Combining Graphical and Algebraic Approaches for Parameter Identification in Latent Variable Structural Equation Models
arxiv(2023)
摘要
Measurement error is ubiquitous in many variables - from blood pressure recordings in physiology to intelligence measures in psychology. Structural equation models (SEMs) account for the process of measurement by explicitly distinguishing between latent variables and their measurement indicators. Users often fit entire SEMs to data, but this can fail if some model parameters are not identified. The model-implied instrumental variables (MIIVs) approach is a more flexible alternative that can estimate subsets of model parameters in identified equations. Numerous methods to identify individual parameters also exist in the field of graphical models (such as DAGs), but many of these do not account for measurement effects. Here, we take the concept of "latent-to-observed" (L2O) transformation from the MIIV approach and develop an equivalent graphical L2O transformation that allows applying existing graphical criteria to latent parameters in SEMs. We combine L2O transformation with graphical instrumental variable criteria to obtain an efficient algorithm for non-iterative parameter identification in SEMs with latent variables. We prove that this graphical L2O transformation with the instrumental set criterion is equivalent to the state-of-the-art MIIV approach for SEMs, and show that it can lead to novel identification strategies when combined with other graphical criteria.
更多查看译文
关键词
structural equation,models,parameter
AI 理解论文
溯源树
样例
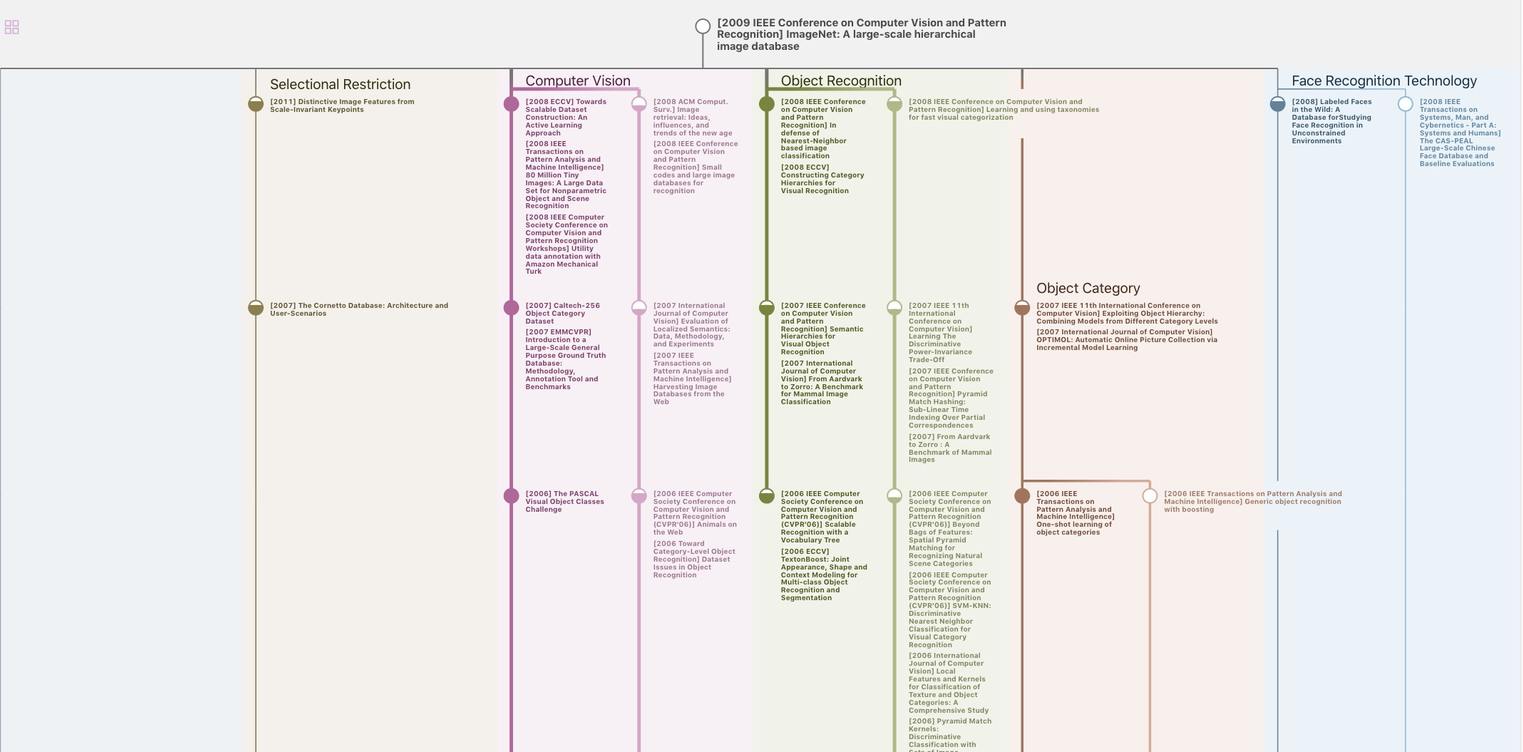
生成溯源树,研究论文发展脉络
Chat Paper
正在生成论文摘要