Ensemble knowledge distillation of self-supervised speech models
arxiv(2023)
摘要
Distilled self-supervised models have shown competitive performance and efficiency in recent years. However, there is a lack of experience in jointly distilling multiple self-supervised speech models. In our work, we performed Ensemble Knowledge Distillation (EKD) on various self-supervised speech models such as HuBERT, RobustHuBERT, and WavLM. We tried two different aggregation techniques, layerwise-average and layerwise-concatenation, to the representations of different teacher models and found that the former was more effective. On top of that, we proposed a multiple prediction head method for student models to predict different layer outputs of multiple teacher models simultaneously. The experimental results show that our method improves the performance of the distilled models on four downstream speech processing tasks, Phoneme Recognition, Speaker Identification, Emotion Recognition, and Automatic Speech Recognition in the hidden-set track of the SUPERB benchmark.
更多查看译文
关键词
ensemble knowledge distillation,models,speech,self-supervised
AI 理解论文
溯源树
样例
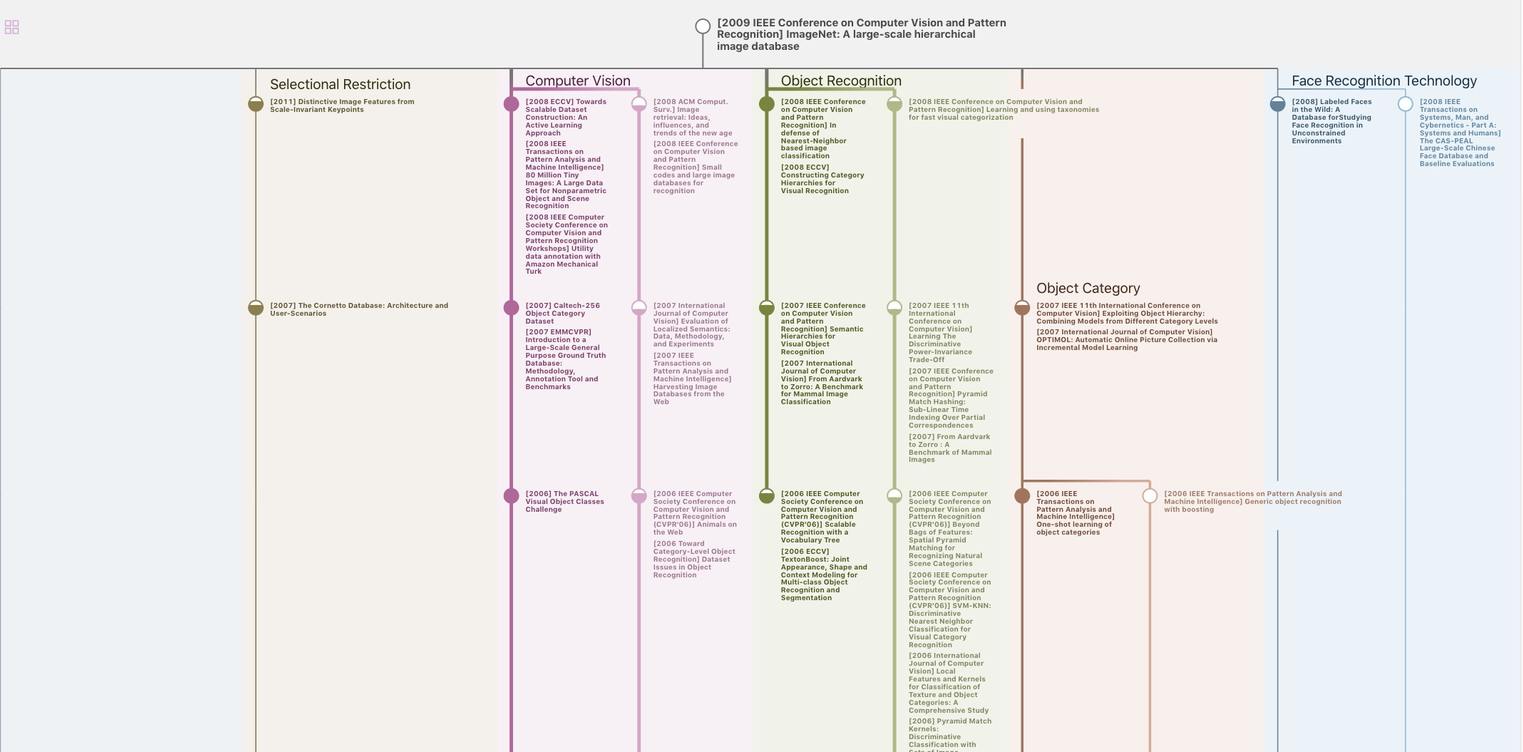
生成溯源树,研究论文发展脉络
Chat Paper
正在生成论文摘要