Elliptic PDE learning is provably data-efficient
PROCEEDINGS OF THE NATIONAL ACADEMY OF SCIENCES OF THE UNITED STATES OF AMERICA(2023)
摘要
Partial differential equations (PDE) learning is an emerging field that combines physics and machine learning to recover unknown physical systems from experimental data. While deep learning models traditionally require copious amounts of training data, recent PDE learning techniques achieve spectacular results with limited data availability. Still, these results are empirical. Our work provides theoretical guarantees on the number of input-output training pairs required in PDE learning. Specifically, we exploit randomized numerical linear algebra and PDE theory to derive a provably data efficient algorithm that recovers solution operators of three-dimensional uniformly elliptic PDEs from input-output data and achieves an exponential convergence rate of the error with respect to the size of the training dataset with an exceptionally high probability of success.
更多查看译文
关键词
deep learning,inverse problems,sample complexity,neural operators
AI 理解论文
溯源树
样例
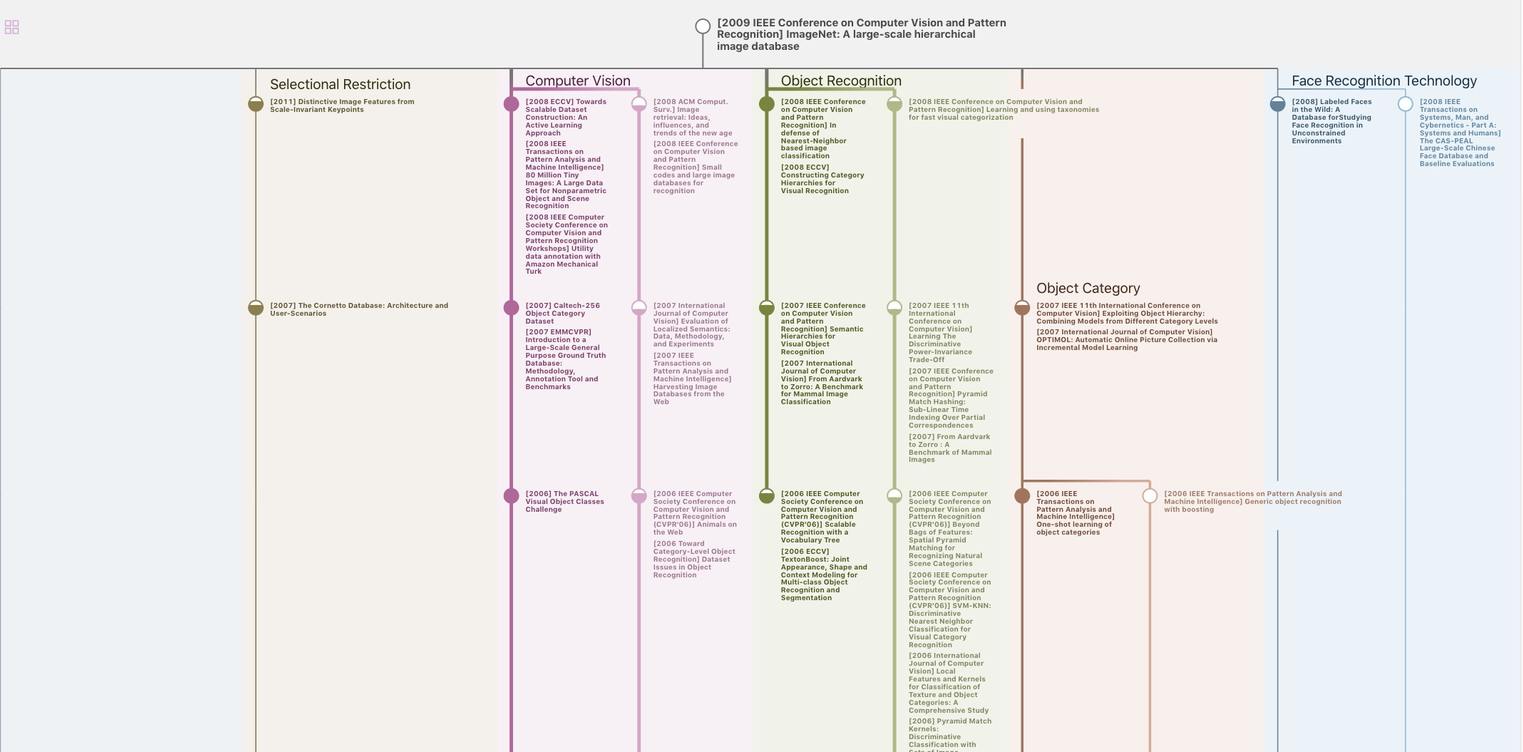
生成溯源树,研究论文发展脉络
Chat Paper
正在生成论文摘要