TransferD2: Automated Defect Detection Approach in Smart Manufacturing using Transfer Learning Techniques
arxiv(2023)
摘要
Quality assurance is crucial in the smart manufacturing industry as it identifies the presence of defects in finished products before they are shipped out. Modern machine learning techniques can be leveraged to provide rapid and accurate detection of these imperfections. We, therefore, propose a transfer learning approach, namely TransferD2, to correctly identify defects on a dataset of source objects and extend its application to new unseen target objects. We present a data enhancement technique to generate a large dataset from the small source dataset for building a classifier. We then integrate three different pre-trained models (Xception, ResNet101V2, and InceptionResNetV2) into the classifier network and compare their performance on source and target data. We use the classifier to detect the presence of imperfections on the unseen target data using pseudo-bounding boxes. Our results show that ResNet101V2 performs best on the source data with an accuracy of 95.72%. Xception performs best on the target data with an accuracy of 91.00% and also provides a more accurate prediction of the defects on the target images. Throughout the experiment, the results also indicate that the choice of a pre-trained model is not dependent on the depth of the network. Our proposed approach can be applied in defect detection applications where insufficient data is available for training a model and can be extended to identify imperfections in new unseen data.
更多查看译文
关键词
automated defect detection approach,transferd2 learning techniques,transferd2 learning,smart manufacturing
AI 理解论文
溯源树
样例
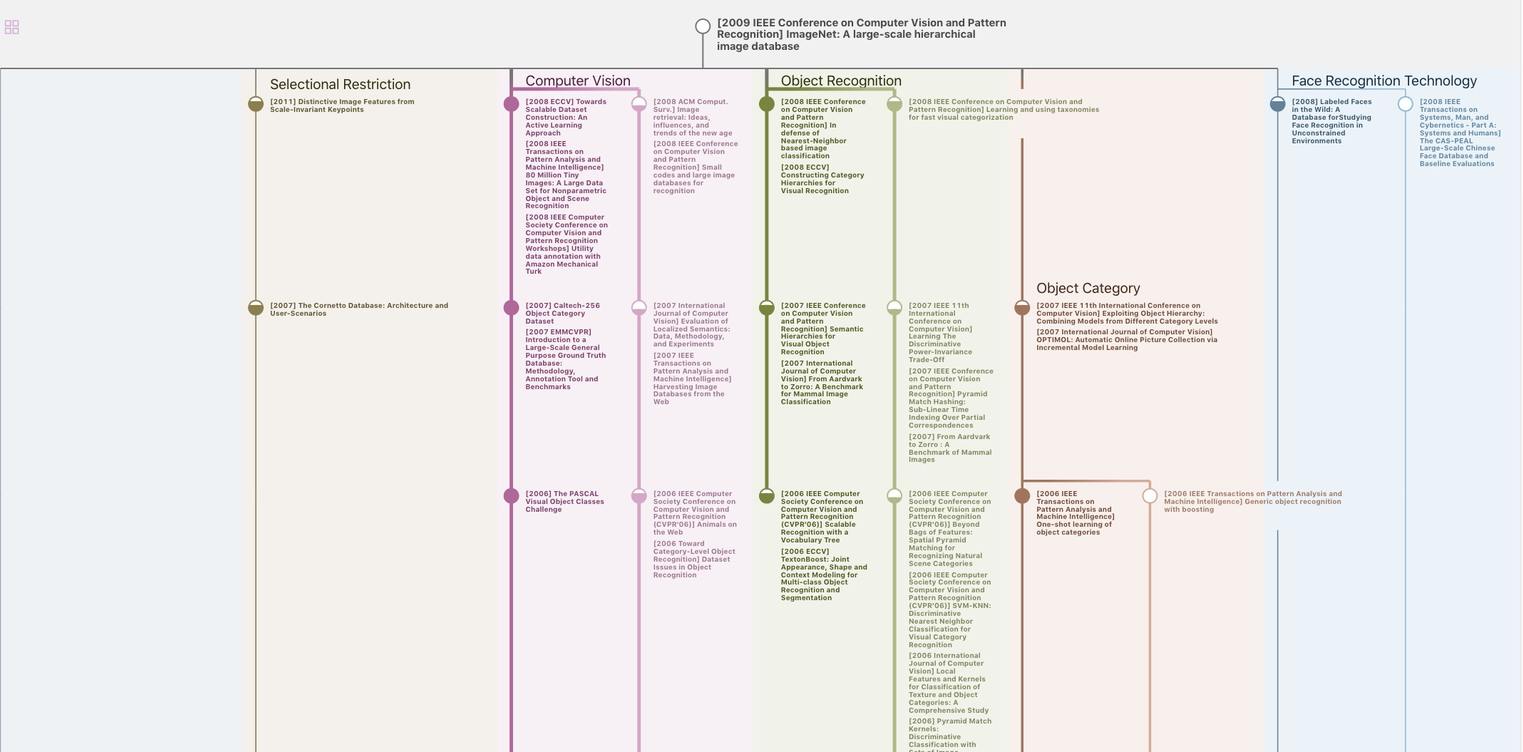
生成溯源树,研究论文发展脉络
Chat Paper
正在生成论文摘要