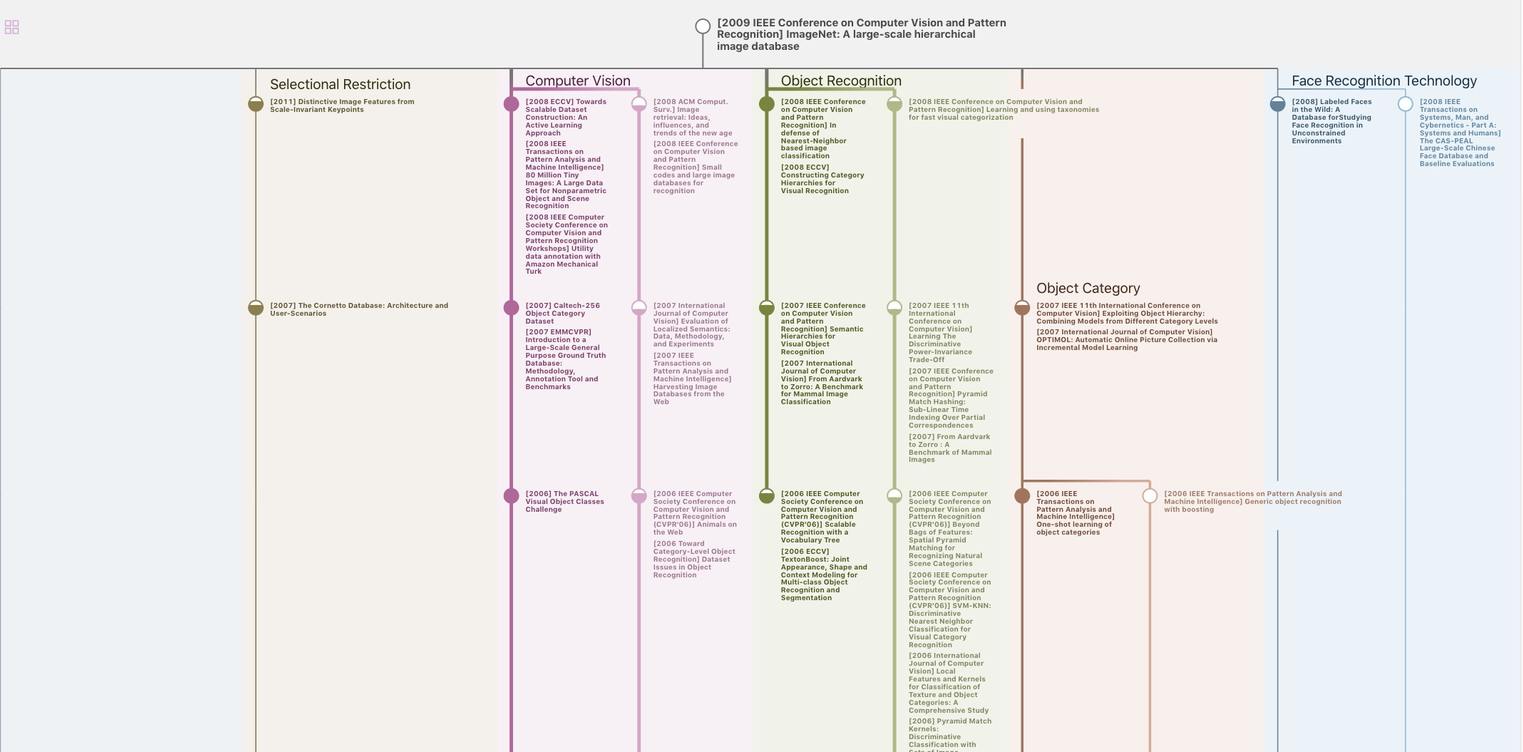
生成溯源树,研究论文发展脉络
Chat Paper
正在生成论文摘要