Emulation methods and adaptive sampling increase the efficiency of sensitivity analysis for computationally expensive models
arxiv(2023)
摘要
Models with high-dimensional parameter spaces are common in many applications. Global sensitivity analyses can provide insights on how uncertain inputs and interactions influence the outputs. Many sensitivity analysis methods face nontrivial challenges for computationally demanding models. Common approaches to tackle these challenges are to (i) use a computationally efficient emulator and (ii) sample adaptively. However, these approaches still involve potentially large computational costs and approximation errors. Here we compare the results and computational costs of four existing global sensitivity analysis methods applied to a test problem. We sample different model evaluation time and numbers of model parameters. We find that the emulation and adaptive sampling approaches are faster than Sobol' method for slow models. The Bayesian adaptive spline surface method is the fastest for most slow and high-dimensional models. Our results can guide the choice of a sensitivity analysis method under computational resources constraints.
更多查看译文
关键词
emulation methods,sensitivity analysis,adaptive sampling increase,expensive models
AI 理解论文
溯源树
样例
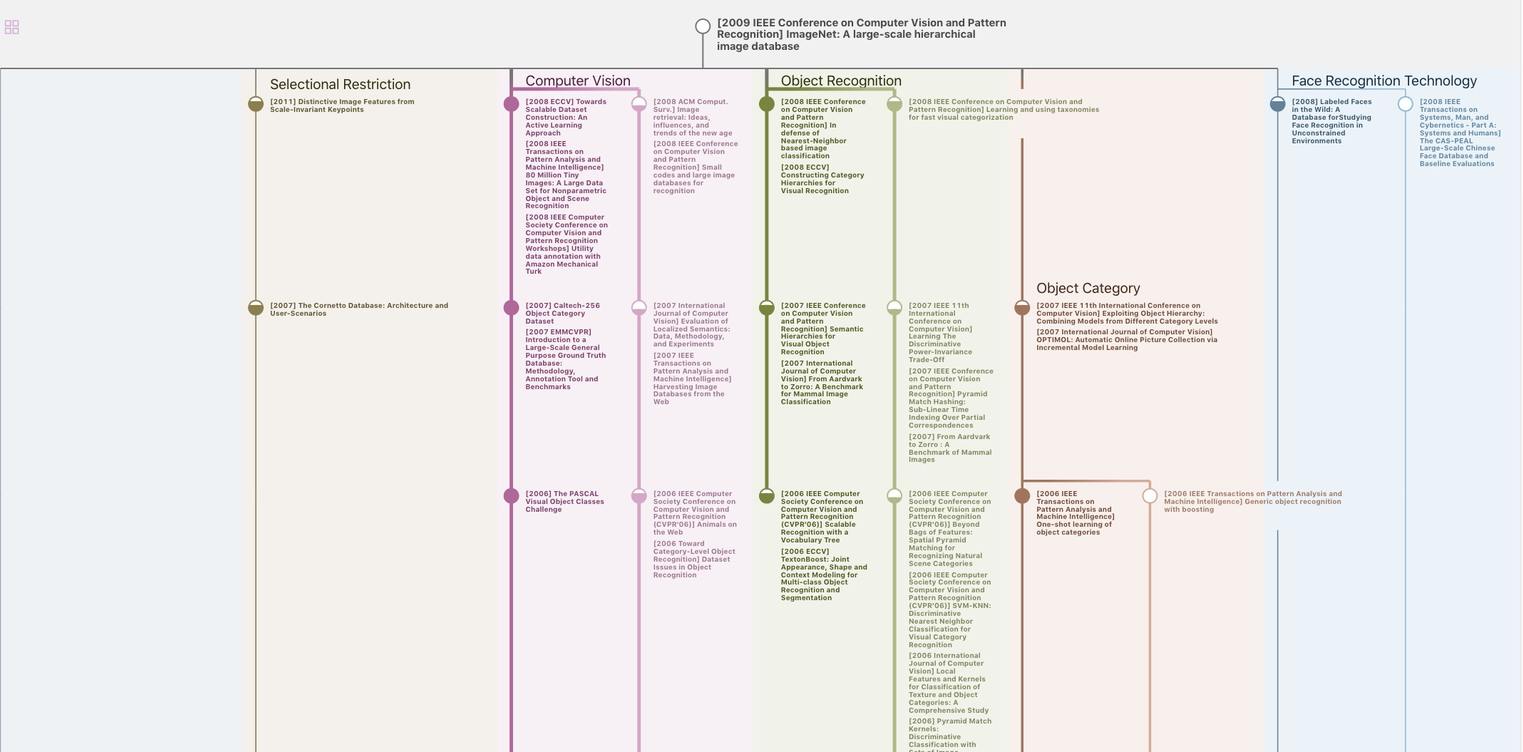
生成溯源树,研究论文发展脉络
Chat Paper
正在生成论文摘要