Temporal Segment Transformer for Action Segmentation
arxiv(2023)
摘要
Recognizing human actions from untrimmed videos is an important task in activity understanding, and poses unique challenges in modeling long-range temporal relations. Recent works adopt a predict-and-refine strategy which converts an initial prediction to action segments for global context modeling. However, the generated segment representations are often noisy and exhibit inaccurate segment boundaries, over-segmentation and other problems. To deal with these issues, we propose an attention based approach which we call \textit{temporal segment transformer}, for joint segment relation modeling and denoising. The main idea is to denoise segment representations using attention between segment and frame representations, and also use inter-segment attention to capture temporal correlations between segments. The refined segment representations are used to predict action labels and adjust segment boundaries, and a final action segmentation is produced based on voting from segment masks. We show that this novel architecture achieves state-of-the-art accuracy on the popular 50Salads, GTEA and Breakfast benchmarks. We also conduct extensive ablations to demonstrate the effectiveness of different components of our design.
更多查看译文
关键词
action segmentation,temporal segment transformer
AI 理解论文
溯源树
样例
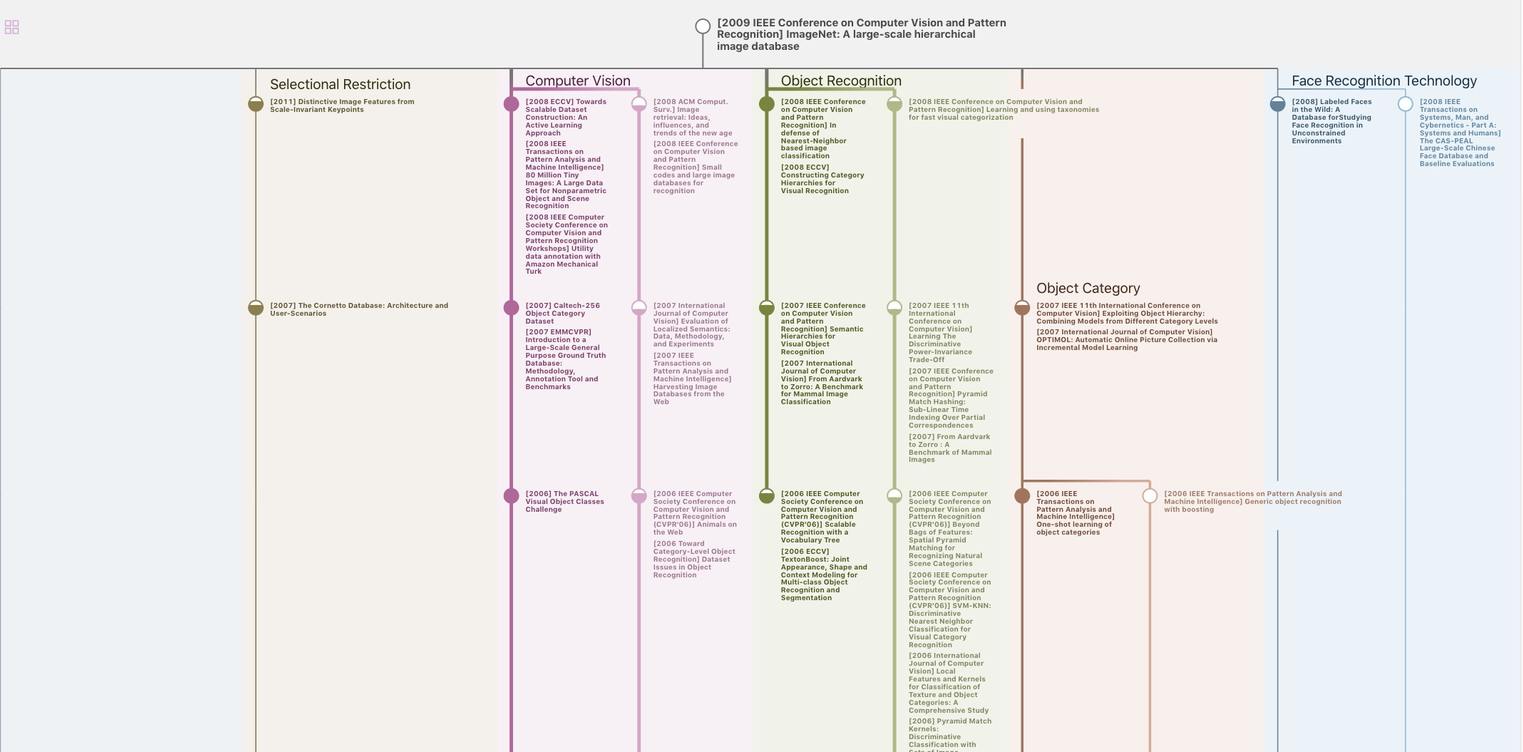
生成溯源树,研究论文发展脉络
Chat Paper
正在生成论文摘要