Failure-aware Policy Learning for Self-assessable Robotics Tasks
arxiv(2023)
摘要
Self-assessment rules play an essential role in safe and effective real-world robotic applications, which verify the feasibility of the selected action before actual execution. But how to utilize the self-assessment results to re-choose actions remains a challenge. Previous methods eliminate the selected action evaluated as failed by the self-assessment rules, and re-choose one with the next-highest affordance (i.e. process-of-elimination strategy [1]), which ignores the dependency between the self-assessment results and the remaining untried actions. However, this dependency is important since the previous failures might help trim the remaining over-estimated actions. In this paper, we set to investigate this dependency by learning a failure-aware policy. We propose two architectures for the failure-aware policy by representing the self-assessment results of previous failures as the variable state, and leveraging recurrent neural networks to implicitly memorize the previous failures. Experiments conducted on three tasks demonstrate that our method can achieve better performances with higher task success rates by less trials. Moreover, when the actions are correlated, learning a failure-aware policy can achieve better performance than the process-of-elimination strategy.
更多查看译文
关键词
failure-aware policy,previous failures,process-of-elimination strategy,real-world robotic applications,remaining over-estimated actions,remaining untried actions,selected action,self-assessable robotics tasks,self-assessment results,self-assessment rules
AI 理解论文
溯源树
样例
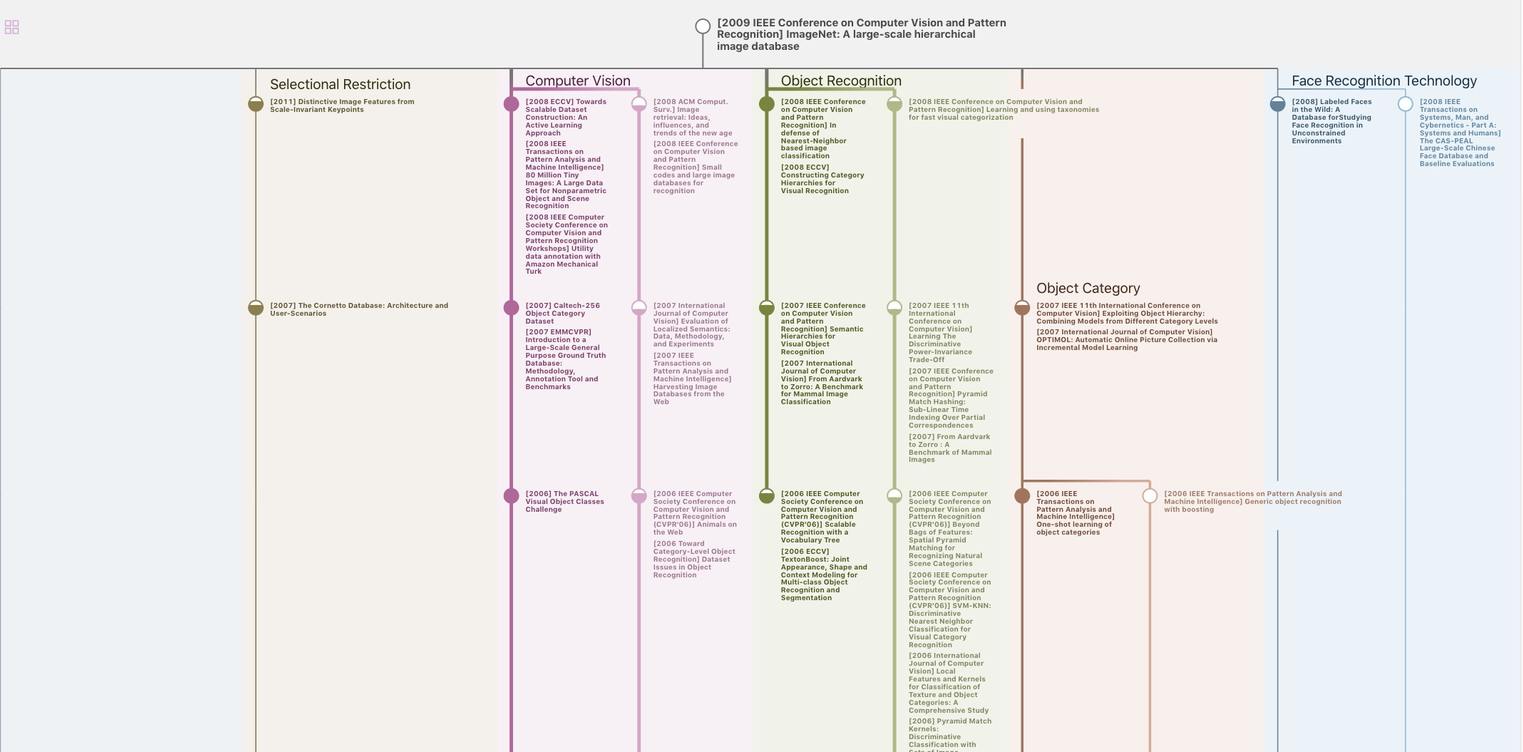
生成溯源树,研究论文发展脉络
Chat Paper
正在生成论文摘要