Finding Support Examples for In-Context Learning.
arxiv(2023)
摘要
In-context learning is a new learning paradigm where a language model observes a few examples and then straightly outputs the test input's prediction. Previous works have shown that in-context learning is sensitive to the provided examples and randomly sampled examples show significantly unstable performance. In this paper, we propose to find ``supporting examples'' for in-context learning: Given the training dataset, we need to select one permutation of a few examples, which are informative for the task's in-context learning and lead to superior performance. Although in traditional gradient-based learning, e.g., fine-tuning, there are numerous methods to find a ``coreset'' from the entire dataset, they are sub-optimal and not suitable for this problem since in-context learning occurs in the language model's inference without gradients or parameter updates. Additionally, the strong dependence among in-context examples makes this problem an NP-hard combinatorial optimization problem and enumerating all possible permutations is infeasible. Hence we propose a two-stage method to tackle this challenge. First we propose a novel metric to select informative examples based on the language model's feedback, with a progressive filtering strategy. And then we propose a diversity-guided beam search method to refine and evaluate the selected examples, iteratively. The experimental results show our method significantly outperforms a wide range of baselines, and further analyses show the effectiveness of our method and shed light on the properties of supporting examples and in-context learning.
更多查看译文
关键词
examples,learning,in-context
AI 理解论文
溯源树
样例
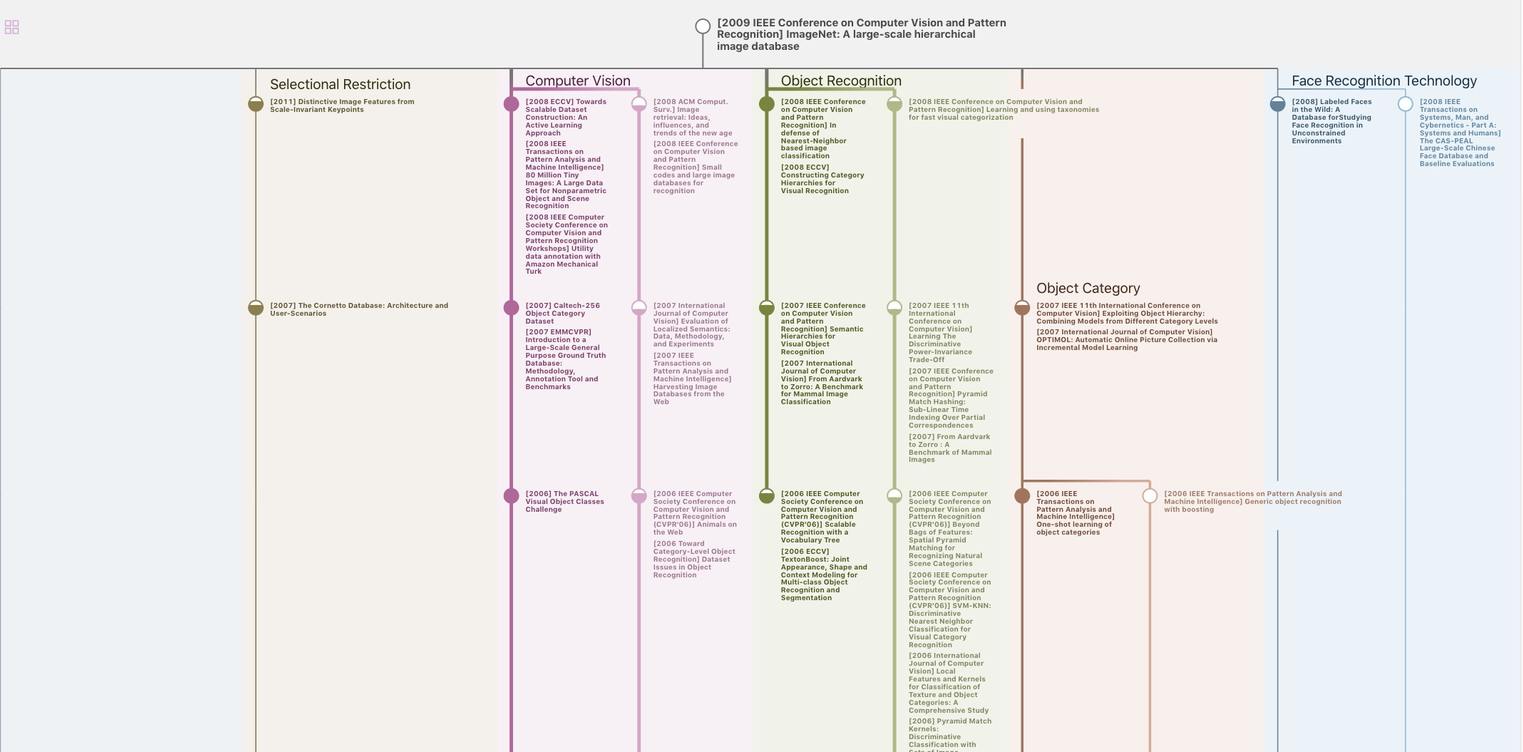
生成溯源树,研究论文发展脉络
Chat Paper
正在生成论文摘要