Euclidean Contractivity of Neural Networks With Symmetric Weights
arxiv(2023)
摘要
This letter investigates stability conditions of continuous-time Hopfield and firing-rate neural networks by leveraging contraction theory. First, we present a number of useful general algebraic results on matrix polytopes and products of symmetric matrices. Then, we give sufficient conditions for strong and weak Euclidean contractivity, i.e., contractivity with respect to the $\ell _{2}$ norm, of both models with symmetric weights and (possibly) non-smooth activation functions. Our contraction analysis leads to contraction rates which are log-optimal in almost all symmetric synaptic matrices. Finally, we use our results to propose a firing-rate neural network model to solve a quadratic optimization problem with box constraints.
更多查看译文
关键词
Symmetric matrices,Fuzzy control,Asymptotic stability,Stability criteria,Recurrent neural networks,Numerical stability,Optimization,Neural networks,contraction theory,optimization,stability of nonlinear systems
AI 理解论文
溯源树
样例
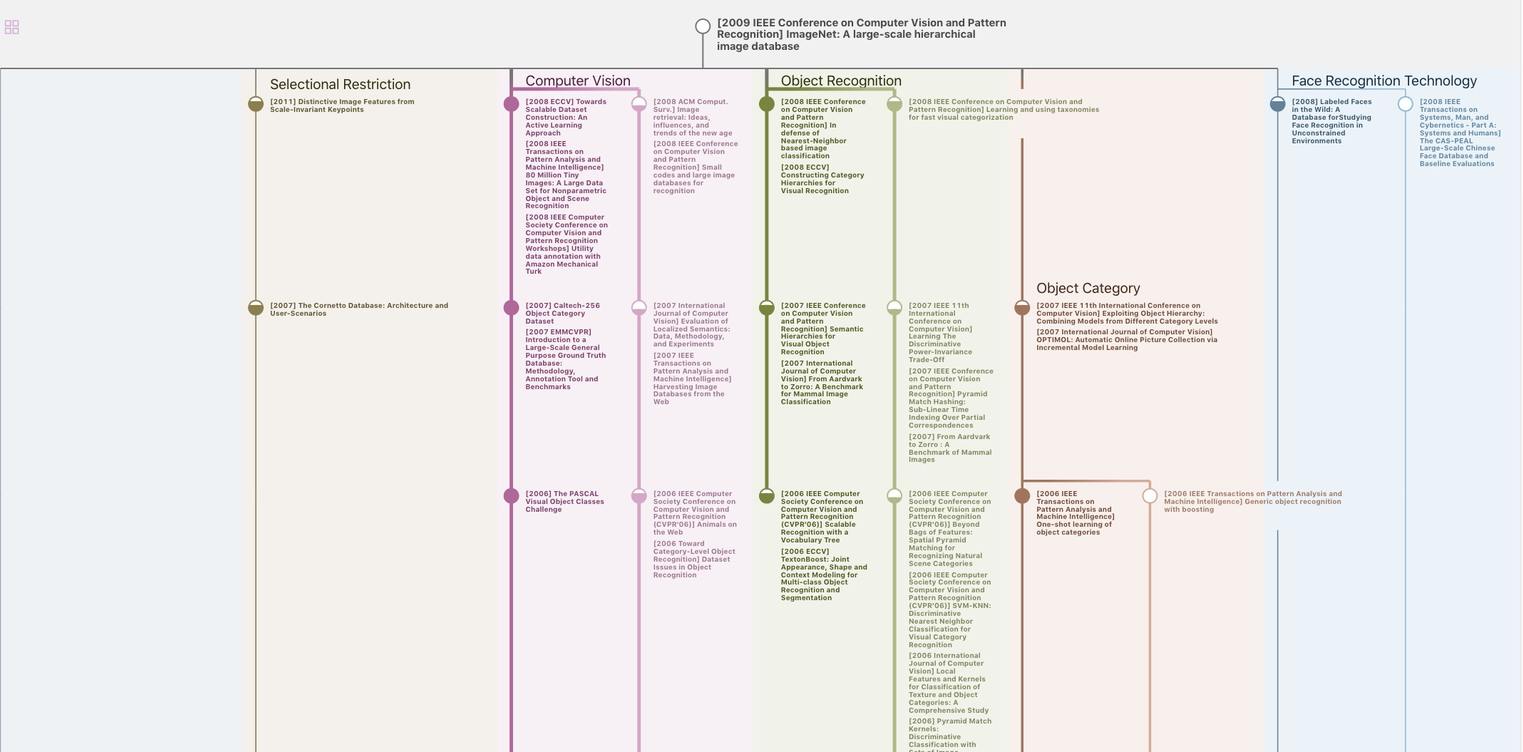
生成溯源树,研究论文发展脉络
Chat Paper
正在生成论文摘要