P4L: Privacy Preserving Peer-to-Peer Learning for Infrastructureless Setups
arxiv(2023)
摘要
Distributed (or Federated) learning enables users to train machine learning models on their very own devices, while they share only the gradients of their models usually in a differentially private way (utility loss). Although such a strategy provides better privacy guarantees than the traditional centralized approach, it requires users to blindly trust a centralized infrastructure that may also become a bottleneck with the increasing number of users. In this paper, we design and implement P4L: a privacy preserving peer-to-peer learning system for users to participate in an asynchronous, collaborative learning scheme without requiring any sort of infrastructure or relying on differential privacy. Our design uses strong cryptographic primitives to preserve both the confidentiality and utility of the shared gradients, a set of peer-to-peer mechanisms for fault tolerance and user churn, proximity and cross device communications. Extensive simulations under different network settings and ML scenarios for three real-life datasets show that P4L provides competitive performance to baselines, while it is resilient to different poisoning attacks. We implement P4L and experimental results show that the performance overhead and power consumption is minimal (less than 3mAh of discharge).
更多查看译文
关键词
privacy,infrastructureless
AI 理解论文
溯源树
样例
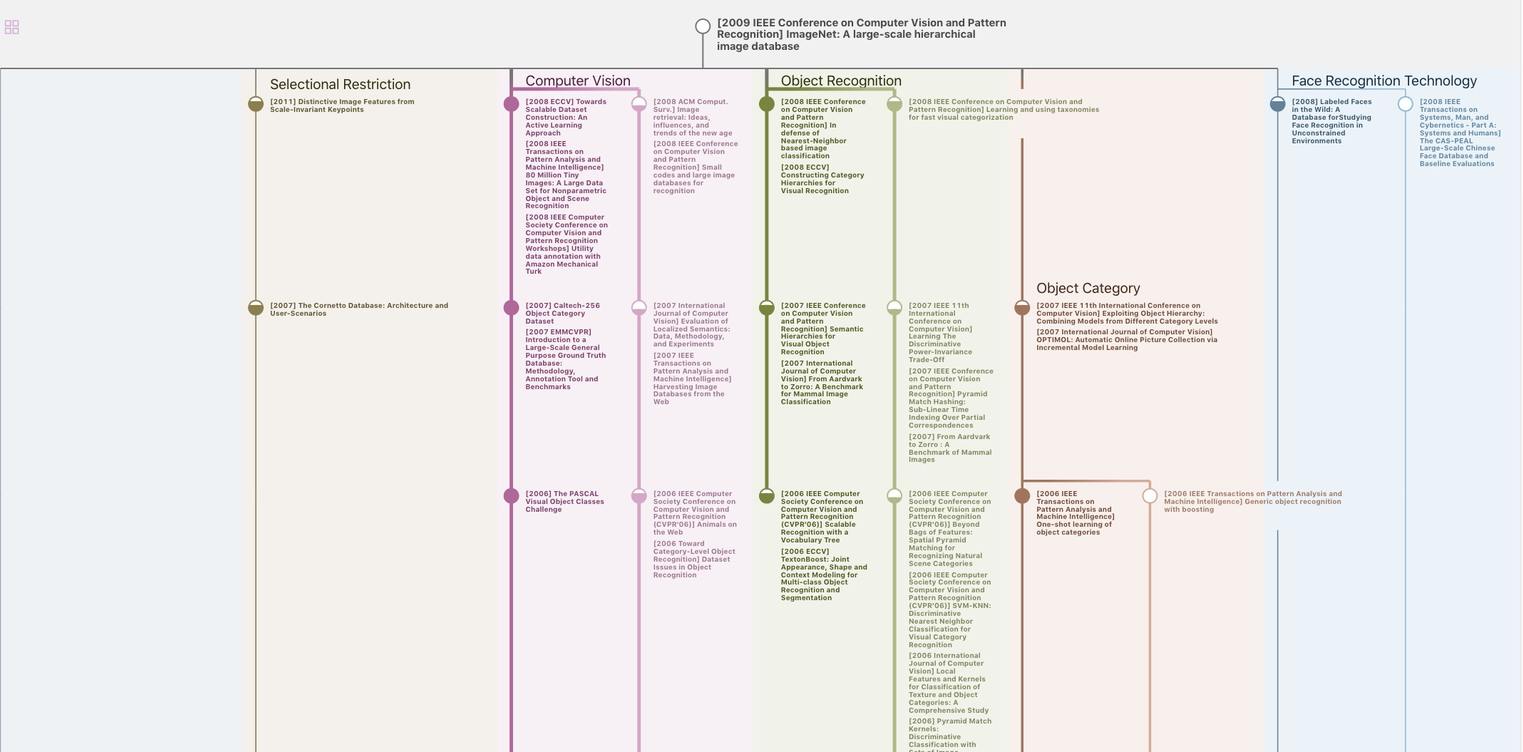
生成溯源树,研究论文发展脉络
Chat Paper
正在生成论文摘要